Unsupervised Semantic Correspondence Using Stable Diffusion
NeurIPS(2023)
摘要
Text-to-image diffusion models are now capable of generating images that are often indistinguishable from real images. To generate such images, these models must understand the semantics of the objects they are asked to generate. In this work we show that, without any training, one can leverage this semantic knowledge within diffusion models to find semantic correspondences -- locations in multiple images that have the same semantic meaning. Specifically, given an image, we optimize the prompt embeddings of these models for maximum attention on the regions of interest. These optimized embeddings capture semantic information about the location, which can then be transferred to another image. By doing so we obtain results on par with the strongly supervised state of the art on the PF-Willow dataset and significantly outperform (20.9% relative for the SPair-71k dataset) any existing weakly or unsupervised method on PF-Willow, CUB-200 and SPair-71k datasets.
更多查看译文
关键词
correspondence,semantic,stable diffusion
AI 理解论文
溯源树
样例
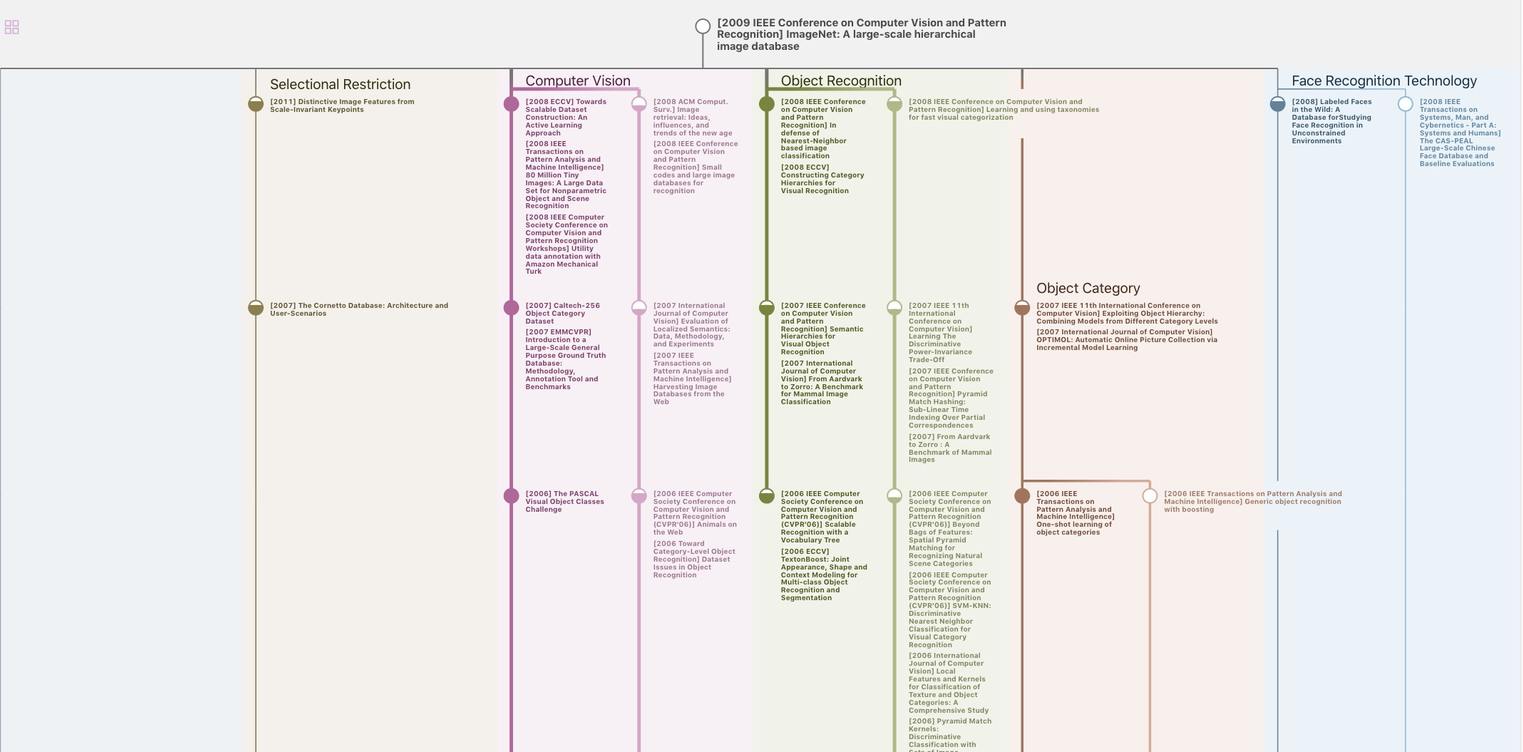
生成溯源树,研究论文发展脉络
Chat Paper
正在生成论文摘要