360$^{\circ}$ Image Saliency Prediction by Embedding Self-Supervised Proxy Task
IEEE Transactions on Broadcasting(2023)
摘要
The development of Metaverse industry produces many 360 $^{\circ}$ images and videos. Transmitting these images or videos efficiently is the key to success of Metaverse. Since the subject’s field of view is limited in Metaverse, from the perception perspective, bit rates can be saved by focusing video encoding on salient regions. On different ways of handling 360 $^{\circ}$ image projections, the existing works either consider combining local and global projections or just use only global projection for saliency prediction, which results in slow detection speed or low accuracy. In this work, we address this problem by Embedding a self-supervised Proxy task in the Saliency prediction Network, dubbed as EPSNet . The main architecture follows an autoencoder with an encoder for feature extraction and a decoder for saliency prediction. The proxy task is combined with the encoder to enforce it to learn local and global information. It is designed to find the location of a certain local projection in the global projection via self-supervised learning. A cross-attention fusion mechanism is used to fuse the global and local features for location prediction. Then, the decoder is trained based on the sole global projection. In this way, the time-consuming local-global feature fusion is placed in the training stage only. Experiments on public dataset show that our method has achieved satisfactory results in terms of inference speed and accuracy. The dataset and code are available at https://github.com/zzz0326/EPSNet.
更多查看译文
关键词
image saliency prediction,self-supervised
AI 理解论文
溯源树
样例
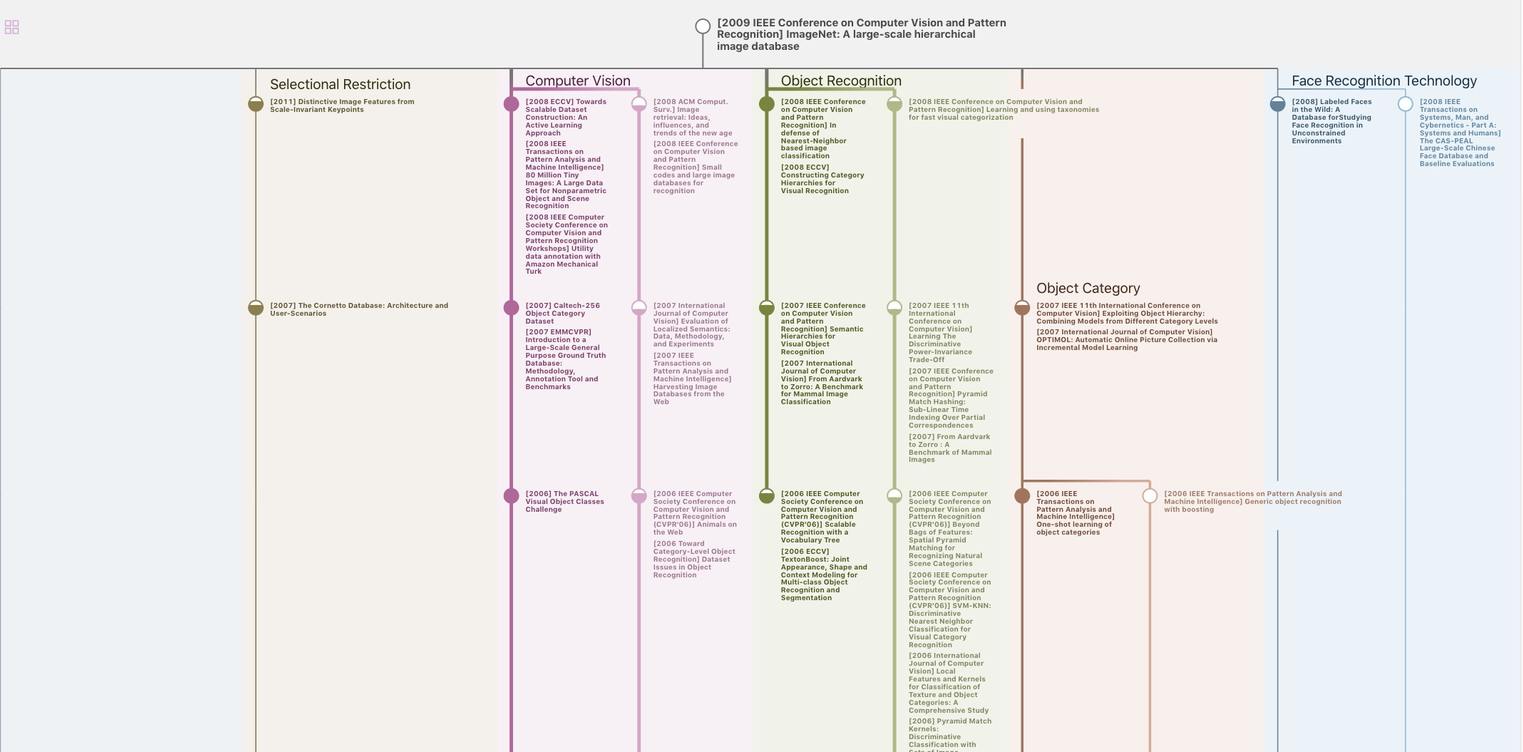
生成溯源树,研究论文发展脉络
Chat Paper
正在生成论文摘要