Correcting for Granularity Bias in Modularity-Based Community Detection Methods
Algorithms and Models for the Web Graph(2023)
摘要
Maximizing modularity is currently the most widely-used community detection method in applications. Modularity comes with a parameter that indirectly controls the granularity of the resulting clustering. Moreover, one can choose this parameter in such a way that modularity maximization becomes equivalent to maximizing the likelihood of a stochastic block model. Thus, this method is statistically justified, while at the same time, it is known to have a bias towards fine-grained clusterings. In this work, we introduce a heuristic to correct for this bias. This heuristic is based on prior work where modularity is described in geometric terms. This has led to a broad generalization of modularity-based community detection methods, and the heuristic presented in this paper applies to each of them. We justify the heuristic by describing a relation between several distances that we observe to hold in many instances. We prove that, assuming the validity of this relation, our heuristic leads to a clustering of the same granularity as the ground-truth clustering. We compare our heuristic to likelihood-based community detection methods on several synthetic graphs and show that our method indeed results in clusterings with granularity closer to the granularity of the ground-truth clustering. Moreover, our heuristic often outperforms likelihood maximization in terms of similarity to the ground-truth clustering.
更多查看译文
关键词
community detection methods,granularity bias,modularity-based
AI 理解论文
溯源树
样例
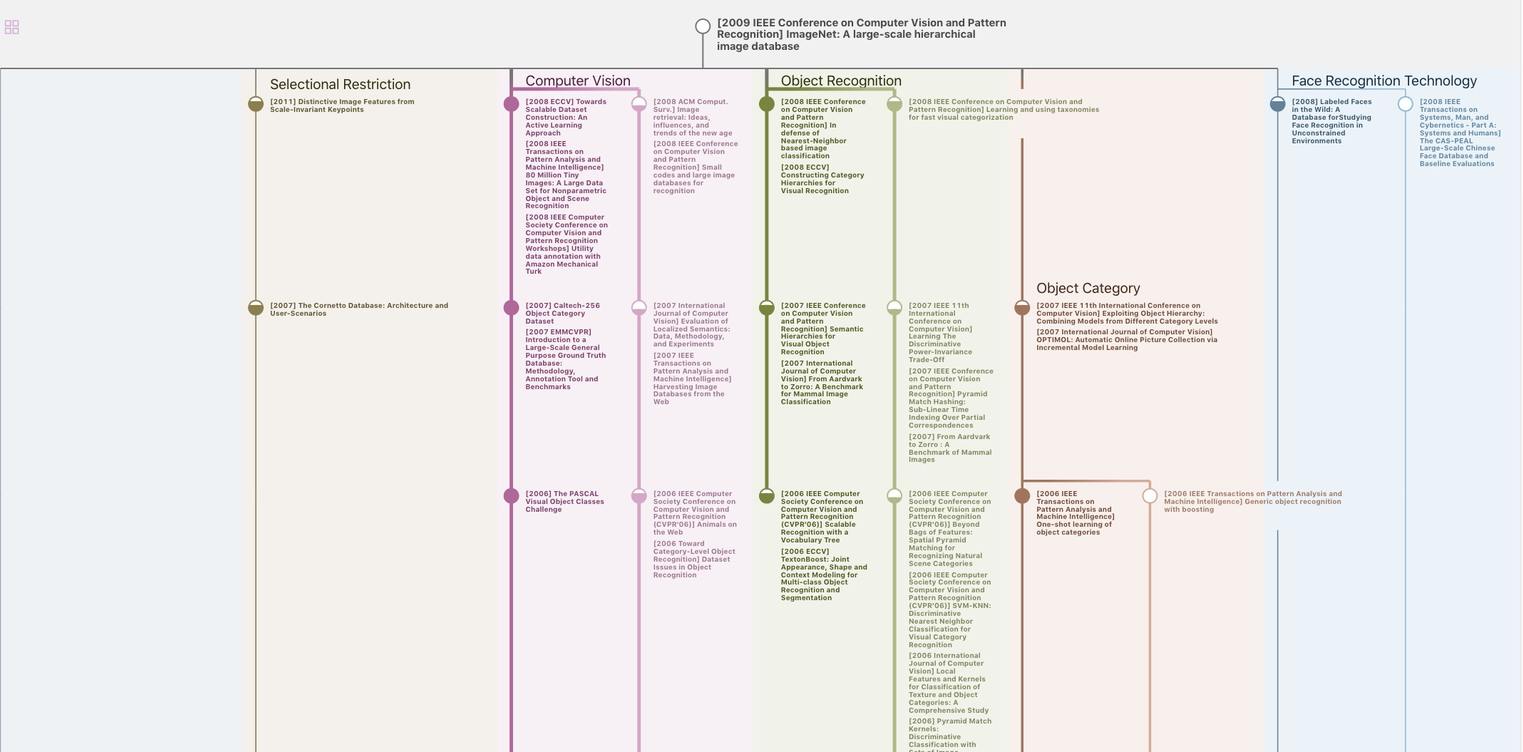
生成溯源树,研究论文发展脉络
Chat Paper
正在生成论文摘要