A Novel Ensemble Classifier Framework for Accurate Fetal Heart Rate Classification
2023 4th International Conference on Signal Processing and Communication (ICSPC)(2023)
摘要
This paper presents a study on the prediction of fetal state, which is crucial for preventing fetal mortality. CTG is a technique widely used for monitoring the fetal heart rate and uterine contractions. In our paper, we implemented a novel ensemble classifier that combines the XGBoost and the Random Forest Classifiers to classify the fetal heart rate in CTG data. This study utilized a publicly available dataset with 2126 instances of fetal heart rate and uterine contractions. The results indicate that the ensemble classifier outperforms the individual XGBoost and Random Forest Classifier in terms of precision and F1 score. It also maintains a high level of accuracy of 96% across all categories, indicating that it effectively strikes a balance between correctly identifying true positives while avoiding false positives and negatives. We also compared the results with other classifiers combined with Adaboost and Gradient Boosting techniques and found that our method is more effective than other classifiers. This consistent performance demonstrates the classifier’s reliability.
更多查看译文
关键词
Cardiotocography,fetal heart rate,XGBoost,Random Forest,Ensemble classifier
AI 理解论文
溯源树
样例
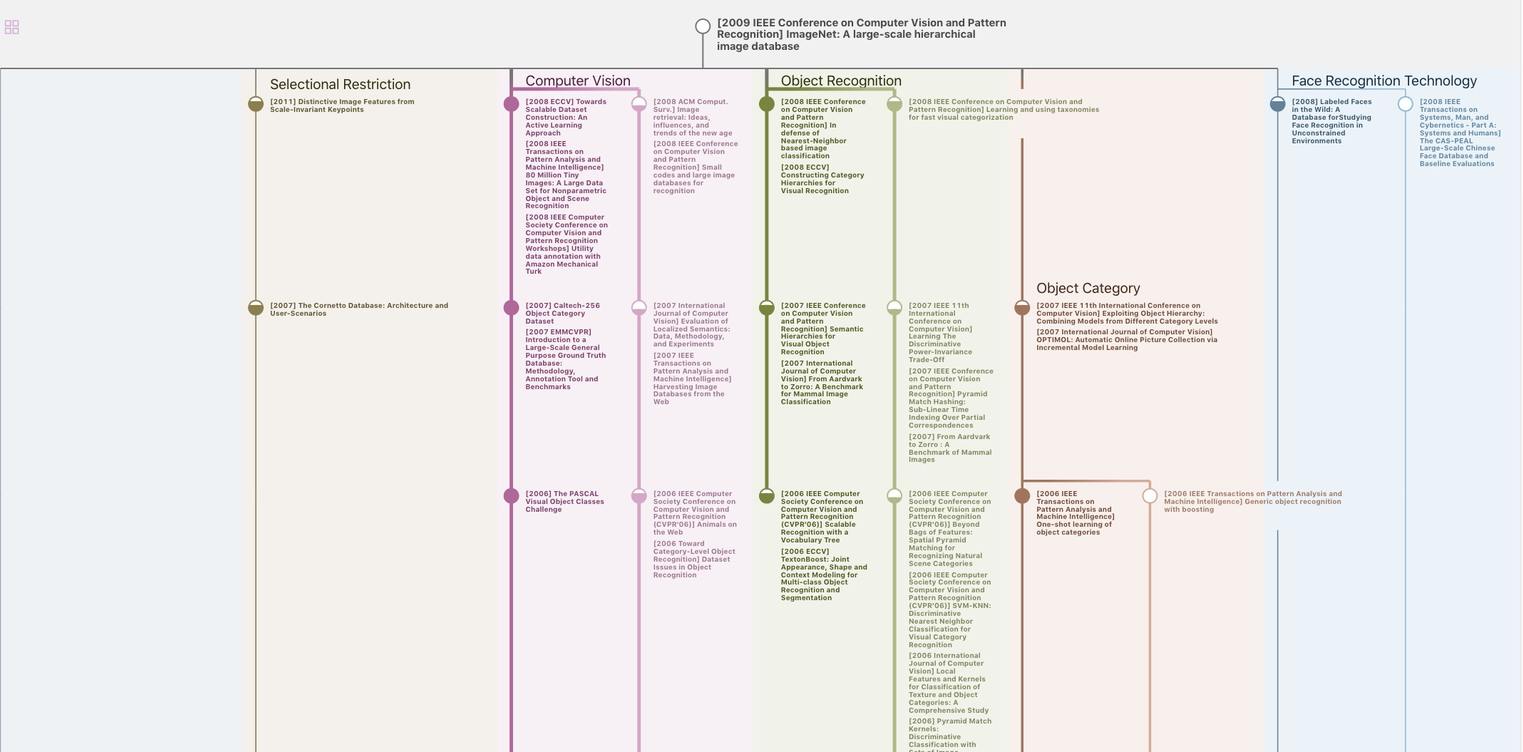
生成溯源树,研究论文发展脉络
Chat Paper
正在生成论文摘要