VioLA: Unified Codec Language Models for Speech Recognition, Synthesis, and Translation
CoRR(2023)
摘要
Recent research shows a big convergence in model architecture, training objectives, and inference methods across various tasks for different modalities. In this paper, we propose VioLA, a single auto-regressive Transformer decoder-only network that unifies various cross-modal tasks involving speech and text, such as speech-to-text, text-to-text, text-to-speech, and speech-to-speech tasks, as a conditional codec language model task via multi-task learning framework. To accomplish this, we first convert all the speech utterances to discrete tokens (similar to the textual data) using an offline neural codec encoder. In such a way, all these tasks are converted to token-based sequence conversion problems, which can be naturally handled with one conditional language model. We further integrate task IDs (TID) and language IDs (LID) into the proposed model to enhance the modeling capability of handling different languages and tasks. Experimental results demonstrate that the proposed VioLA model can support both single-modal and cross-modal tasks well, and the decoder-only model achieves a comparable and even better performance than the strong baselines.
更多查看译文
关键词
unified codec language models,speech recognition,viola
AI 理解论文
溯源树
样例
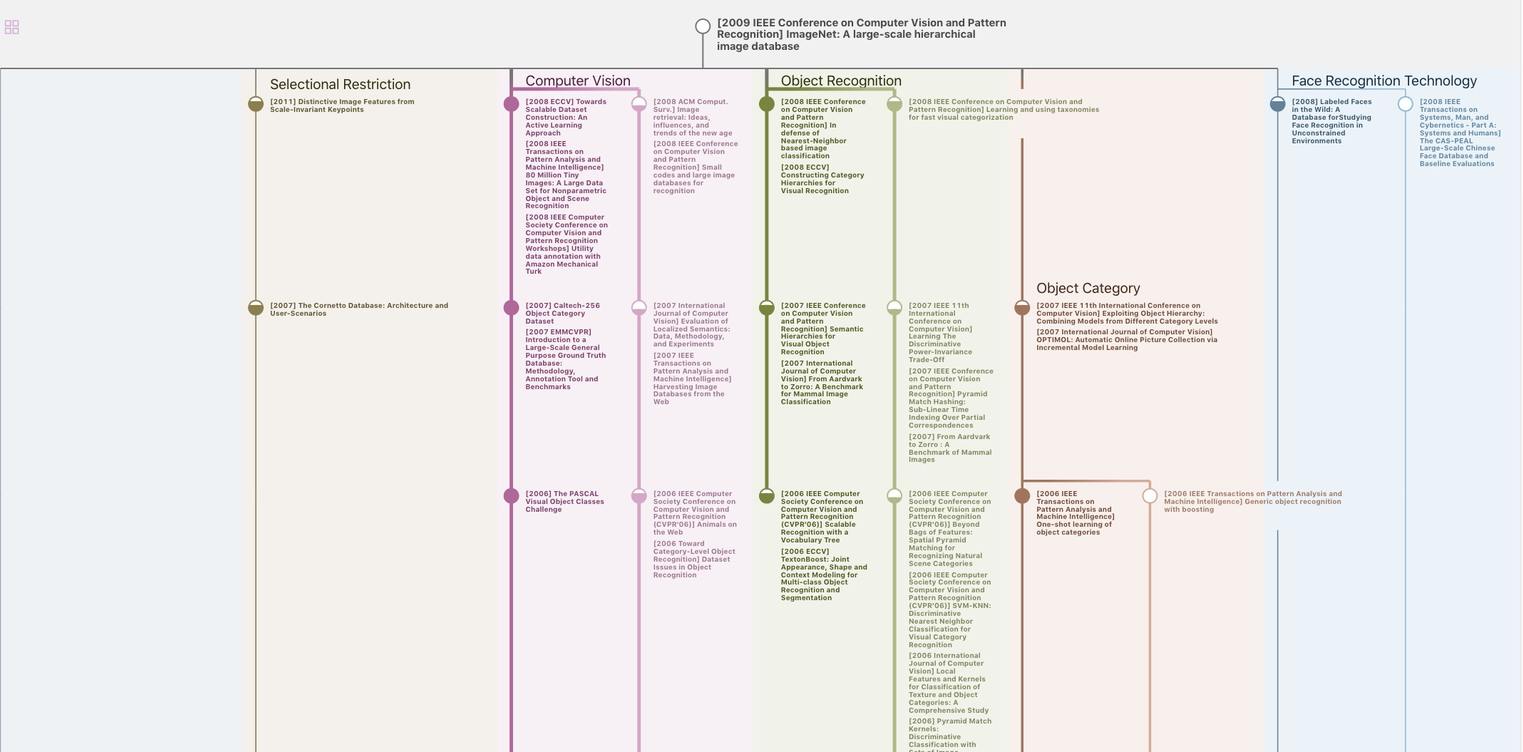
生成溯源树,研究论文发展脉络
Chat Paper
正在生成论文摘要