BookGPT: A General Framework for Book Recommendation Empowered by Large Language Model
ELECTRONICS(2023)
摘要
With the continuous development and change exhibited by large language model (LLM) technology, represented by generative pretrained transformers (GPTs), many classic scenarios in various fields have re-emerged with new opportunities. This paper takes ChatGPT as the modeling object, incorporates LLM technology into the typical book resource understanding and recommendation scenario for the first time, and puts it into practice. By building a ChatGPT-like book recommendation system (BookGPT) framework based on ChatGPT, this paper attempts to apply ChatGPT to recommendation modeling for three typical tasks: book rating recommendation, user rating recommendation, and the book summary recommendation; it also explores the feasibility of LLM technology in book recommendation scenarios. At the same time, based on different evaluation schemes for book recommendation tasks and the existing classic recommendation models, this paper discusses the advantages and disadvantages of the BookGPT in book recommendation scenarios and analyzes the opportunities and improvement directions for subsequent LLMs in these scenarios. The experimental research shows the following: (1) The BookGPT can achieve good recommendation results in existing classic book recommendation tasks. Especially in cases containing less information about the target object to be recommended, such as zero-shot or one-shot learning tasks, the performance of the BookGPT is close to or even better than that of the current classic book recommendation algorithms, and this method has great potential for improvement. (2) In text generation tasks such as book summary recommendation, the recommendation effect of the BookGPT model is better than that of the manual editing process of Douban Reading, and it can even perform personalized interpretable content recommendations based on readers' attribute and identity information, making it more persuasive than interpretable one-size-fits-all recommendation models. Finally, we have open-sourced the relevant datasets and experimental codes, hoping that the exploratory program proposed in this paper can inspire the development of more LLMs to expand their applications and theoretical research prospects in the field of book recommendation and general recommendation tasks.
更多查看译文
关键词
book recommendation,large language model,general recommendation
AI 理解论文
溯源树
样例
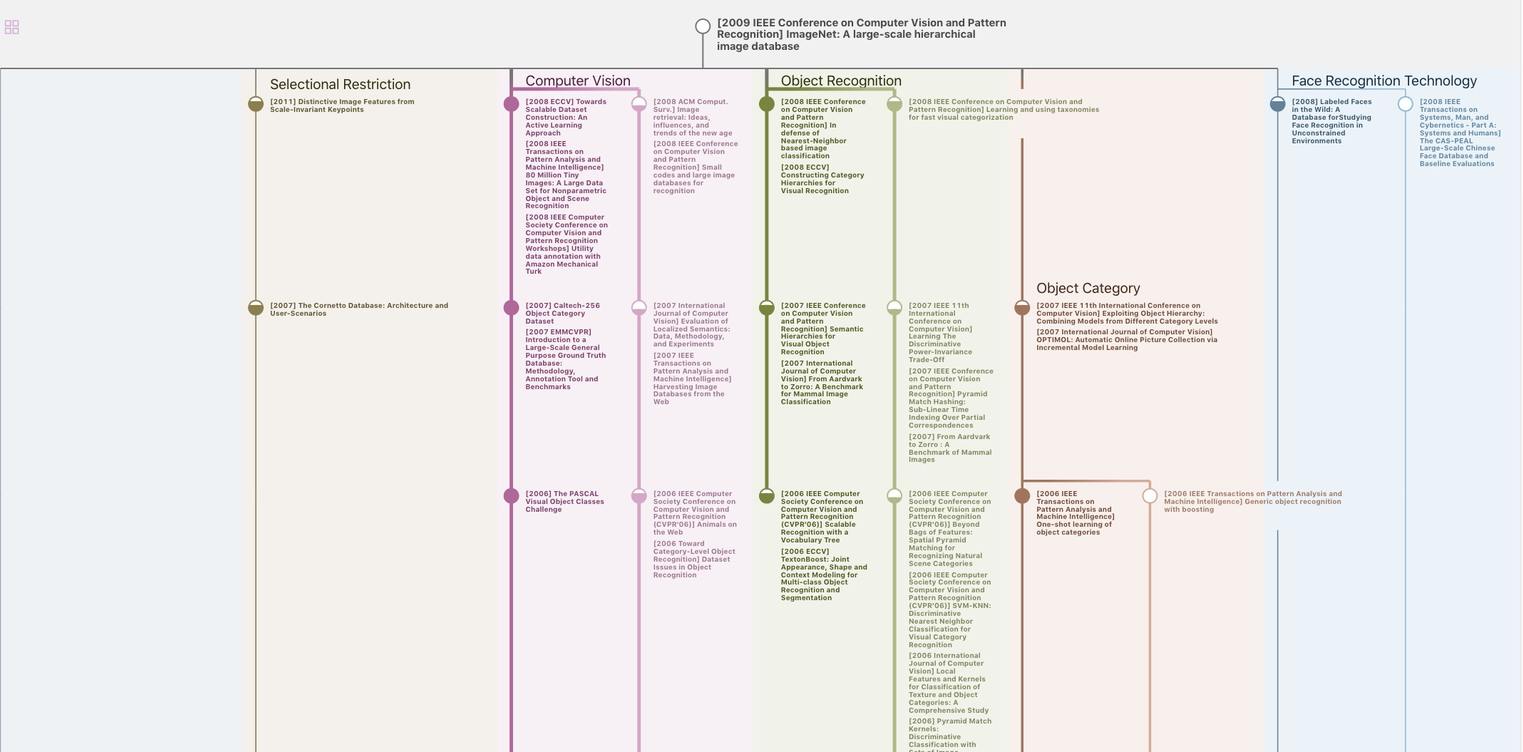
生成溯源树,研究论文发展脉络
Chat Paper
正在生成论文摘要