Deep Learning–Based Computer-Aided Diagnosis for Breast Lesion Classification on Ultrasound: A Prospective Multicenter Study of Radiologists Without Breast Ultrasound Expertise
American Journal of Roentgenology(2023)
摘要
Computer-aided diagnosis (CAD) systems for breast ultrasound interpretation have been primarily evaluated at tertiary and/or urban medical centers by radiologists with breast ultrasound expertise. To evaluate the utility of deep learning-based CAD software on the diagnostic performance of radiologists without breast ultrasound expertise at secondary or rural hospitals in the differentiation of benign and malignant breast lesions measuring up to 2.0 cm on ultrasound. This prospective study included patients scheduled to undergo biopsy or surgical resection at any of eight participating secondary or rural hospitals in China of a breast lesion classified as BI-RADS category 3-5 on prior breast ultrasound from November 2021 to September 2022. Patients underwent an additional investigational breast ultrasound, performed and interpreted by a radiologist without breast ultrasound expertise (hybrid body-breast radiologists, either who lacked breast imaging subspecialty training or in whom the number of breast ultrasounds performed annually accounted for less than 10% of all ultrasounds performed annually by the radiologist), who assigned a BI-RADS category. CAD results were used to upgrade reader-assigned BI-RADS category 3 lesions to category 4A, and to downgrade reader-assigned BI-RADS category 4A lesions to category 3. Histologic results of biopsy or resection served as reference standard. The study included 313 patients (mean age, 47.0±14.0 years) with 313 breast lesions (102 malignant, 211 benign). Of BI-RADS category 3 lesions, 6.0% (6/100) were upgraded by CAD to category 4A, of which 16.7% (1/6) were malignant. Of category 4A lesions, 79.1% (87/110) were downgraded by CAD to category 3, of which 4.6% (4/87) were malignant. Diagnostic performance was significantly better after application of CAD, in comparison with before application of CAD, in terms of accuracy (86.6% vs 62.6%; p<.001), specificity (82.9% vs 46.0%; p<.001), and PPV (72.7% vs 46.5%; p<.001), but not significantly different in terms of sensitivity (94.1% vs 97.1%, p=.38) or NPV (96.7% vs 97.0%, p>.99). CAD significantly improved radiologists' diagnostic performance, showing particular potential to reduce the frequency of benign breast biopsies. The findings indicate the ability of CAD to improve patient care in settings with incomplete access to breast imaging expertise.
更多查看译文
关键词
breast lesion classification,deep learning–based,ultrasound,radiologists,computer-aided
AI 理解论文
溯源树
样例
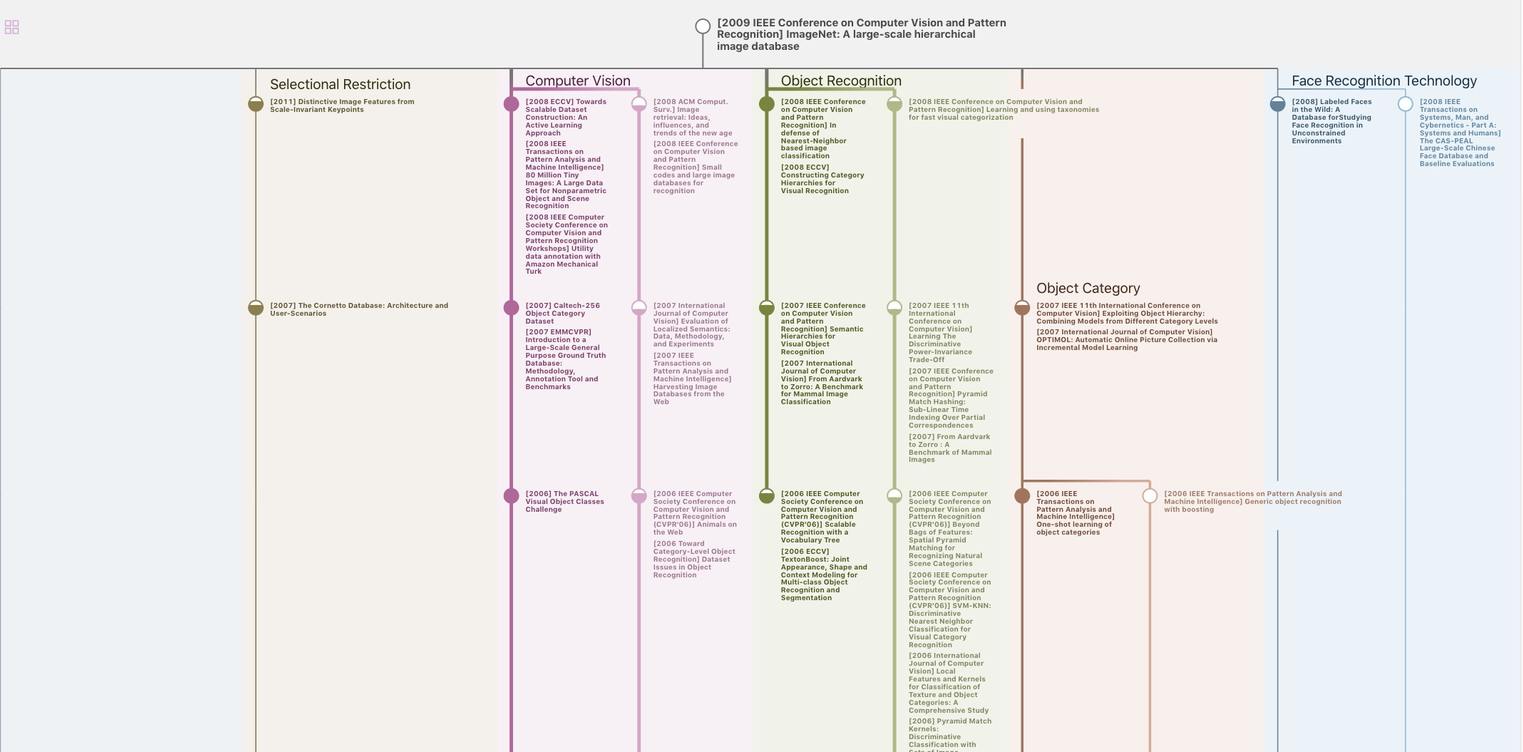
生成溯源树,研究论文发展脉络
Chat Paper
正在生成论文摘要