Control-A-Video: Controllable Text-to-Video Generation with Diffusion Models
CoRR(2023)
摘要
This paper presents a controllable text-to-video (T2V) diffusion model, named Video-ControlNet, that generates videos conditioned on a sequence of control signals, such as edge or depth maps. Video-ControlNet is built on a pre-trained conditional text-to-image (T2I) diffusion model by incorporating a spatial-temporal self-attention mechanism and trainable temporal layers for efficient cross-frame modeling. A first-frame conditioning strategy is proposed to facilitate the model to generate videos transferred from the image domain as well as arbitrary-length videos in an auto-regressive manner. Moreover, Video-ControlNet employs a novel residual-based noise initialization strategy to introduce motion prior from an input video, producing more coherent videos. With the proposed architecture and strategies, Video-ControlNet can achieve resource-efficient convergence and generate superior quality and consistent videos with fine-grained control. Extensive experiments demonstrate its success in various video generative tasks such as video editing and video style transfer, outperforming previous methods in terms of consistency and quality. Project Page: https://controlavideo.github.io/
更多查看译文
关键词
generation,control-a-video,text-to-video
AI 理解论文
溯源树
样例
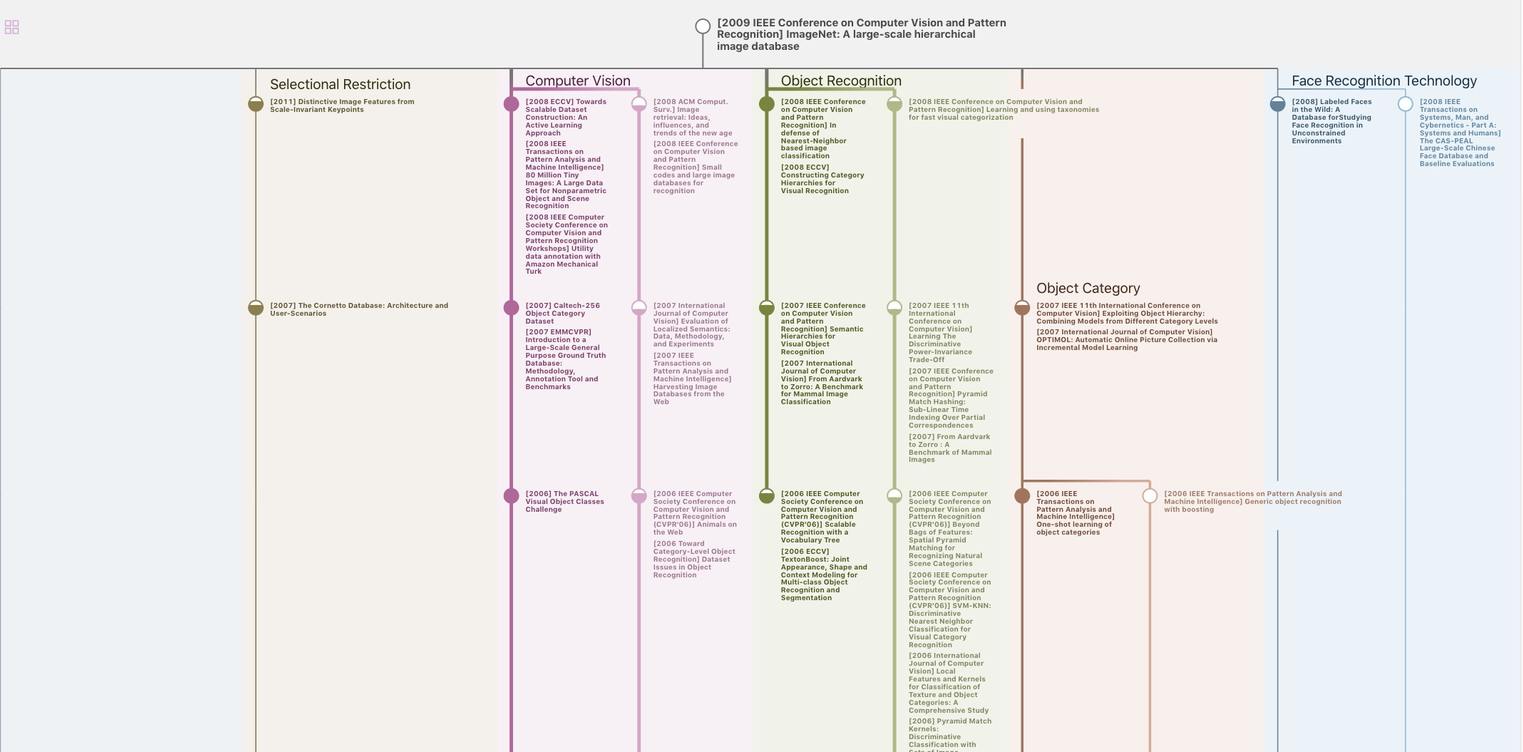
生成溯源树,研究论文发展脉络
Chat Paper
正在生成论文摘要