Rethinking Machine Learning Collective Communication as a Multi-Commodity Flow Problem
CoRR(2023)
摘要
We show communication schedulers' recent work proposed for ML collectives does not scale to the increasing problem sizes that arise from training larger models. These works also often produce suboptimal schedules. We make a connection with similar problems in traffic engineering and propose a new method, TECCL, that finds better quality schedules (e.g., finishes collectives faster and/or while sending fewer bytes) and does so more quickly on larger topologies. We present results on many different GPU topologies that show substantial improvement over the state-of-the-art.
更多查看译文
关键词
machine learning collective communication,flow,machine learning,multi-commodity
AI 理解论文
溯源树
样例
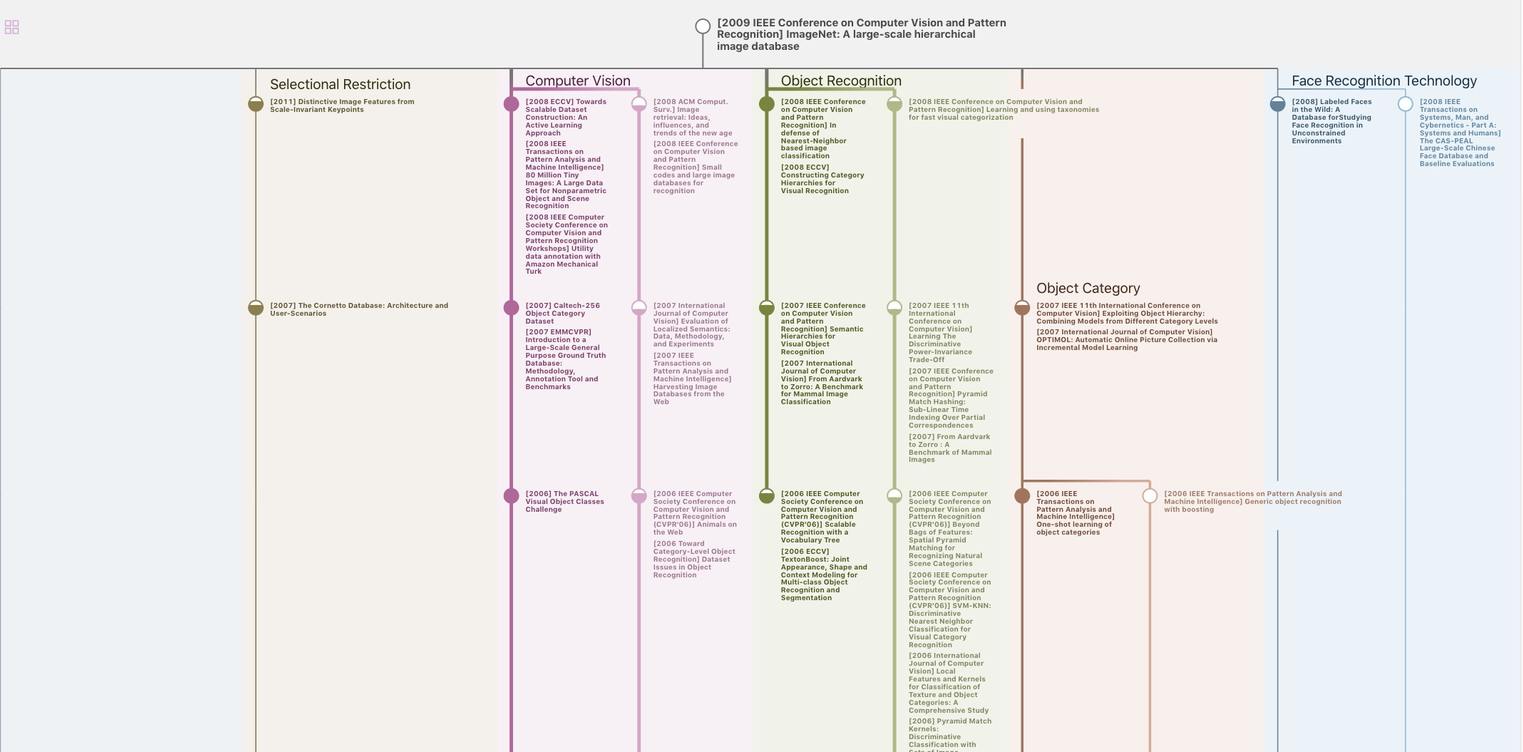
生成溯源树,研究论文发展脉络
Chat Paper
正在生成论文摘要