Federated Primal Dual Fixed Point Algorithm
arXiv (Cornell University)(2023)
摘要
Federated learning (FL) is a distributed learning paradigm that allows several clients to learn a global model without sharing their private data. In this paper, we generalize a primal dual fixed point (PDFP) \cite{PDFP} method to federated learning setting and propose an algorithm called Federated PDFP (FPDFP) for solving composite optimization problems. In addition, a quantization scheme is applied to reduce the communication overhead during the learning process. An $O(\frac{1}{k})$ convergence rate (where $k$ is the communication round) of the proposed FPDFP is provided. Numerical experiments, including graph-guided logistic regression, 3D Computed Tomography (CT) reconstruction are considered to evaluate the proposed algorithm.
更多查看译文
关键词
algorithm
AI 理解论文
溯源树
样例
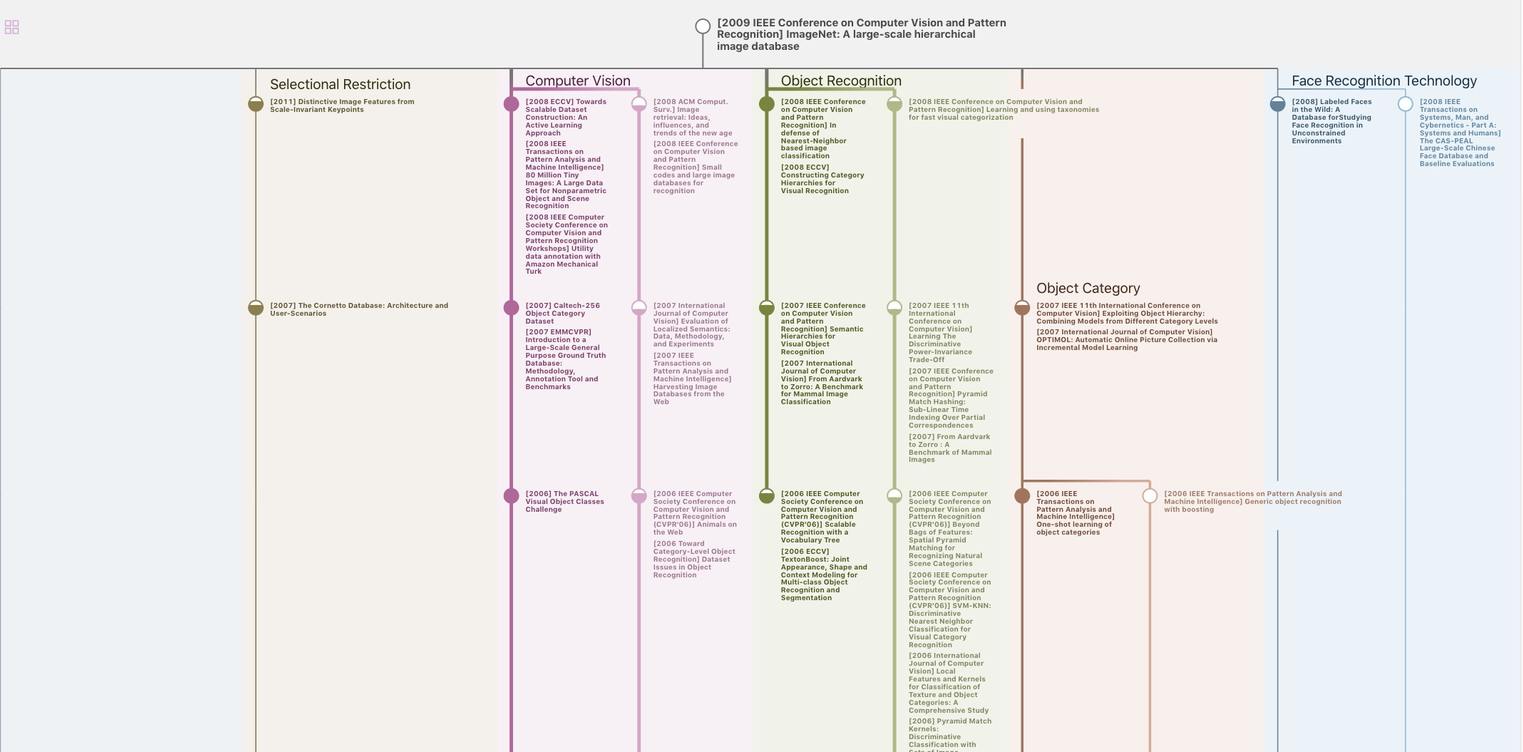
生成溯源树,研究论文发展脉络
Chat Paper
正在生成论文摘要