MultiRes Attention Deep Learning Approach for Abdominal Fat Compartment Segmentation and Quantification
Deep Learning and Reinforcement Learning [Working Title] Artificial Intelligence(2023)
摘要
Global increase in obesity has led to alarming rise in co-morbidities leading to deteriorated quality of life. Obesity phenotyping benefits profiling and management of the condition but warrants accurate quantification of fat compartments. Manual quantification MR scans are time consuming and laborious. Hence, many studies rely on semi/automatic methods for quantification of abdominal fat compartments. We propose a MultiRes-Attention U-Net with hybrid loss function for segmentation of different abdominal fata compartments namely (i) Superficial subcutaneous adipose tissue (SSAT), (ii) Deep subcutaneous adipose tissue (DSAT), and (iii) Visceral adipose tissue (VAT) using abdominal MR scans. MultiRes block, ResAtt-Path, and attention gates can handle shape, scale, and heterogeneity in the data. Dataset involved MR scans from 190 community-dwelling older adults (mainly Chinese, 69.5% females) with mean age—67.85 ± 7.90 years), BMI 23.75 ± 3.65 kg/m2. Twenty-six datasets were manually segmented to generate the ground truth. Data augmentations were performed using MR data acquisition variations. Training and validation were performed on 105 datasets, while testing was conducted on 25 datasets. Median Dice scores were 0.97 for SSAT & DSAT and 0.96 for VAT, and mean Hausdorff distance was <5 mm for all the three fat compartments. Further, MultiRes-Attention U-Net was tested on a new 190 datasets (unseen during training; upper & lower abdomen scans with different resolution), which yielded accurate results. MultiRes-Attention U-Net significantly improved the performance over MultiResUNet, showed excellent generalization and holds promise for body-profiling in large cohort studies.
更多查看译文
关键词
abdominal fat compartment segmentation,attention
AI 理解论文
溯源树
样例
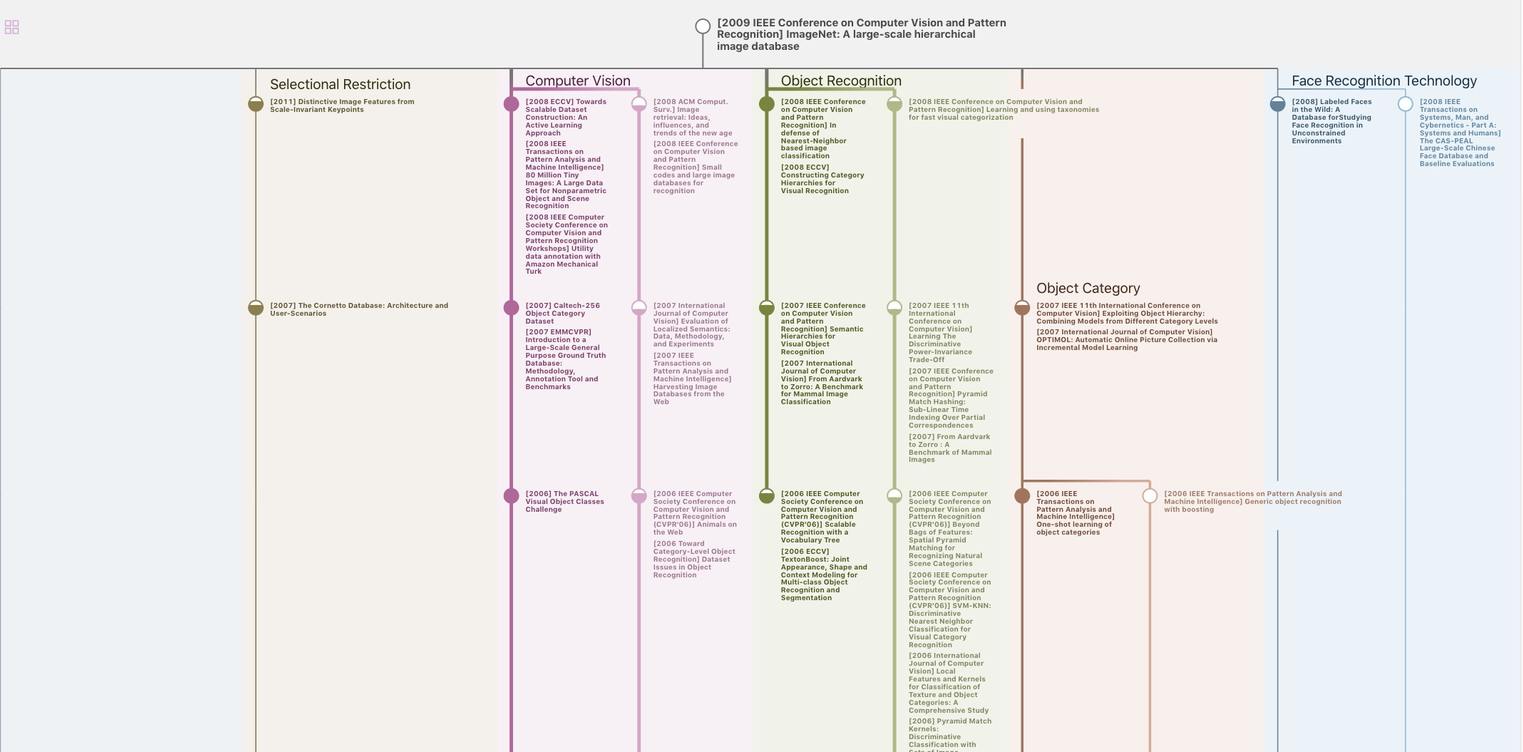
生成溯源树,研究论文发展脉络
Chat Paper
正在生成论文摘要