Matrix Autoregressive Model with Vector Time Series Covariates for Spatio-Temporal Data
arXiv (Cornell University)(2023)
摘要
We develop a new methodology for forecasting matrix-valued time series with
historical matrix data and auxiliary vector time series data. We focus on time
series of matrices with observations distributed on a fixed 2-D spatial grid,
i.e., the spatio-temporal data, and an auxiliary time series of non-spatial
vectors. The proposed model, Matrix AutoRegression with Auxiliary Covariates
(MARAC), contains an autoregressive component for the historical matrix
predictors and an additive component that maps the auxiliary vector predictors
to a matrix response via tensor-vector product. The autoregressive component
adopts a bi-linear transformation framework following Chen et al. (2021),
significantly reducing the number of parameters. The auxiliary component posits
that the tensor coefficient, which maps non-spatial predictors to a spatial
response, contains slices of spatially-smooth matrix coefficients that are
discrete evaluations of smooth functions from a Reproducible Kernel Hilbert
Space (RKHS). We propose to estimate the model parameters under a penalized
maximum likelihood estimation framework coupled with an alternating
minimization algorithm. We establish the joint asymptotics of the
autoregressive and tensor parameters under fixed and high-dimensional regimes.
Extensive simulations and a geophysical application for forecasting the global
Total Electron Content (TEC) are conducted to validate the performance of
MARAC.
更多查看译文
关键词
vector time series covariates,time series,matrix,spatio-temporal
AI 理解论文
溯源树
样例
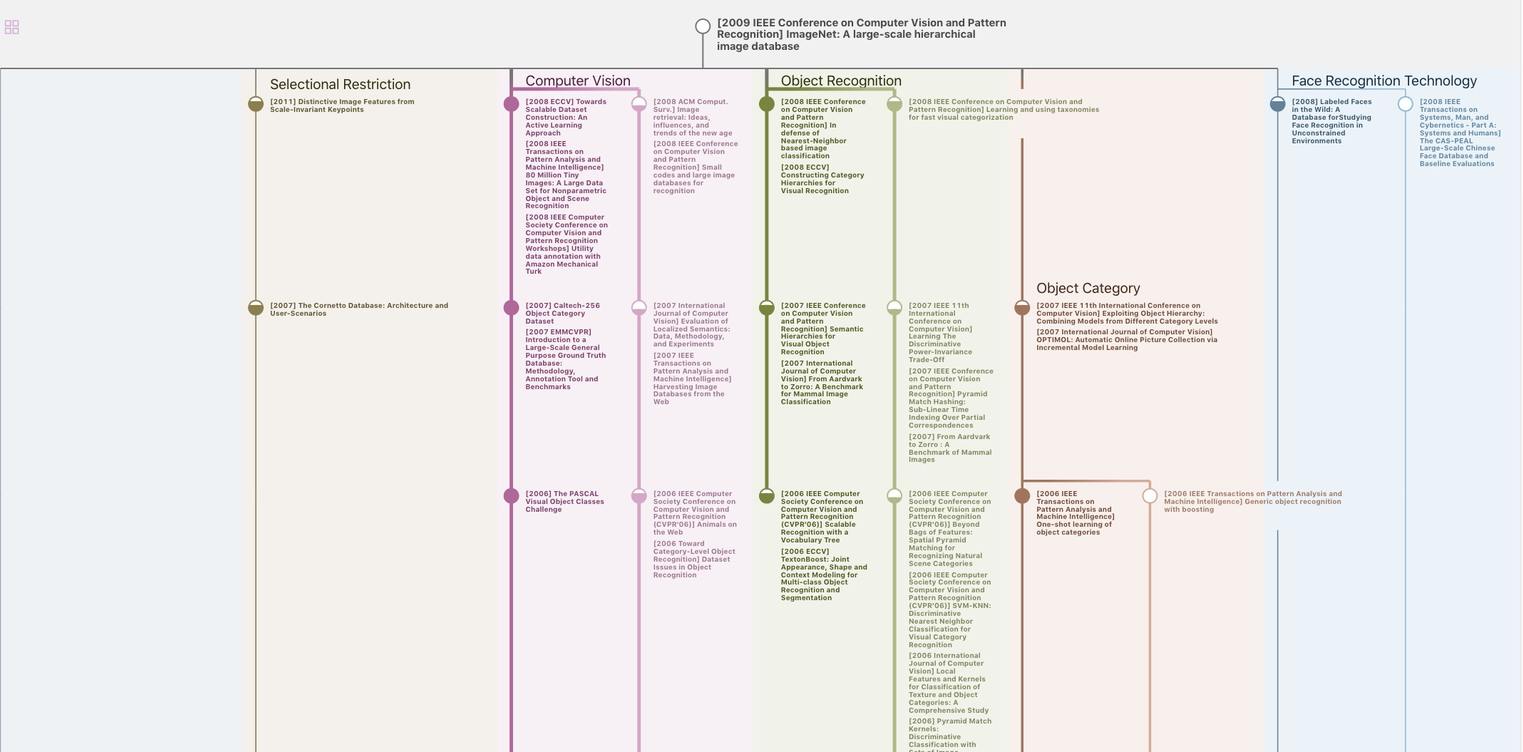
生成溯源树,研究论文发展脉络
Chat Paper
正在生成论文摘要