A LSTM Deep Learning Approach for Forecasting Global Air Quality Index
Third Congress on Intelligent Systems(2023)
摘要
The air quality index (AQI) provides information on air quality and its impact on human health using a single number. The AQI is derived from the measured concentration of air pollutants over a period. In this work, we present an approach based on deep learning using the long short-term memory (LSTM) neural network architecture, with the ability to predict an AQI for the next few hours. The predictive model learns to infer an air quality index at the hourly level and in an average range of 8 h, a requirement in the environmental regulations for AQI notification. Our experiment uses real data on pollutant concentration levels and meteorological factors collected at four air quality monitoring stations. The performance of the global AQI forecasting made by the LSTM model is measured considering mean absolute error (MAE), root-mean-squared error (RMSE), and mean absolute percentage error (MAPE) parameters reaching very acceptable results in most cases.
更多查看译文
关键词
Forecasting, Deep learning, AQI, LSTM, Pollution
AI 理解论文
溯源树
样例
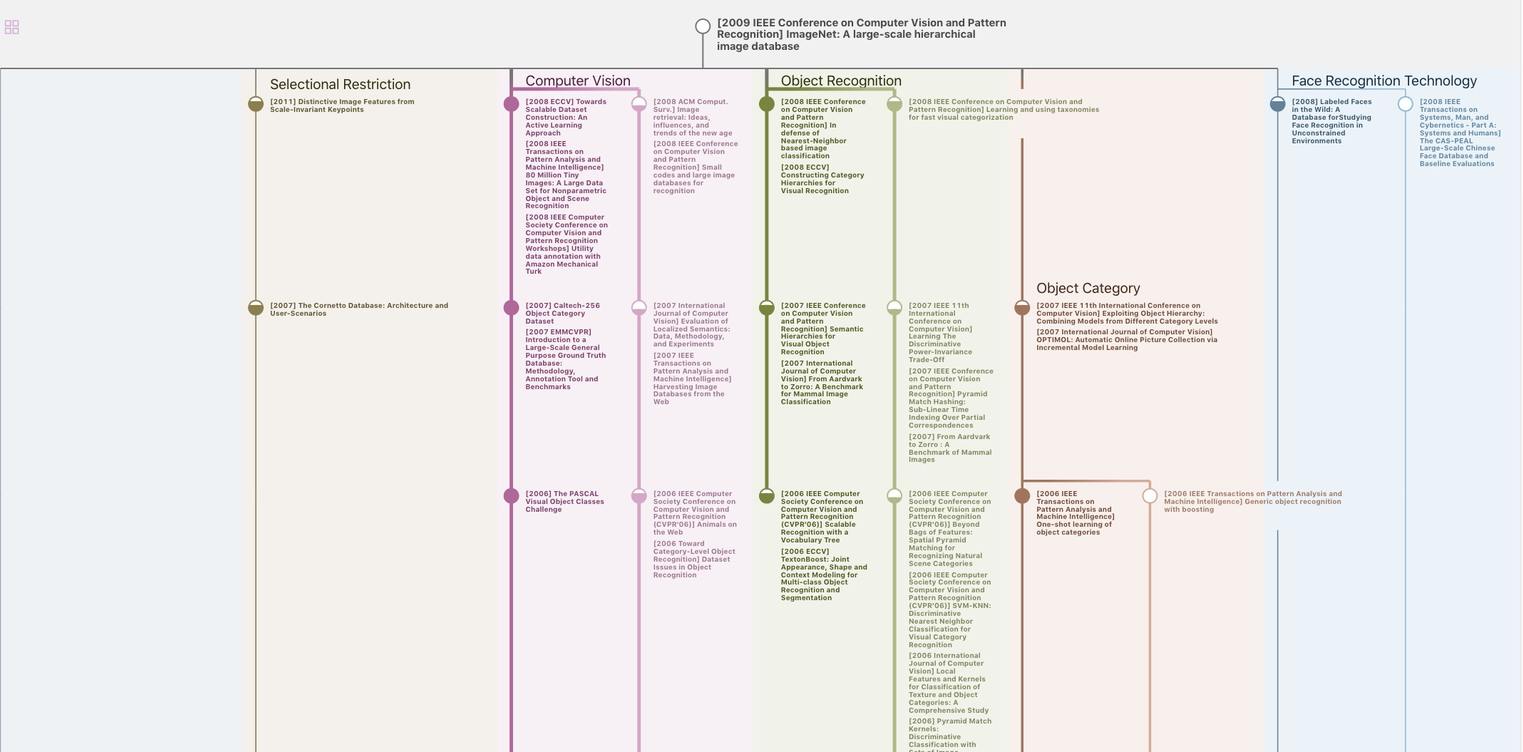
生成溯源树,研究论文发展脉络
Chat Paper
正在生成论文摘要