Collaborative World Models: An Online-Offline Transfer RL Approach
CoRR(2023)
摘要
Training visual reinforcement learning (RL) models in offline datasets is challenging due to overfitting issues in representation learning and overestimation problems in value function. In this paper, we propose a transfer learning method called Collaborative World Models (CoWorld) to improve the performance of visual RL under offline conditions. The core idea is to use an easy-to-interact, off-the-shelf simulator to train an auxiliary RL model as the online ``test bed'' for the offline policy learned in the target domain, which provides a flexible constraint for the value function -- Intuitively, we want to mitigate the overestimation problem of value functions outside the offline data distribution without impeding the exploration of actions with potential advantages. Specifically, CoWorld performs domain-collaborative representation learning to bridge the gap between online and offline hidden state distributions. Furthermore, it performs domain-collaborative behavior learning that enables the source RL agent to provide target-aware value estimation, allowing for effective offline policy regularization. Experiments show that CoWorld significantly outperforms existing methods in offline visual control tasks in DeepMind Control and Meta-World.
更多查看译文
关键词
models,transfer,world,online-offline
AI 理解论文
溯源树
样例
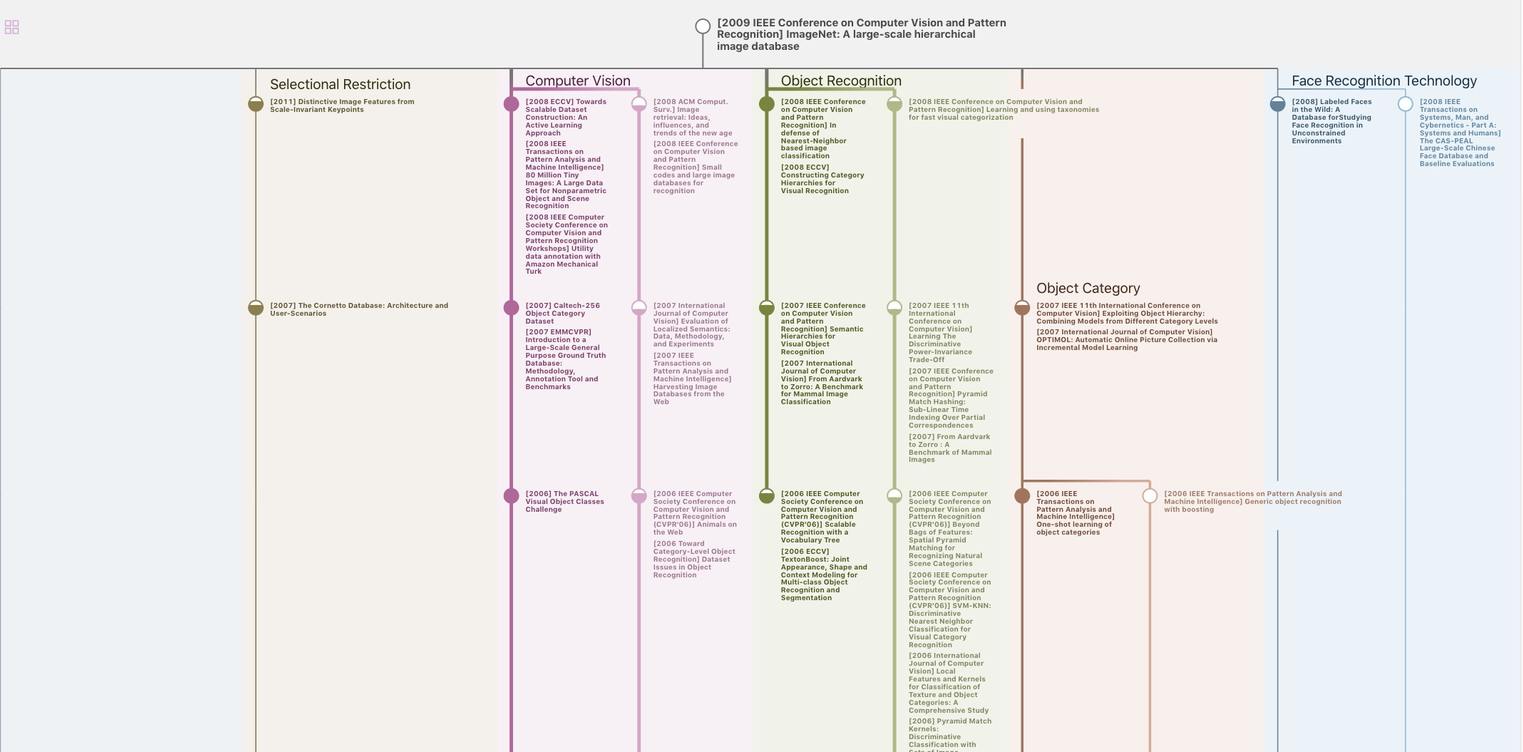
生成溯源树,研究论文发展脉络
Chat Paper
正在生成论文摘要