The Hardness of Learning Access Control Policies
PROCEEDINGS OF THE 28TH ACM SYMPOSIUM ON ACCESS CONTROL MODELS AND TECHNOLOGIES, SACMAT 2023(2023)
摘要
The problem of learning access control policies is receiving increasing attention in research. We contribute to the foundations of this problem by posing and addressing meaningful questions on computational hardness. Our work addresses learning access control policies in the context of three different models from the literature: the access matrix, and Role- and Relationship-Based Access Control (RBAC and ReBAC, respectively). Our underlying theory is the well-established notion of Probably Approximately Correct (PAC), with careful extensions for our setting. The data, or examples, a learning algorithm is provided in our setup is that related to access enforcement, which is the process by which a request for access to a resource is decided. For the access matrix, we pose a learning problem that turns out to be computationally easy, and another that we prove is computationally hard. We generalize the former result so we have a sufficient condition for establishing other problems to be computationally easy. With these results as the basis, we consider five learning problems in the context of RBAC, two of which turn out to be computationally hard. Finally, we consider four learning problems in the context of ReBAC, all of which turn out to be computationally easy. Every proof for a problem that is computationally easy is constructive, in that we propose a learning algorithm for the problem that is efficient, and probably, approximately correct. As such, our work makes contributions at the foundations of an important, emerging aspect of access control, and thereby, information security.
更多查看译文
关键词
Probably approximately correct,Computational hardness
AI 理解论文
溯源树
样例
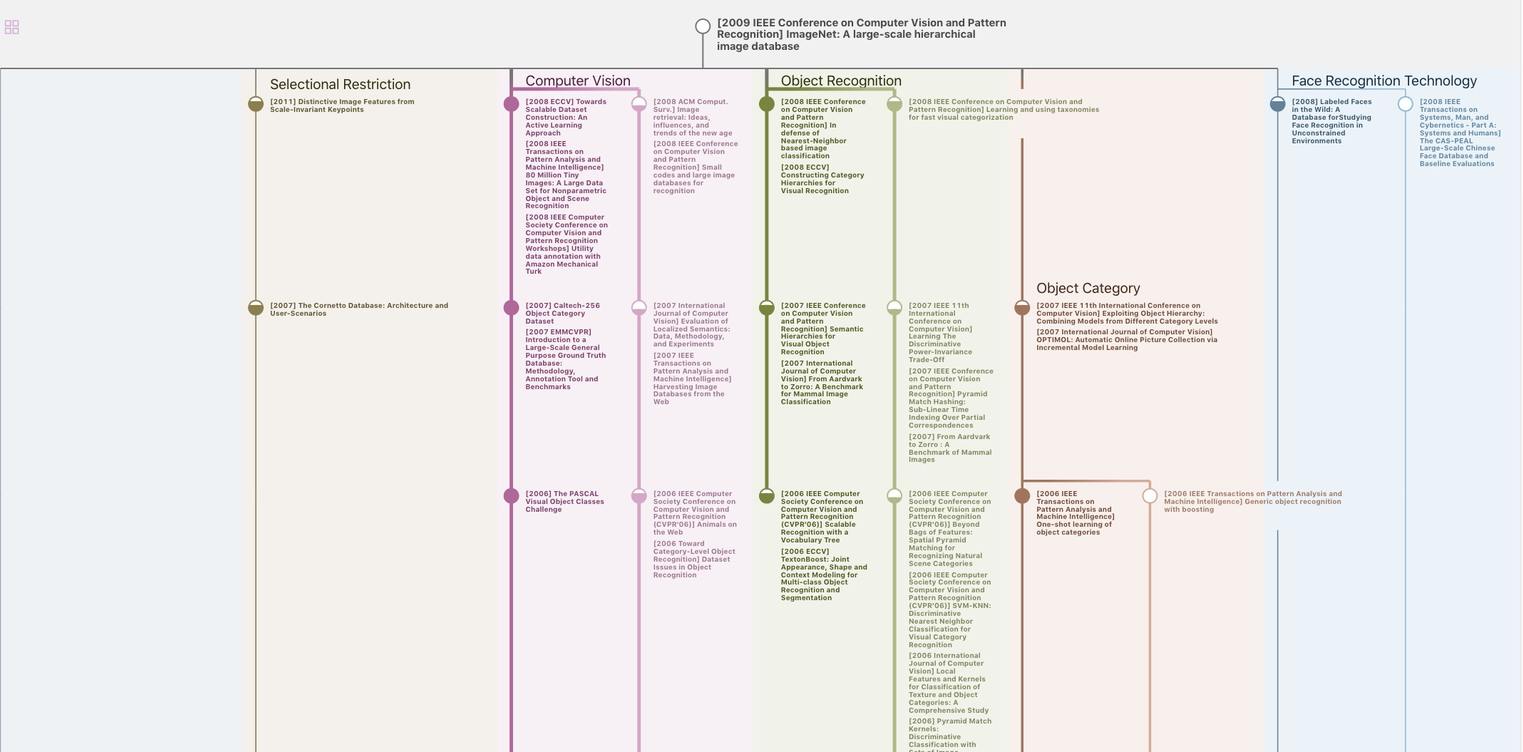
生成溯源树,研究论文发展脉络
Chat Paper
正在生成论文摘要