Deep Learning and Image Super-Resolution-Guided Beam and Power Allocation for mmWave Networks
IEEE TRANSACTIONS ON VEHICULAR TECHNOLOGY(2023)
摘要
In this article, we develop a deep learning (DL)-guided hybrid beam and power allocation approach for multiuser millimeter-wave (mmWave) networks, which facilitates swift beamforming at the base station (BS). The following persisting challenges motivated our research: (i) User and vehicular mobility, as well as redundant beam-reselections in mmWave networks, degrade the efficiency; (ii) Due to the large beamforming dimension at the BS, the beamforming weights predicted by the cutting-edge DL-based methods often do not suit the channel distributions; (iii) Co-located user devices may cause a severe beam conflict, thus deteriorating system performance. To address the aforementioned challenges, we exploit the synergy of supervised learning and super-resolution technology to enable low-overhead beam- and power allocation. In the first step, we propose a method for beam-quality prediction. It is based on deep learning and explores the relationship between high- and low-resolution beam images (energy). Afterward, we develop a DL-based allocation approach, which enables high-accuracy beam and power allocation with only a portion of the available time-sequential low-resolution images. Theoretical and numerical results verify the effectiveness of our proposed framework.
更多查看译文
关键词
Array signal processing,Millimeter wave communication,Resource management,Training,Neural networks,Deep learning,Downlink,Deep learning,power allocation,temporal and spatial resolution,mmWave networks,super-resolution
AI 理解论文
溯源树
样例
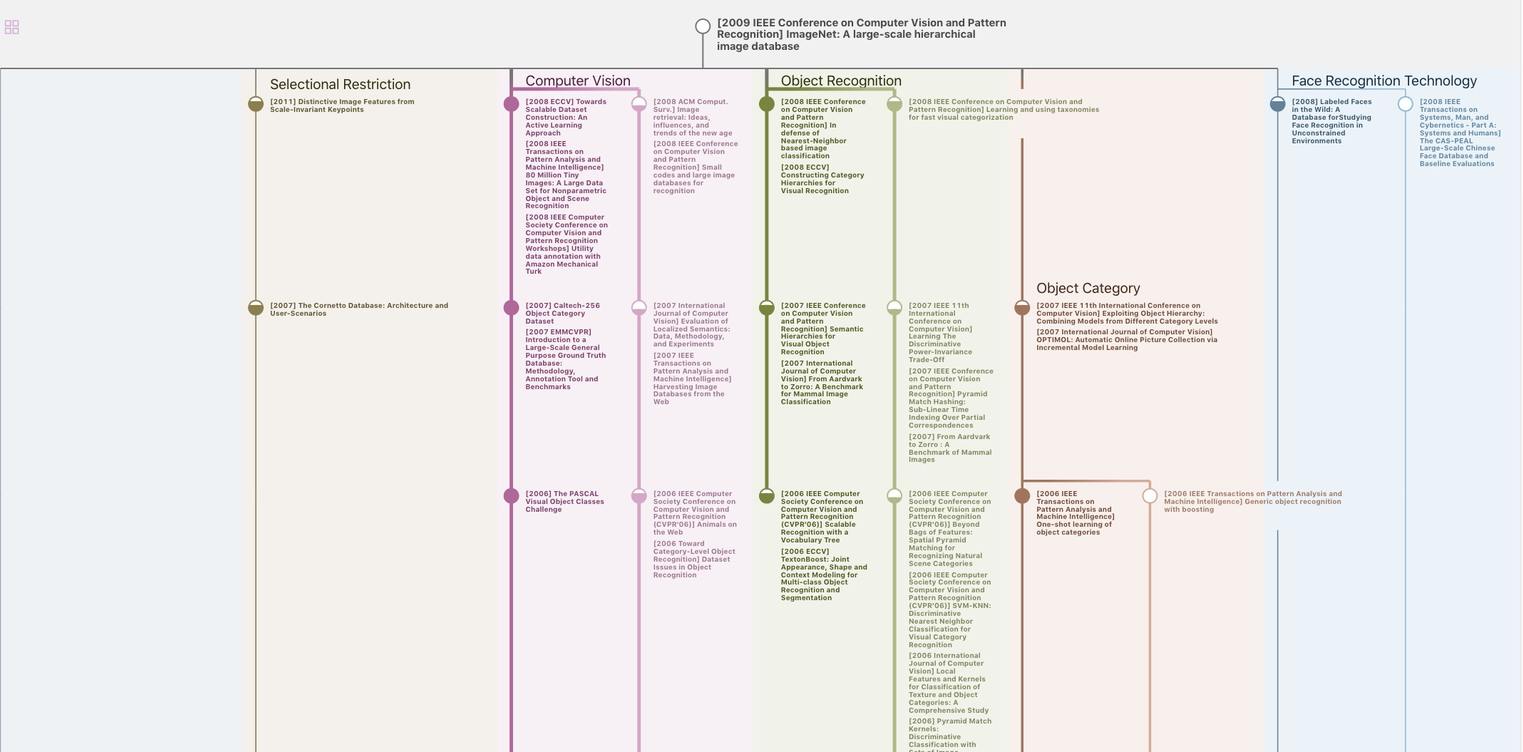
生成溯源树,研究论文发展脉络
Chat Paper
正在生成论文摘要