Leveraging Human Feedback to Evolve and Discover Novel Emergent Behaviors in Robot Swarms
CoRR(2023)
摘要
Robot swarms often exhibit emergent behaviors that are fascinating to observe; however, it is often difficult to predict what swarm behaviors can emerge under a given set of agent capabilities. We seek to efficiently leverage human input to automatically discover a taxonomy of collective behaviors that can emerge from a particular multi-agent system, without requiring the human to know beforehand what behaviors are interesting or even possible. Our proposed approach adapts to user preferences by learning a similarity space over swarm collective behaviors using self-supervised learning and human-in-the-loop queries. We combine our learned similarity metric with novelty search and clustering to explore and categorize the space of possible swarm behaviors. We also propose several general-purpose heuristics that improve the efficiency of our novelty search by prioritizing robot controllers that are likely to lead to interesting emergent behaviors. We test our approach in simulation on two robot capability models and show that our methods consistently discover a richer set of emergent behaviors than prior work. Code, videos, and datasets are available at https://sites.google.com/view/evolving- novel-swarms.
更多查看译文
关键词
Swarms,Novelty search,Emergent behaviors,Similarity learning
AI 理解论文
溯源树
样例
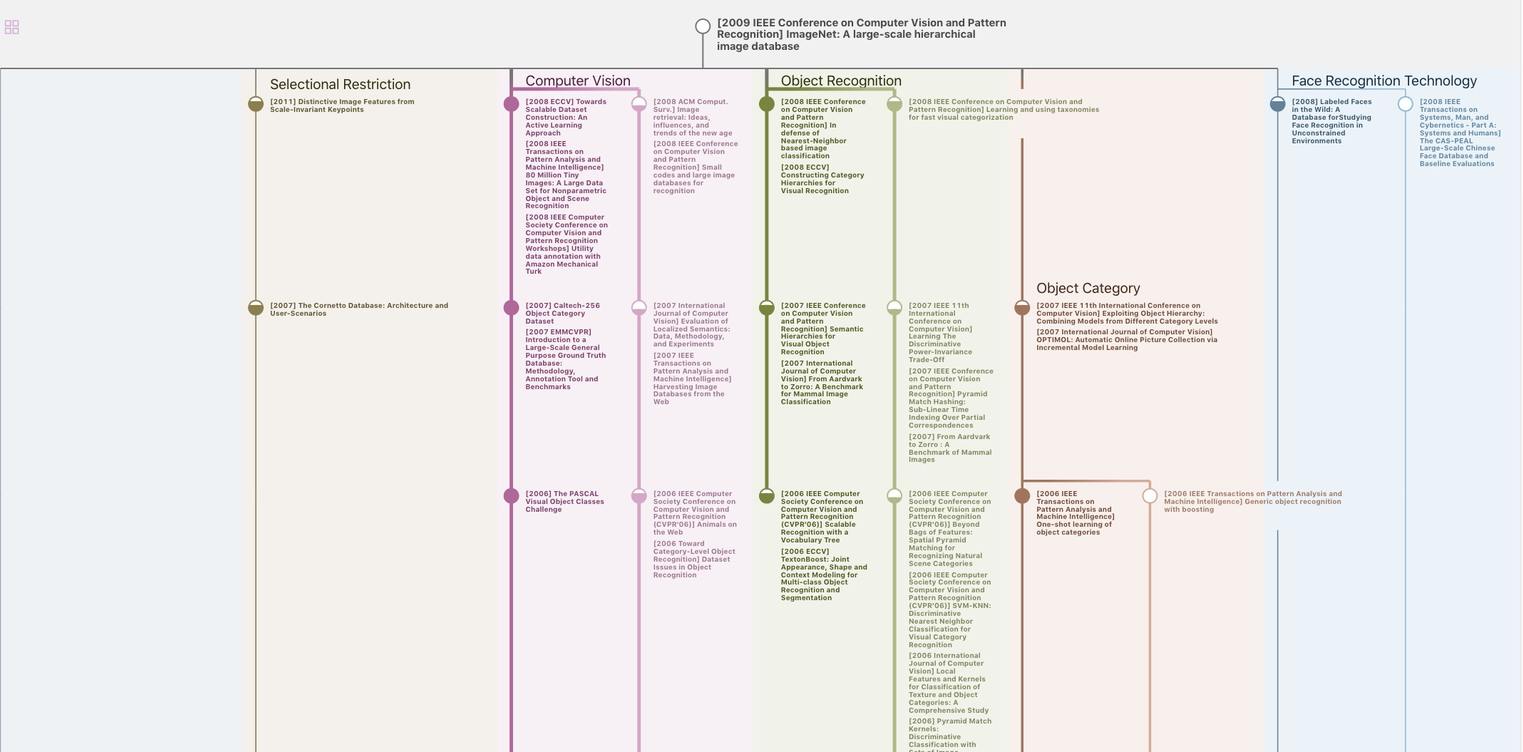
生成溯源树,研究论文发展脉络
Chat Paper
正在生成论文摘要