U-SSLN: A U-Net based and optimization preferred semi-supervised learning network for medical image segmentation
2022 4th International Conference on Machine Learning, Big Data and Business Intelligence (MLBDBI)(2022)
摘要
In the medical field, image analysis is an important and complex task for clinical diagnosis. While image analysis can provide an important diagnostic basis for clinical treatment, image analysis also needs to determine its different analysis purposes (i.e., purpose preference for image analysis) according to different treatment requirements. Such purpose-preference image analysis will help improve the diagnostic capability of clinical care. In recent years, with the rapid development of deep learning for semantic segmentation, this technique has become an important auxiliary aid for medical image analysis. However, the expensive manual labeling cost makes the training data for semantic segmentation insufficient. This may lead to models that exhibit poor segmentation ability, while increasing the likelihood of models providing misleading information. To solve the problem of insufficient training data, many improvement methods have been proposed. For example, transfer learning, unsupervised learning, and semi-supervised learning. However, some of the methods have been poorly optimized on medical images. The semantic segmentation network UA-MT provides a semi-supervised approach based on the V-Net architecture to alleviate the data shortage problem, but it rarely integrates the segmentation performance with the purpose preference of image analysis. In this paper, we propose a semi-supervised learning network (U-SSLN) based on the U-Net architecture, which can be integrated with the purposeful preference of image analysis by different optimization combination strategies. U-SSLN integrates the advantages of UA-MT and U-Net architecture, and its combination strategies are mainly through residual concatenation (RC) on the segmentation model and batch normalization (BN) on the up-down sampling layer to implementation. These combined strategies can further optimize the segmentation performance of the segmentation network on different metrics, allowing the segmentation network to better assist in different stages of medical analysis. In order to verify the feasibility and effectiveness of U-SSLN, we conducted a large number of comparative experiments. The results of the experiments show that the segmentation models based on USSLN outperform the supervised segmentation models in all of them. Moreover, the U-SSLN without RC and BN achieves optimal performance (87.91% and 78.71%) on the area metrics Dice and Jaccard. And on the geometric metrics HD95 and Asd, the U-SSLN with RC and BN reached the optimal performance (10.27 and 1.99).
更多查看译文
关键词
image analysis,purpose preference,semantic segmentation,semi-supervised learning,U-Net architecture,residual connectivity,batch normalization
AI 理解论文
溯源树
样例
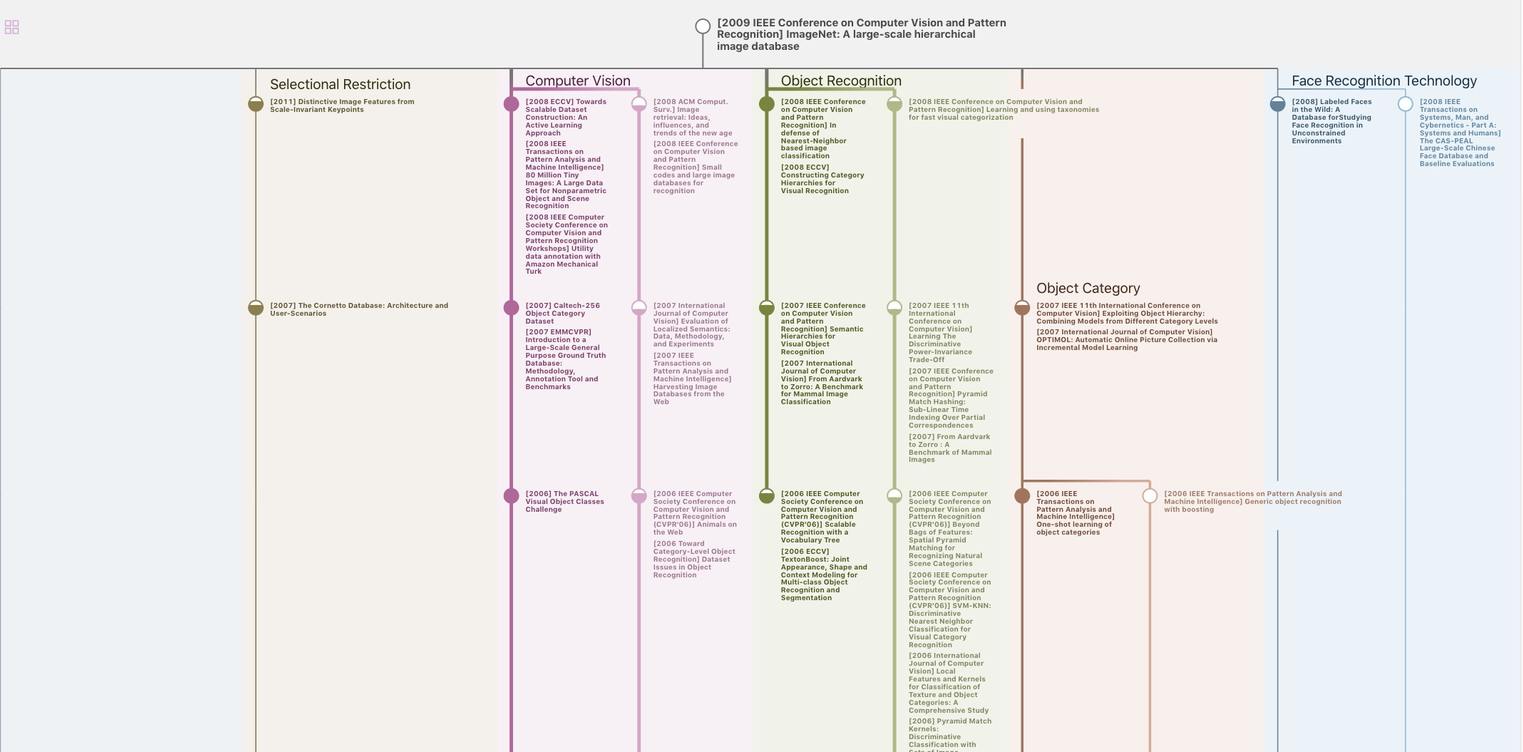
生成溯源树,研究论文发展脉络
Chat Paper
正在生成论文摘要