PIVOINE: Instruction Tuning for Open-world Information Extraction
CoRR(2023)
摘要
We consider the problem of Open-world Information Extraction (Open-world IE), which extracts comprehensive entity profiles from unstructured texts. Different from the conventional closed-world setting of Information Extraction (IE), Open-world IE considers a more general situation where entities and relations could be beyond a predefined ontology. More importantly, we seek to develop a large language model (LLM) that is able to perform Open-world IE to extract desirable entity profiles characterized by (possibly fine-grained) natural language instructions. We achieve this by finetuning LLMs using instruction tuning. In particular, we construct INSTRUCTOPENWIKI, a substantial instruction tuning dataset for Open-world IE enriched with a comprehensive corpus, extensive annotations, and diverse instructions. We finetune the pretrained BLOOM models on INSTRUCTOPENWIKI and obtain PIVOINE, an LLM for Open-world IE with strong instruction-following capabilities. Our experiments demonstrate that PIVOINE significantly outperforms traditional closed-world methods and other LLM baselines, displaying impressive generalization capabilities on both unseen instructions and out-of-ontology cases. Consequently, PIVOINE emerges as a promising solution to tackle the open-world challenge in IE effectively.
更多查看译文
关键词
instruction tuning,extraction,information,open-world
AI 理解论文
溯源树
样例
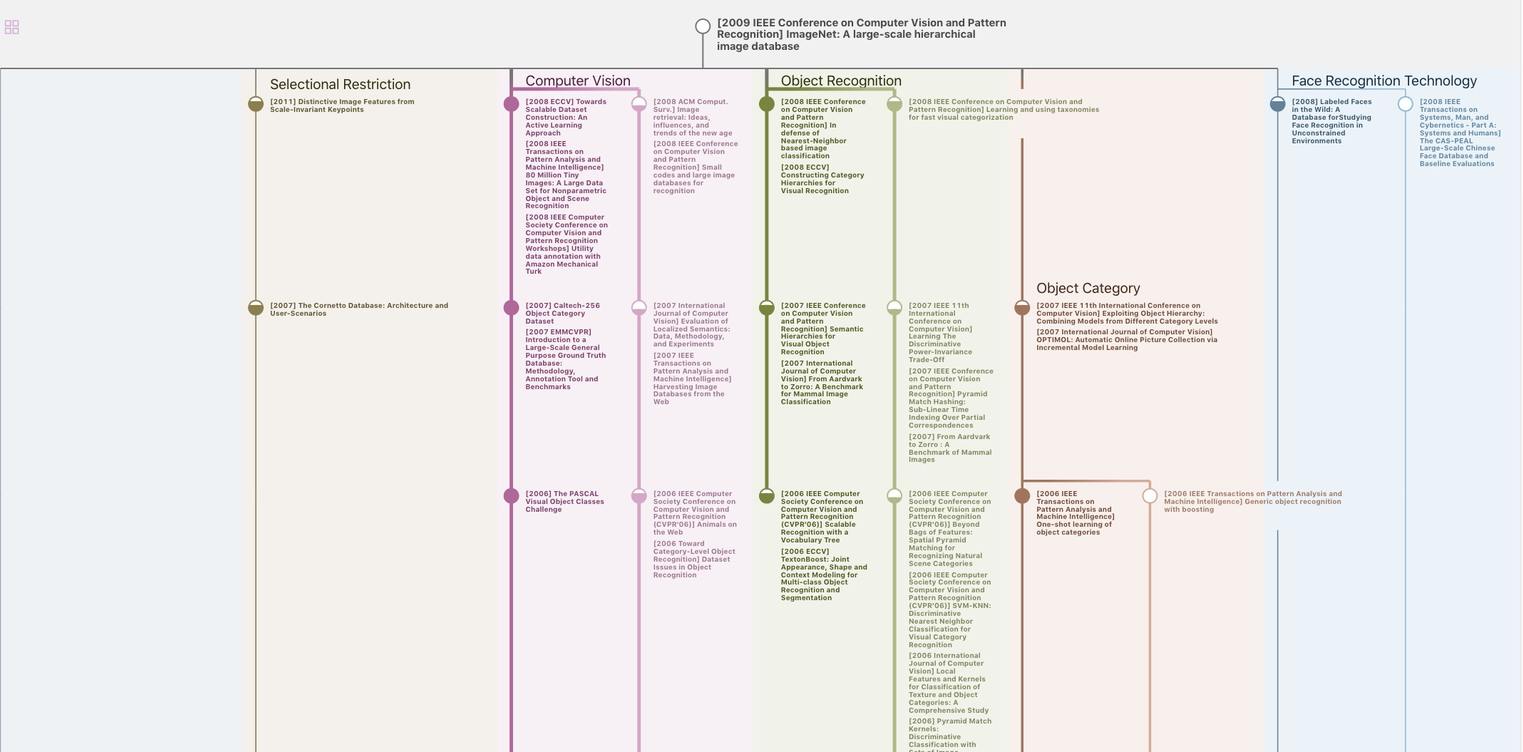
生成溯源树,研究论文发展脉络
Chat Paper
正在生成论文摘要