Practical Cloud-Edge Scheduling for Large-Scale Crowdsourced Live Streaming
IEEE Transactions on Parallel and Distributed Systems(2023)
摘要
Even though conventional wisdom claims that in order to improve viewer engagement, the cloud-edge providers should serve the viewers with the nearest edge nodes, however, we show that doing this for crowdsourced live streaming (CLS) services can introduce significant costs inefficiency. In this paper, we first carry out large-scale measurement analysis by using the real-world service data from Huawei Cloud, a representative cloud-edge provider in China. We observe that the massive number of channels has proposed great burdens to the operating expenditure of the cloud-edge providers, and most importantly, unbalanced viewer distribution makes the edge nodes suffer significant costs inefficiency. To tackle the above concerns, we propose
AggCast
, a novel CLS scheduling framework to optimize the edge node utilization for the cloud-edge provider. The core idea of
AggCast
is to aggregate some viewers that are initially scattered on different regions, and assign them to fewer pre-selected nodes, thereby reducing bandwidth costs. In particular, by integrating the useful insights obtained from our large-scale measurement,
AggCast
can not only ensure that quality of experience (QoS) does not suffer degradation, but also satisfy the systematic requirements of CLS services.
AggCast
has been A/B tested and fully deployed. The online and trace-driven experiments show that, compared to the most prevalent method,
AggCast
saves over 16.3%
back-to-source
(BTS) bandwidth costs while significantly improving QoS (startup latency, stall frequency and stall time are reduced over 12.3%, 4.57% and 3.91%, respectively).
更多查看译文
关键词
Costs, Quality of service, Bandwidth, Servers, Optimization, Computer science, Systematics, Cloud edge computing, content delivery, Index Terms, live streaming, resource scheduling, traffic engineering
AI 理解论文
溯源树
样例
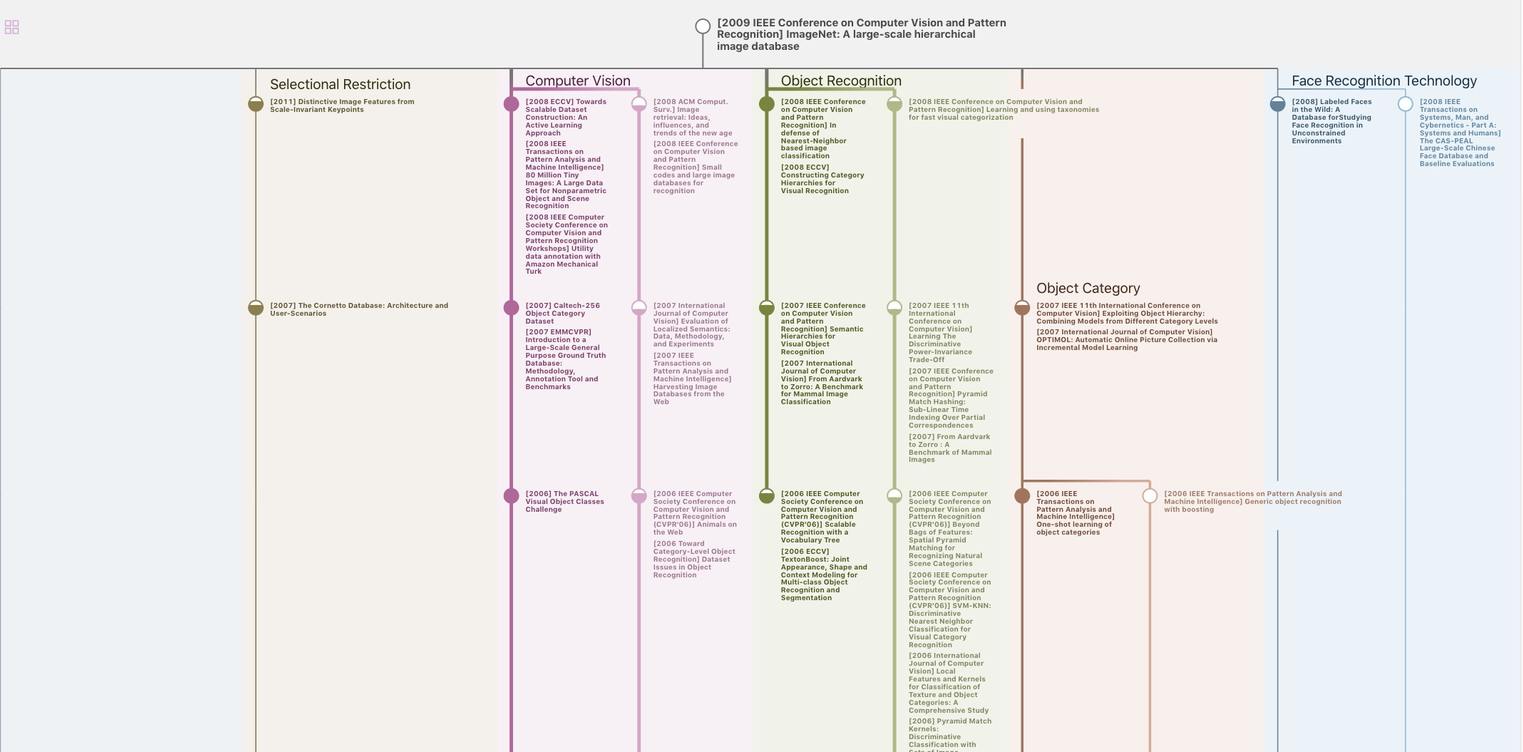
生成溯源树,研究论文发展脉络
Chat Paper
正在生成论文摘要