SiCL: Silhouette-Driven Contrastive Learning for Unsupervised Person Re-Identification with Clothes Change
arxiv(2023)
摘要
In this paper, we address a highly challenging yet critical task:
unsupervised long-term person re-identification with clothes change. Existing
unsupervised person re-id methods are mainly designed for short-term scenarios
and usually rely on RGB cues so that fail to perceive feature patterns that are
independent of the clothes. To crack this bottleneck, we propose a
silhouette-driven contrastive learning (SiCL) method, which is designed to
learn cross-clothes invariance by integrating both the RGB cues and the
silhouette information within a contrastive learning framework. To our
knowledge, this is the first tailor-made framework for unsupervised long-term
clothes change , with superior performance on six benchmark datasets. We
conduct extensive experiments to evaluate our proposed SiCL compared to the
state-of-the-art unsupervised person reid methods across all the representative
datasets. Experimental results demonstrate that our proposed SiCL significantly
outperforms other unsupervised re-id methods.
更多查看译文
AI 理解论文
溯源树
样例
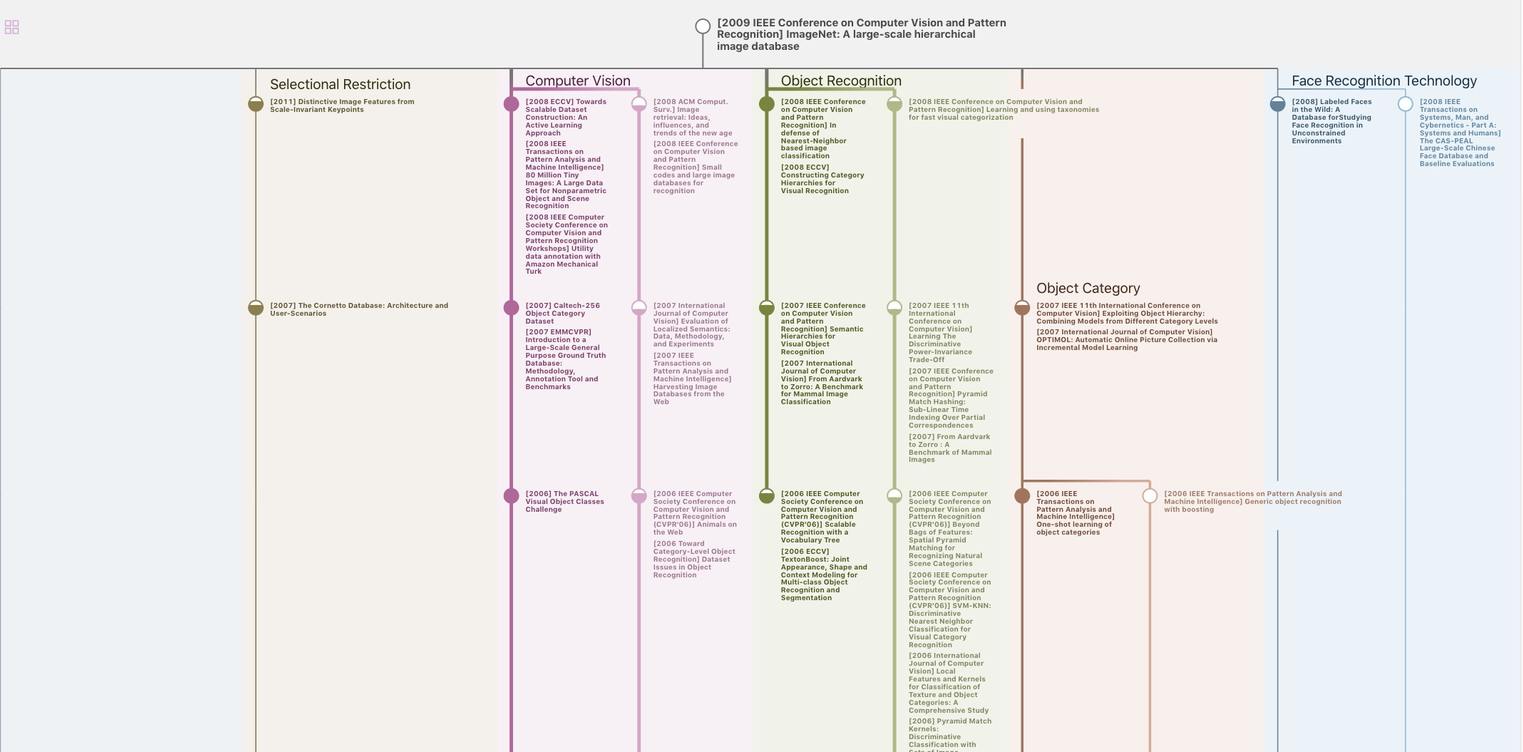
生成溯源树,研究论文发展脉络
Chat Paper
正在生成论文摘要