Non-Log-Concave and Nonsmooth Sampling via Langevin Monte Carlo Algorithms
CoRR(2023)
摘要
We study the problem of approximate sampling from non-log-concave distributions, e.g., Gaussian mixtures, which is often challenging even in low dimensions due to their multimodality. We focus on performing this task via Markov chain Monte Carlo (MCMC) methods derived from discretizations of the overdamped Langevin diffusions, which are commonly known as Langevin Monte Carlo algorithms. Furthermore, we are also interested in two nonsmooth cases for which a large class of proximal MCMC methods have been developed: (i) a nonsmooth prior is considered with a Gaussian mixture likelihood; (ii) a Laplacian mixture distribution. Such nonsmooth and non-log-concave sampling tasks arise from a wide range of applications to Bayesian inference and imaging inverse problems such as image deconvolution. We perform numerical simulations to compare the performance of most commonly used Langevin Monte Carlo algorithms.
更多查看译文
关键词
nonsmooth sampling,langevin monte carlo,non-log-concave
AI 理解论文
溯源树
样例
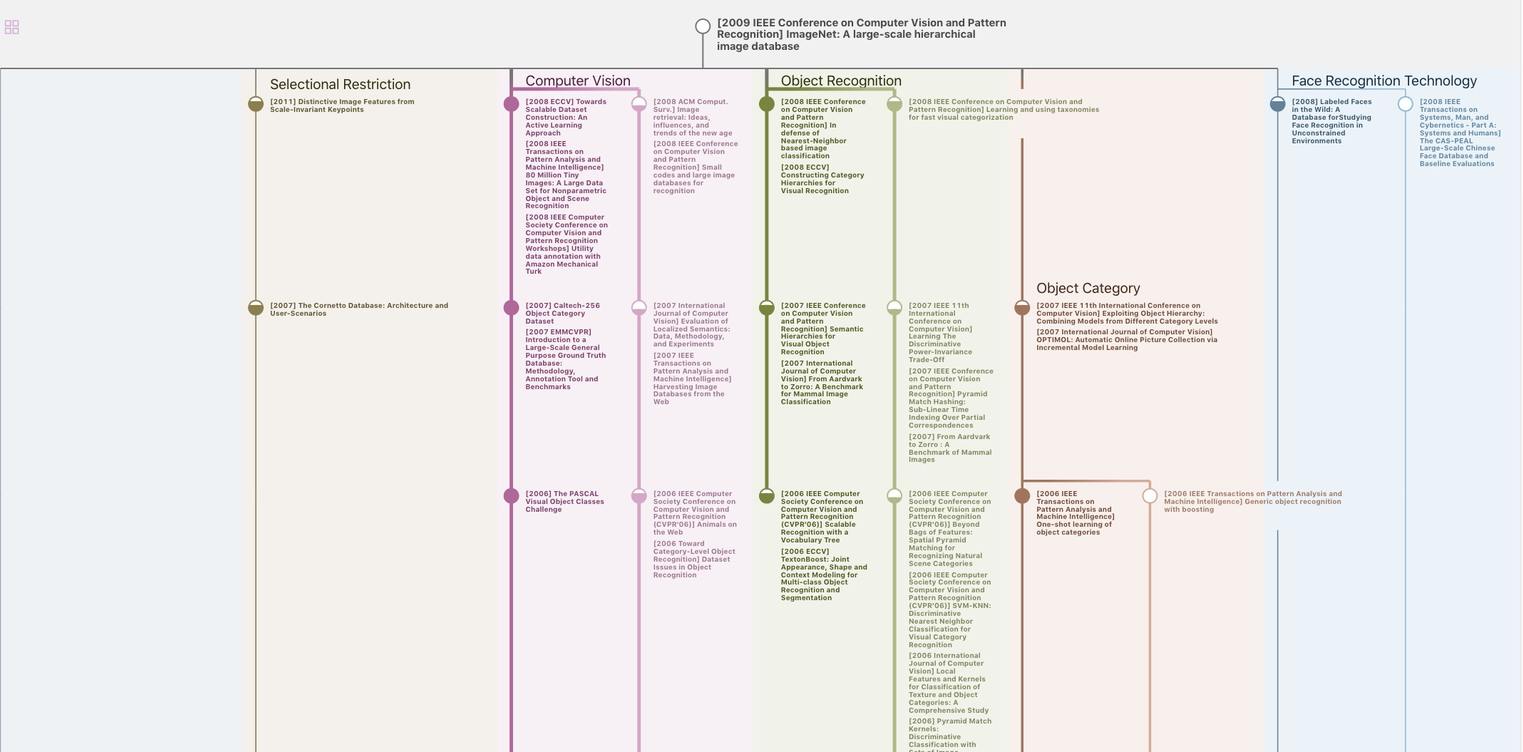
生成溯源树,研究论文发展脉络
Chat Paper
正在生成论文摘要