Discriminative analysis dictionary learning with adaptively ordinal locality preserving
Neural Networks(2023)
摘要
Dictionary learning has found broad applications in signal and image processing. By adding constraints to the traditional dictionary learning model, dictionaries with discriminative capability can be obtained which can deal with the task of image classification. The Discriminative Convolutional Analysis Dictionary Learning (DCADL) algorithm proposed recently has achieved promising results with low computational complexity. However, DCADL is still limited in classification performance because of the lack of constraints on dictionary structures. To solve this problem, this study introduces an adaptively ordinal locality preserving (AOLP) term to the original model of DCADL to further improve the classification performance. With the AOLP term, the distance ranking in the neighborhood of each atom can be preserved, which can improve the discrimination of coding coefficients. In addition, a linear classifier for the classification of coding coefficients is trained along with the dictionary. A new method is designed specifically to solve the optimization problem corresponding to the proposed model. Experiments are performed on several commonly used datasets to show the promising results of the proposed algorithm in classification performance and computational efficiency.
更多查看译文
关键词
Discriminative dictionary learning, Ordinal locality preserving, Analysis dictionary learning, Image classification
AI 理解论文
溯源树
样例
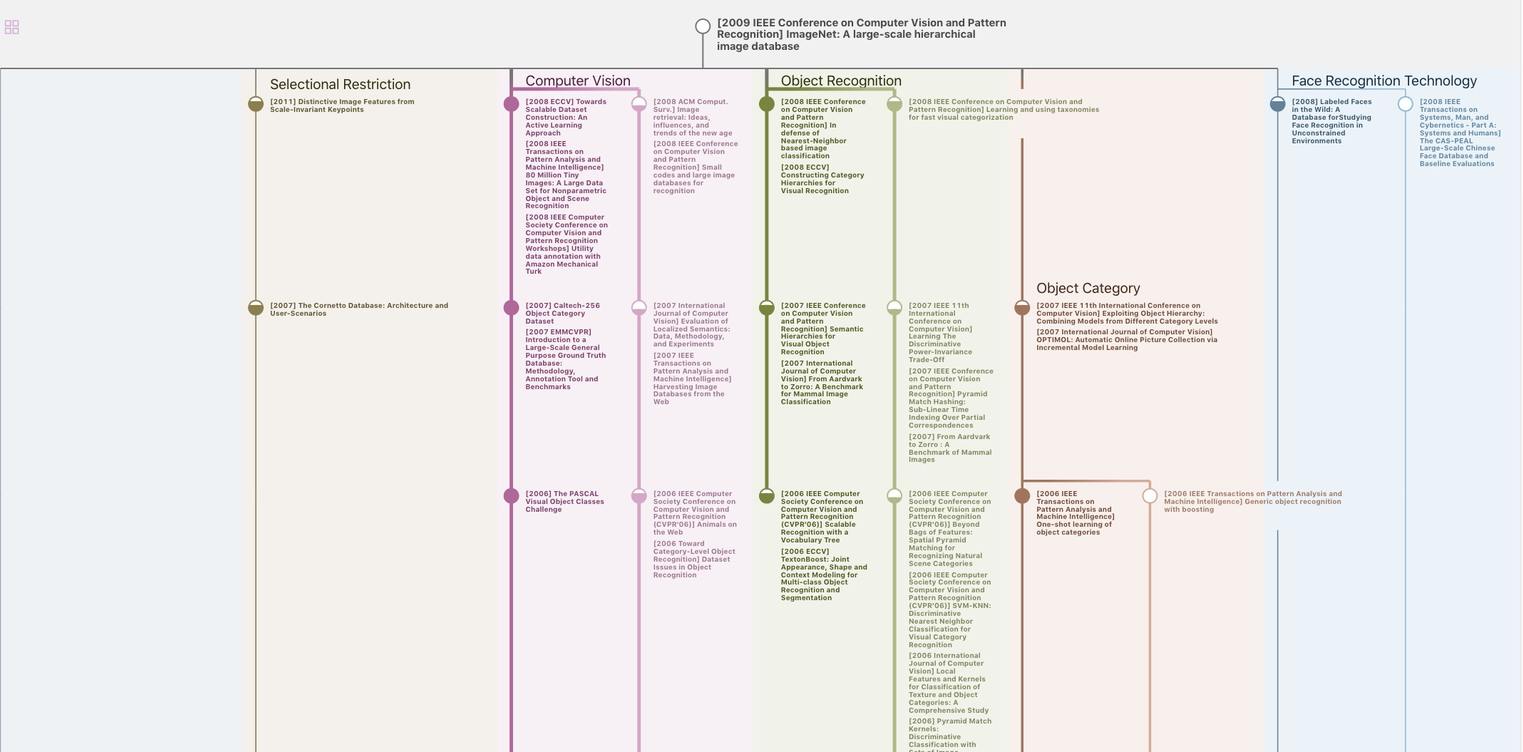
生成溯源树,研究论文发展脉络
Chat Paper
正在生成论文摘要