Empowering Practical Root Cause Analysis by Large Language Models for Cloud Incidents
CoRR(2023)
摘要
Ensuring the reliability and availability of cloud services necessitates efficient root cause analysis (RCA) for cloud incidents. Traditional RCA methods, which rely on manual investigations of data sources such as logs and traces, are often laborious, error-prone, and challenging for on-call engineers. In this paper, we introduce RCACopilot, an innovative On-call system empowered by the Large Language Model for automating RCA of cloud incidents. RCACopilot matches incoming incidents to corresponding handlers based on their alert types, aggregates the critical runtime diagnostic information, predicts the incident's root cause category, and provides an explanatory narrative. We evaluate RCACopilot using a real-world dataset consisting of a year's worth of incidents from serviceX in companyX. Our evaluation demonstrates that RCACopilot achieves RCA accuracy up to 0.766. Furthermore, the diagnostic information collection component of RCACopilot has been successfully in use at companyX for over four years.
更多查看译文
AI 理解论文
溯源树
样例
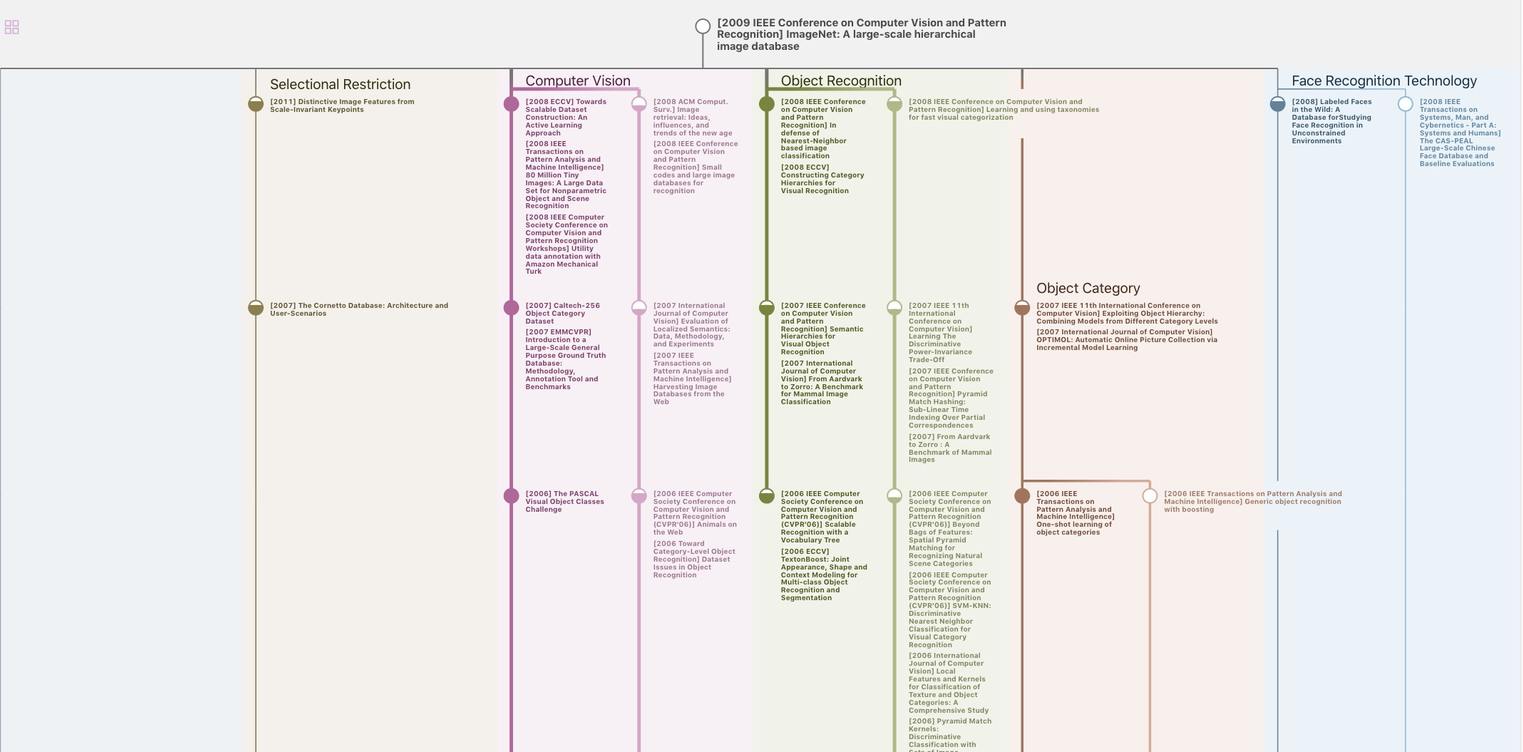
生成溯源树,研究论文发展脉络
Chat Paper
正在生成论文摘要