Perturbation-Based Self-Supervised Attention for Attention Bias in Text Classification
CoRR(2023)
摘要
In text classification, the traditional attention mechanisms usually focus too much on frequent words, and need extensive labeled data in order to learn. This article proposes a perturbation-based self-supervised attention approach to guide attention learning without any annotation overhead. Specifically, we add as much noise as possible to all the words in the sentence without changing their semantics and predictions. We hypothesize that words that tolerate more noise are less significant, and we can use this information to refine the attention distribution. Experimental results on three text classification tasks show that our approach can significantly improve the performance of current attention-based models, and is more effective than existing self-supervised methods. We also provide a visualization analysis to verify the effectiveness of our approach.
更多查看译文
关键词
Attention bias,perturbation,self-supervised learning,text classification
AI 理解论文
溯源树
样例
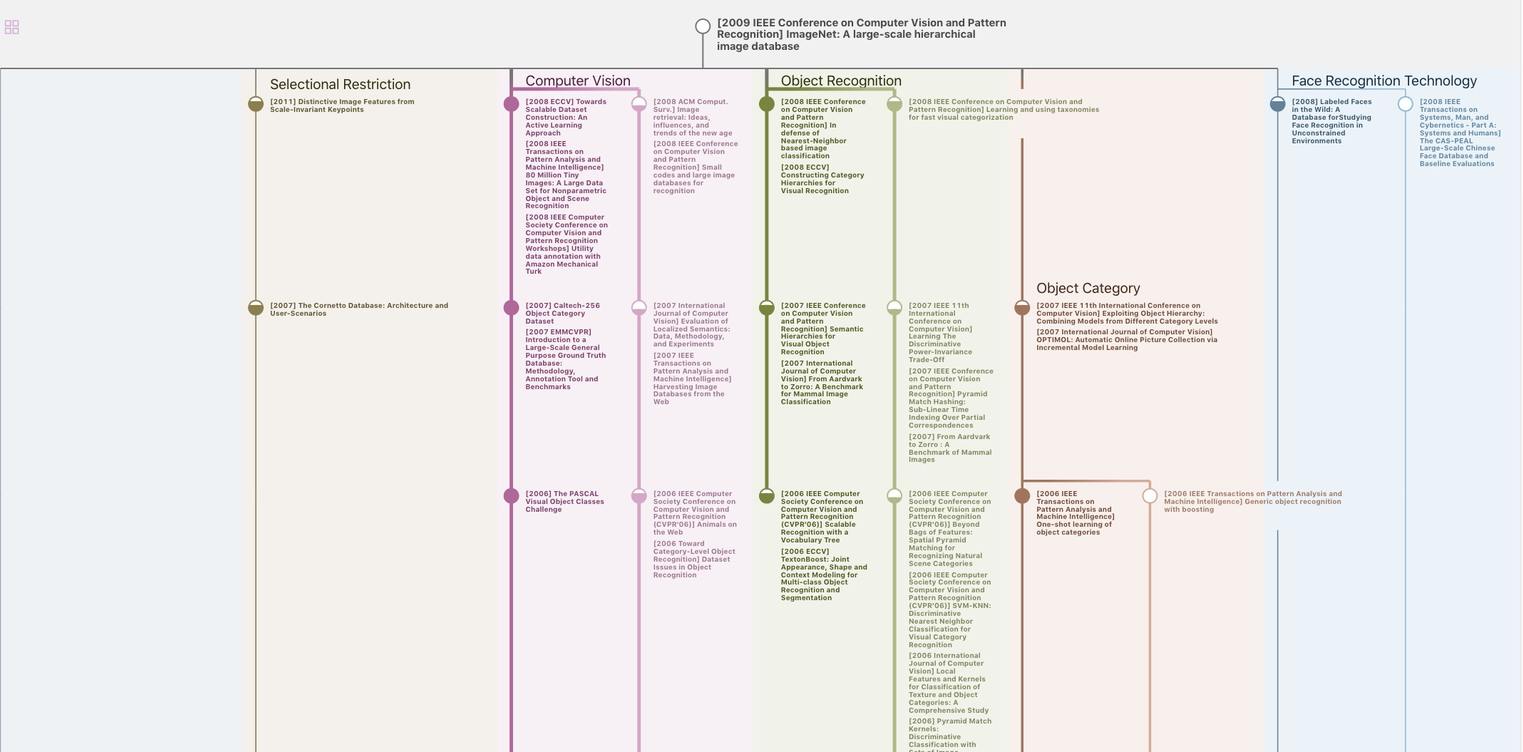
生成溯源树,研究论文发展脉络
Chat Paper
正在生成论文摘要