AdvFunMatch: When Consistent Teaching Meets Adversarial Robustness
CoRR(2023)
摘要
\emph{Consistent teaching} is an effective paradigm for implementing knowledge distillation (KD), where both student and teacher models receive identical inputs, and KD is treated as a function matching task (FunMatch). However, one limitation of FunMatch is that it does not account for the transfer of adversarial robustness, a model's resistance to adversarial attacks. To tackle this problem, we propose a simple but effective strategy called Adversarial Function Matching (AdvFunMatch), which aims to match distributions for all data points within the $\ell_p$-norm ball of the training data, in accordance with consistent teaching. Formulated as a min-max optimization problem, AdvFunMatch identifies the worst-case instances that maximizes the KL-divergence between teacher and student model outputs, which we refer to as "mismatched examples," and then matches the outputs on these mismatched examples. Our experimental results show that AdvFunMatch effectively produces student models with both high clean accuracy and robustness. Furthermore, we reveal that strong data augmentations (\emph{e.g.}, AutoAugment) are beneficial in AdvFunMatch, whereas prior works have found them less effective in adversarial training. Code is available at \url{https://gitee.com/zihui998/adv-fun-match}.
更多查看译文
关键词
adversarial robustness,consistent teaching
AI 理解论文
溯源树
样例
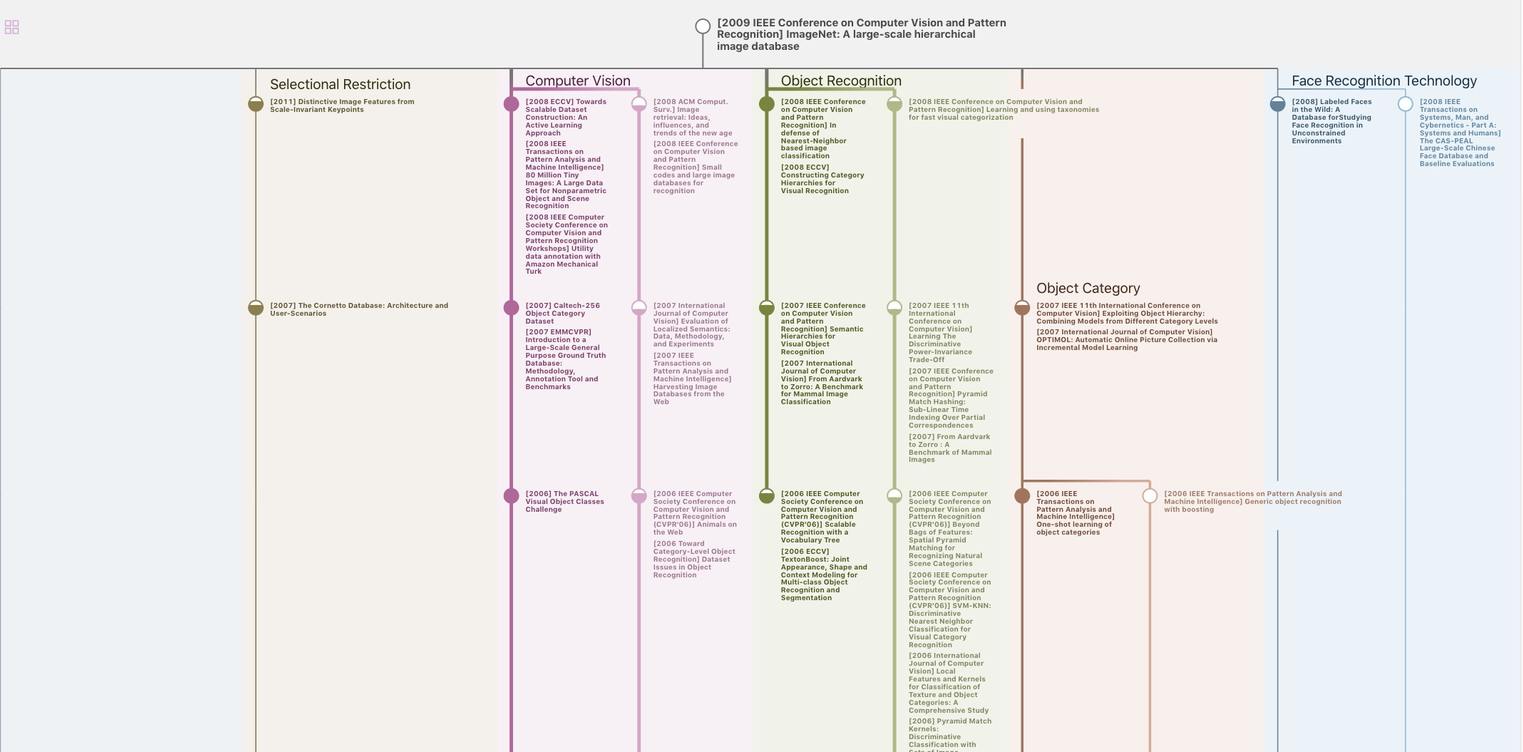
生成溯源树,研究论文发展脉络
Chat Paper
正在生成论文摘要