Conditional Mutual Information for Disentangled Representations in Reinforcement Learning
arXiv (Cornell University)(2023)
摘要
Reinforcement Learning (RL) environments can produce training data with spurious correlations between features due to the amount of training data or its limited feature coverage. This can lead to RL agents encoding these misleading correlations in their latent representation, preventing the agent from generalising if the correlation changes within the environment or when deployed in the real world. Disentangled representations can improve robustness, but existing disentanglement techniques that minimise mutual information between features require independent features, thus they cannot disentangle correlated features. We propose an auxiliary task for RL algorithms that learns a disentangled representation of high-dimensional observations with correlated features by minimising the conditional mutual information between features in the representation. We demonstrate experimentally, using continuous control tasks, that our approach improves generalisation under correlation shifts, as well as improving the training performance of RL algorithms in the presence of correlated features.
更多查看译文
关键词
disentangled representations,information,learning
AI 理解论文
溯源树
样例
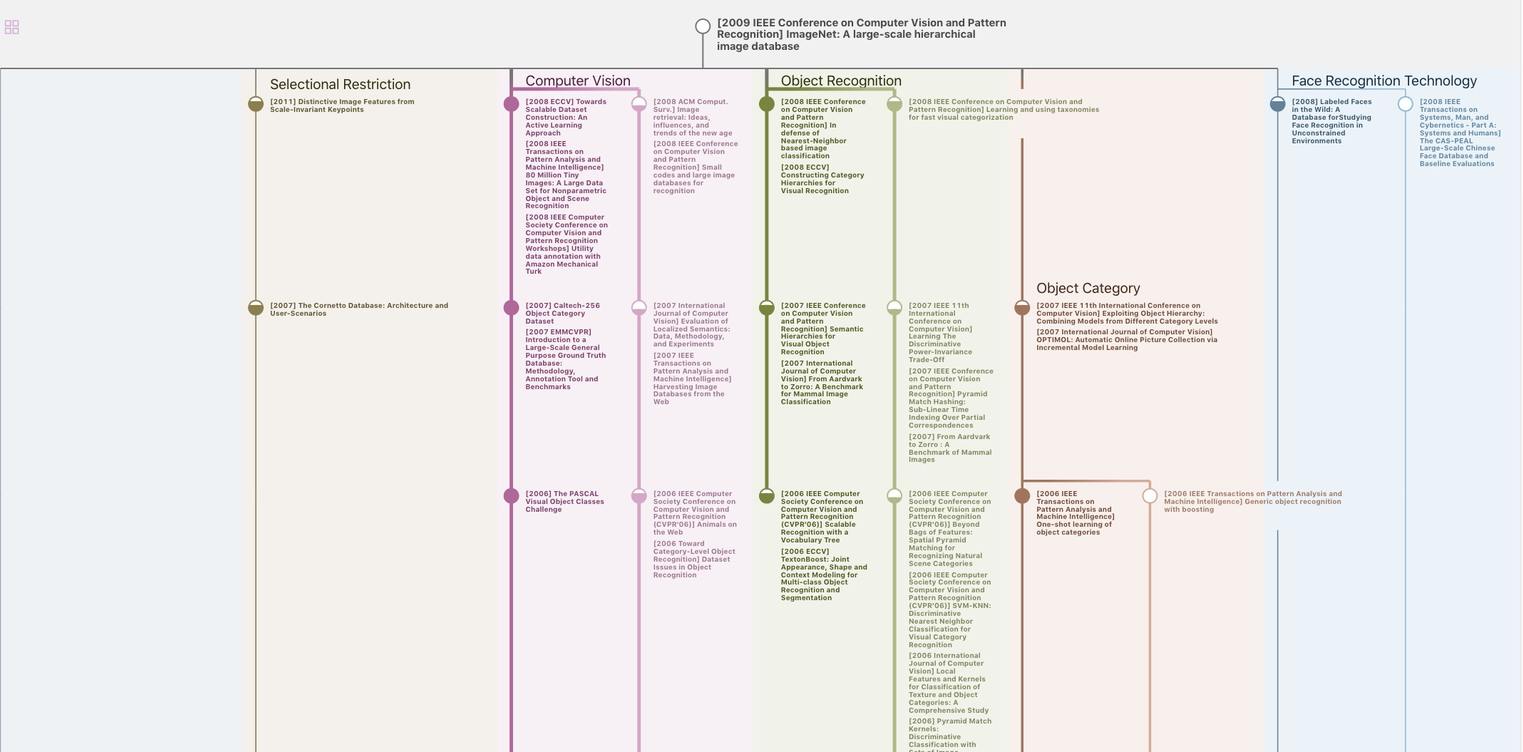
生成溯源树,研究论文发展脉络
Chat Paper
正在生成论文摘要