Source-Load Scenario Generation Based on Weakly Supervised Adversarial Learning and Its Data-Driven Application in Energy Storage Capacity Sizing
IEEE Transactions on Sustainable Energy(2023)
摘要
The historical measured data of renewable energy sources and loads can be processed in various ways to generate scenarios for energy storage planning. With the development of advanced forecast technology, the valuable reference of massive forecast data accumulated by the prediction platform in scenario generation is ignored. To this end, we propose a new paradigm based on scenario generation for energy storage planning considering source-load uncertainties. First, a novel generative adversarial network (GAN) is constructed under weakly supervised learning. The network can extract the prediction errors between predicted data and measured data to generate source-load profiles. The loss function of the network is redesigned to adapt to the data generation task. To match the planning process, scenario generation and reduction algorithms are embedded in the GAN. Then, an energy storage sizing model considering battery health constraints is established. Case studies show that the proposed method can accurately portray the local fluctuation characteristics of source-load power and properly reduce conservatism compared with the model-driven method.
更多查看译文
关键词
weakly supervised adversarial learning,energy storage capacity sizing,source-load,data-driven
AI 理解论文
溯源树
样例
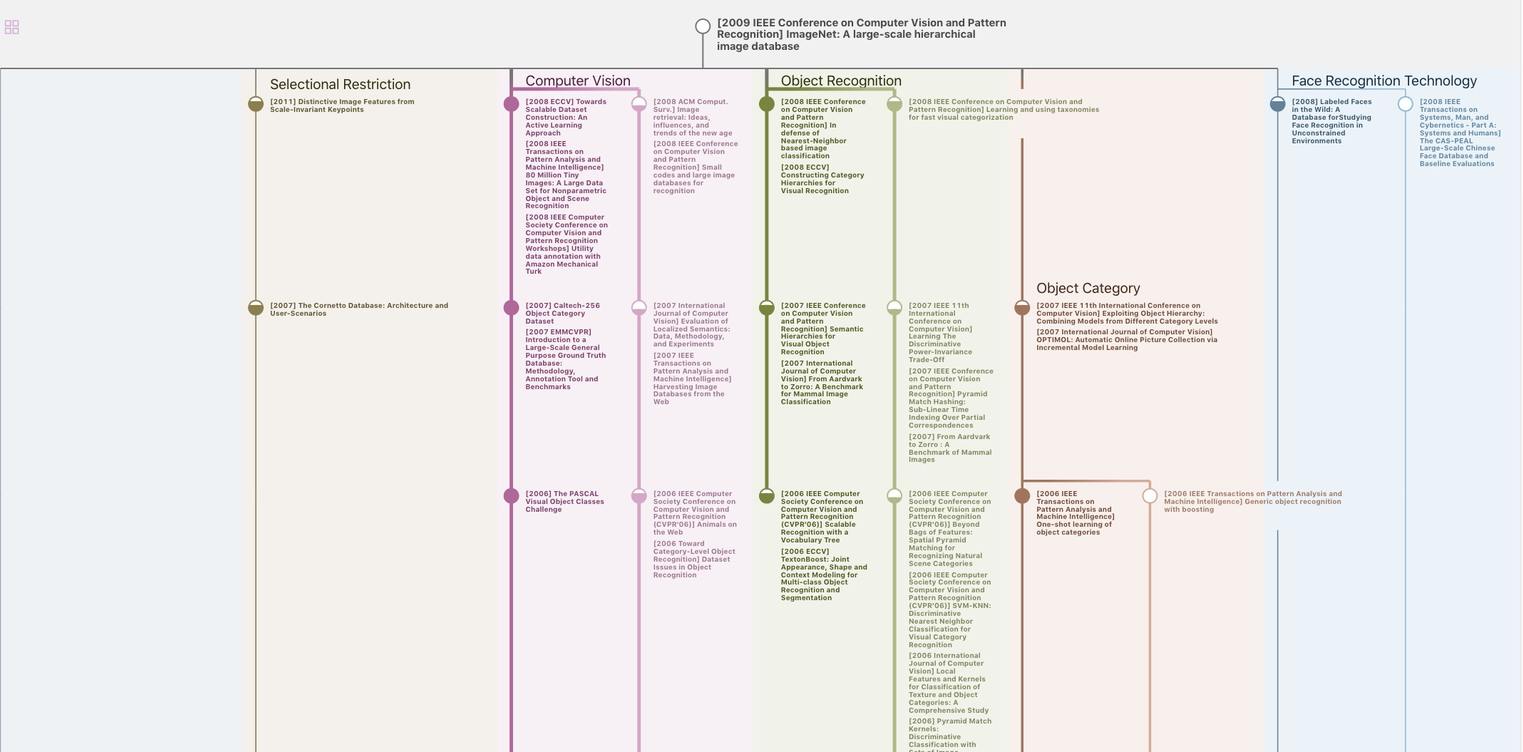
生成溯源树,研究论文发展脉络
Chat Paper
正在生成论文摘要