Unit-based Speech-to-Speech Translation Without Parallel Data
CoRR(2023)
摘要
We propose an unsupervised speech-to-speech translation (S2ST) system that does not rely on parallel data between the source and target languages. Our approach maps source and target language speech signals into automatically discovered, discrete units and reformulates the problem as unsupervised unit-to-unit machine translation. We develop a three-step training procedure that involves (a) pre-training an unit-based encoder-decoder language model with a denoising objective (b) training it with word-by-word translated utterance pairs created by aligning monolingual text embedding spaces and (c) running unsupervised backtranslation bootstrapping off of the initial translation model. Our approach avoids mapping the speech signal into text and uses speech-to-unit and unit-to-speech models instead of automatic speech recognition and text to speech models. We evaluate our model on synthetic-speaker Europarl-ST English-German and German-English evaluation sets, finding that unit-based translation is feasible under this constrained scenario, achieving 9.29 ASR-BLEU in German to English and 8.07 in English to German.
更多查看译文
关键词
parallel data,translation,unit-based,speech-to-speech
AI 理解论文
溯源树
样例
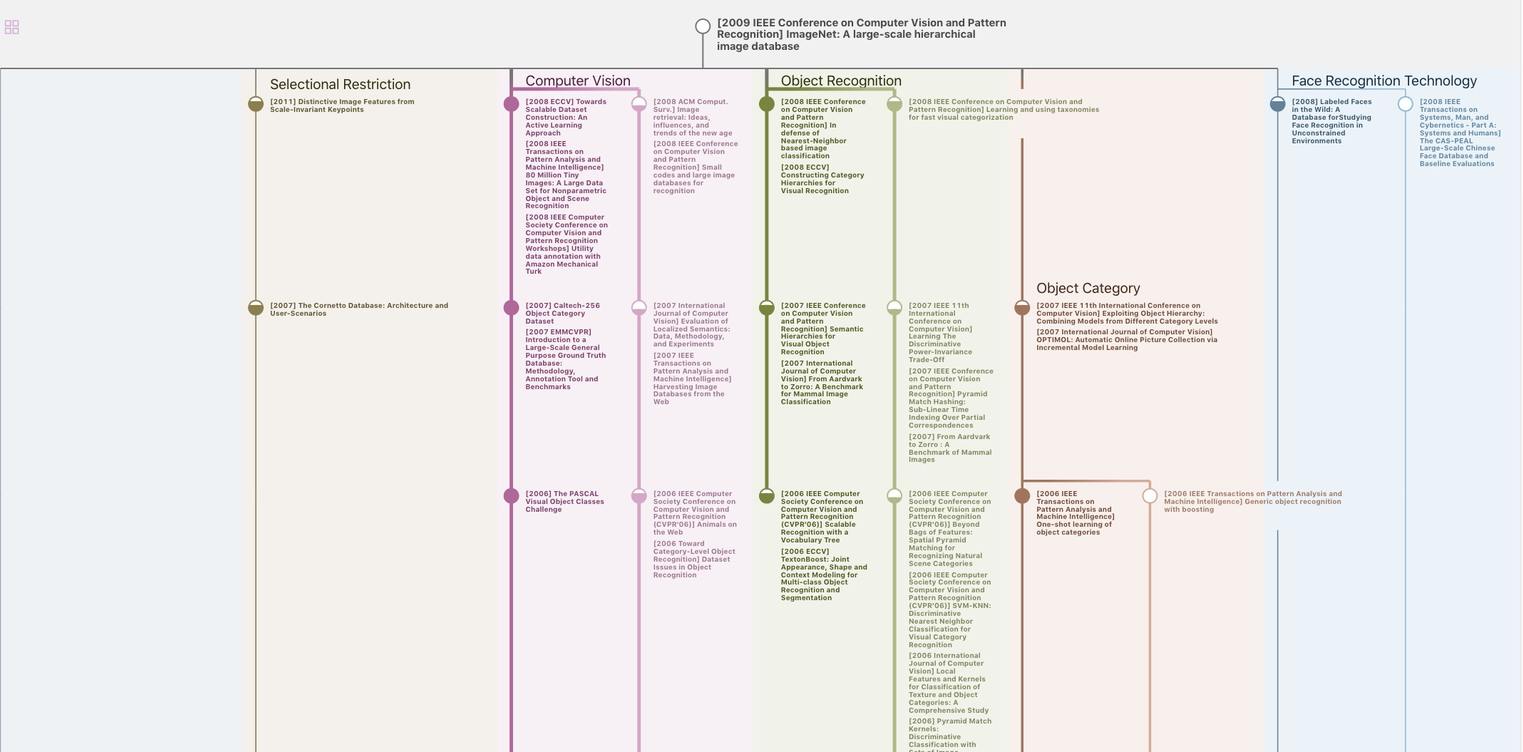
生成溯源树,研究论文发展脉络
Chat Paper
正在生成论文摘要