Interpretable Automatic Fine-grained Inconsistency Detection in Text Summarization
conf_acl(2023)
摘要
Existing factual consistency evaluation approaches for text summarization provide binary predictions and limited insights into the weakness of summarization systems. Therefore, we propose the task of fine-grained inconsistency detection, the goal of which is to predict the fine-grained types of factual errors in a summary. Motivated by how humans inspect factual inconsistency in summaries, we propose an interpretable fine-grained inconsistency detection model, FineGrainFact, which explicitly represents the facts in the documents and summaries with semantic frames extracted by semantic role labeling, and highlights the related semantic frames to predict inconsistency. The highlighted semantic frames help verify predicted error types and correct inconsistent summaries. Experiment results demonstrate that our model outperforms strong baselines and provides evidence to support or refute the summary.
更多查看译文
关键词
summarization,inconsistency detection,text
AI 理解论文
溯源树
样例
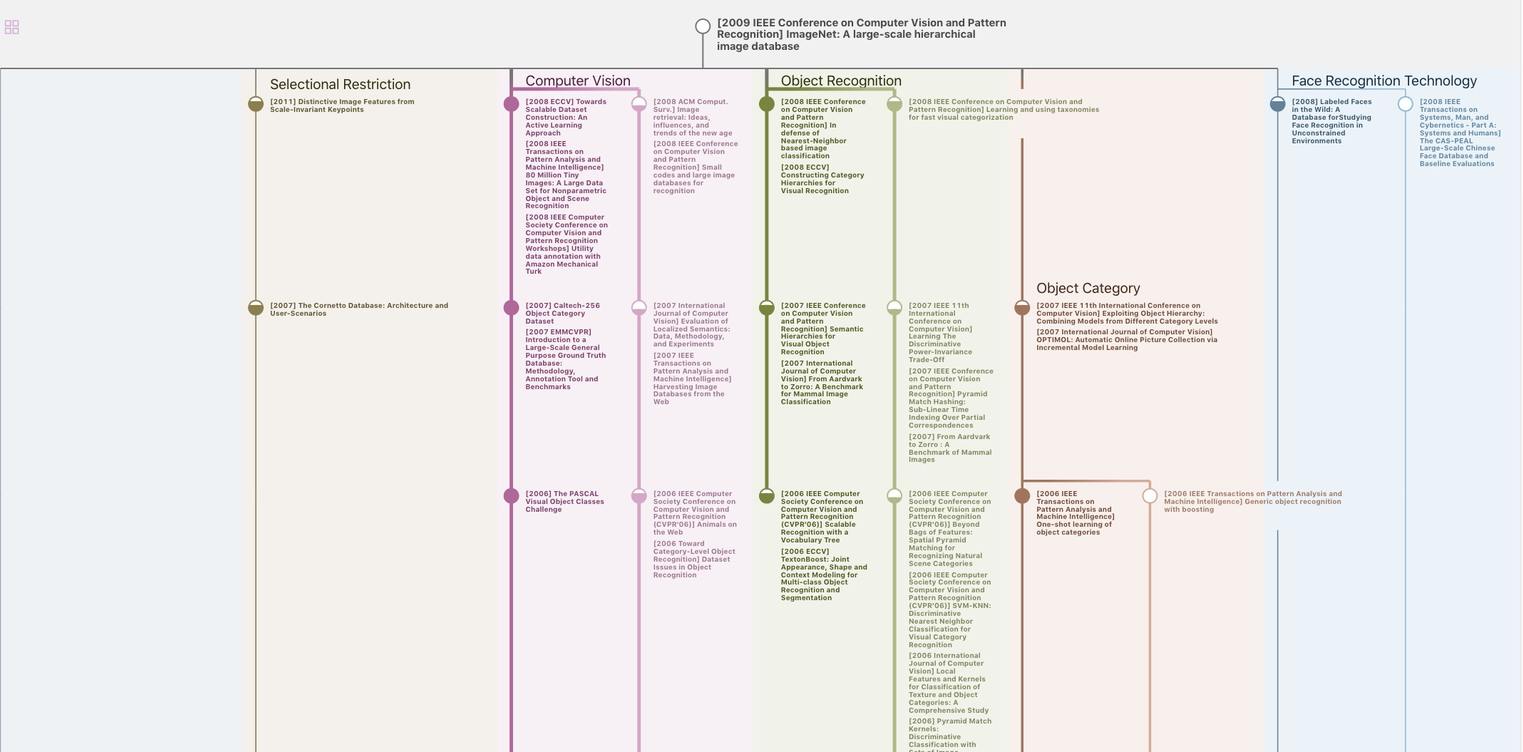
生成溯源树,研究论文发展脉络
Chat Paper
正在生成论文摘要