Theory and rationale of interpretable all-in-one pattern discovery and disentanglement system
npj Digital Medicine(2023)
摘要
In machine learning (ML), association patterns in the data, paths in decision trees, and weights between layers of the neural network are often entangled due to multiple underlying causes, thus masking the pattern-to-source relation, weakening prediction, and defying explanation. This paper presents a revolutionary ML paradigm: pattern discovery and disentanglement (PDD) that disentangles associations and provides an all-in-one knowledge system capable of (a) disentangling patterns to associate with distinct primary sources; (b) discovering rare/imbalanced groups, detecting anomalies and rectifying discrepancies to improve class association, pattern and entity clustering; and (c) organizing knowledge for statistically supported interpretability for causal exploration. Results from case studies have validated such capabilities. The explainable knowledge reveals pattern-source relations on entities, and underlying factors for causal inference, and clinical study and practice; thus, addressing the major concern of interpretability, trust, and reliability when applying ML to healthcare, which is a step towards closing the AI chasm.
更多查看译文
关键词
disentanglement system,pattern discovery,theory,all-in-one
AI 理解论文
溯源树
样例
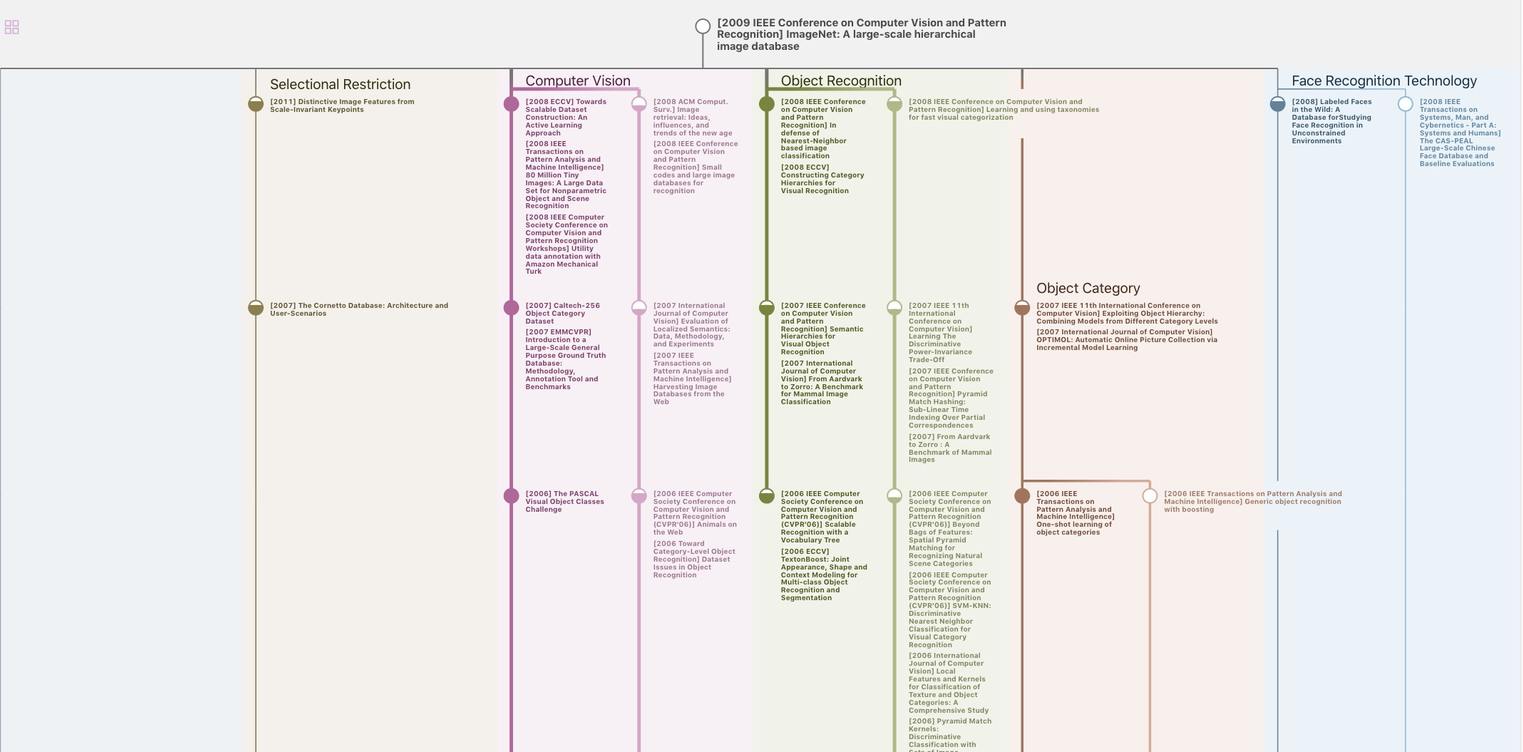
生成溯源树,研究论文发展脉络
Chat Paper
正在生成论文摘要