Multijugate Dual Learning for Low-Resource Task-Oriented Dialogue System
conf_acl(2023)
摘要
Dialogue data in real scenarios tend to be sparsely available, rendering data-starved end-to-end dialogue systems trained inadequately. We discover that data utilization efficiency in low-resource scenarios can be enhanced by mining alignment information uncertain utterance and deterministic dialogue state. Therefore, we innovatively implement dual learning in task-oriented dialogues to exploit the correlation of heterogeneous data. In addition, the one-to-one duality is converted into a multijugate duality to reduce the influence of spurious correlations in dual training for generalization. Without introducing additional parameters, our method could be implemented in arbitrary networks. Extensive empirical analyses demonstrate that our proposed method improves the effectiveness of end-to-end task-oriented dialogue systems under multiple benchmarks and obtains state-of-the-art results in low-resource scenarios.
更多查看译文
关键词
dialogue system,dual,learning,low-resource,task-oriented
AI 理解论文
溯源树
样例
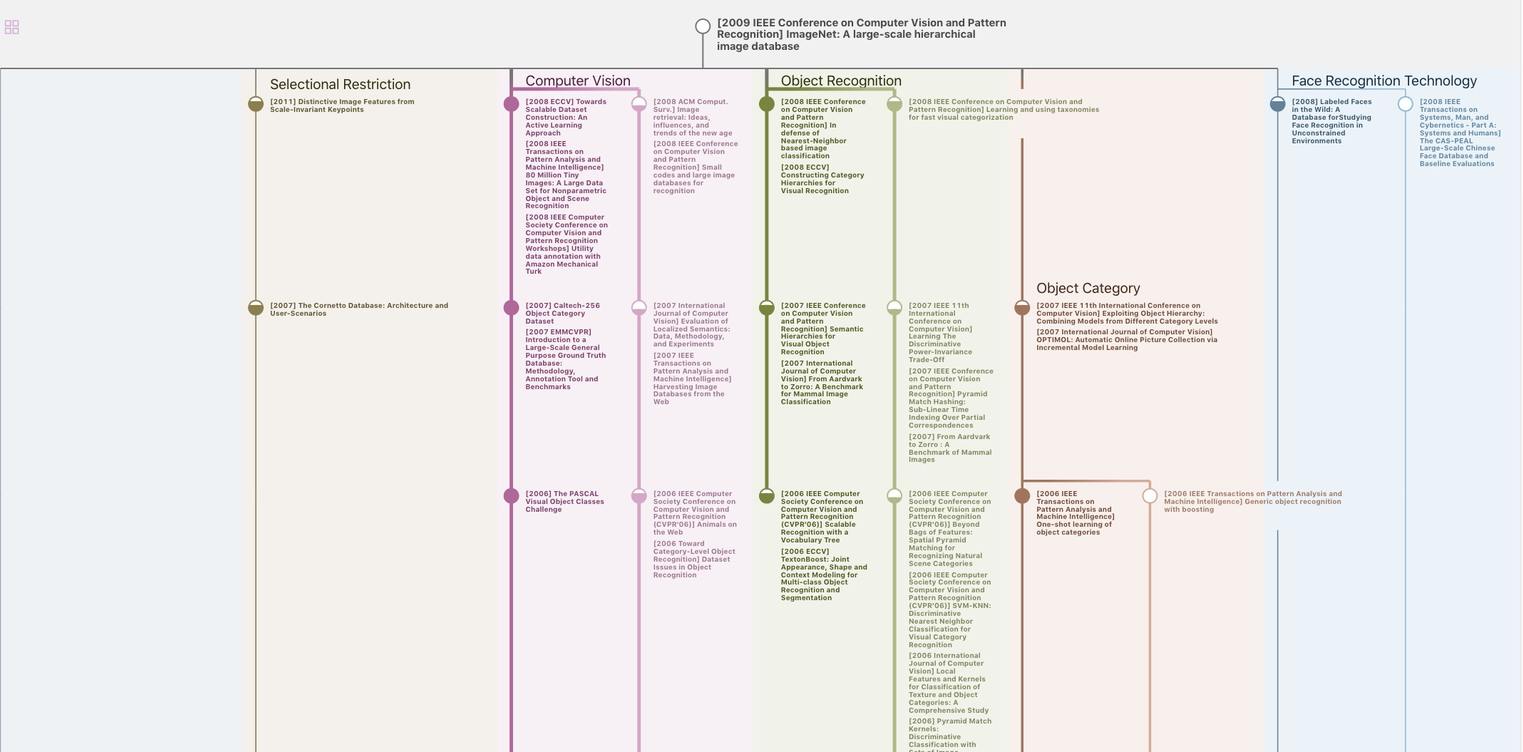
生成溯源树,研究论文发展脉络
Chat Paper
正在生成论文摘要