Monitoring industrial control systems via spatio-temporal graph neural networks
Engineering Applications of Artificial Intelligence(2023)
摘要
Massive amounts of industrial data, which are often gathered by industrial control systems (ICS), have been generated by the fast growth of industrial intelligence. One of the hottest topics in ICS is how to extract the most useful information from industrial ”Big Data” and provide a more comprehensive service for monitoring the condition of industrial production processes. As the industrial environment gets increasingly complicated, production tasks change often and malicious attacks are on the rise. It remains a grand challenge to perform fine-grained anomaly detection in high-dimensional, noisy industrial data. In response to this problem, we propose a spatio-temporal graph neural network-based anomaly detection framework for fine-grained state monitoring of ICSs. First, based on prior knowledge, we propose a method for feature dimensionality reduction and dynamic graph modeling. After that, the variational mode decomposition (VMD) module is then utilized to remove noise from industrial data. Finally, we propose a spatio-temporal feature extraction module for fine-grained anomaly detection. Numerical experiments are conducted on a real-world ICS dataset called HAI. The results demonstrate that the proposed framework can effectively deal with high-dimensional, high-noise, and imbalanced industrial data. The framework’s concepts are interconnected and extensible to various industrial scenarios, including metallurgy, smart shop floors, etc. In terms of recall, precision, and F1-Score, a comparison between the proposed framework and eight representative methods reveals the merits of the proposed framework.
更多查看译文
关键词
Anomaly detection, Industry 4.0, Industrial control systems, Discrete time dynamic graph
AI 理解论文
溯源树
样例
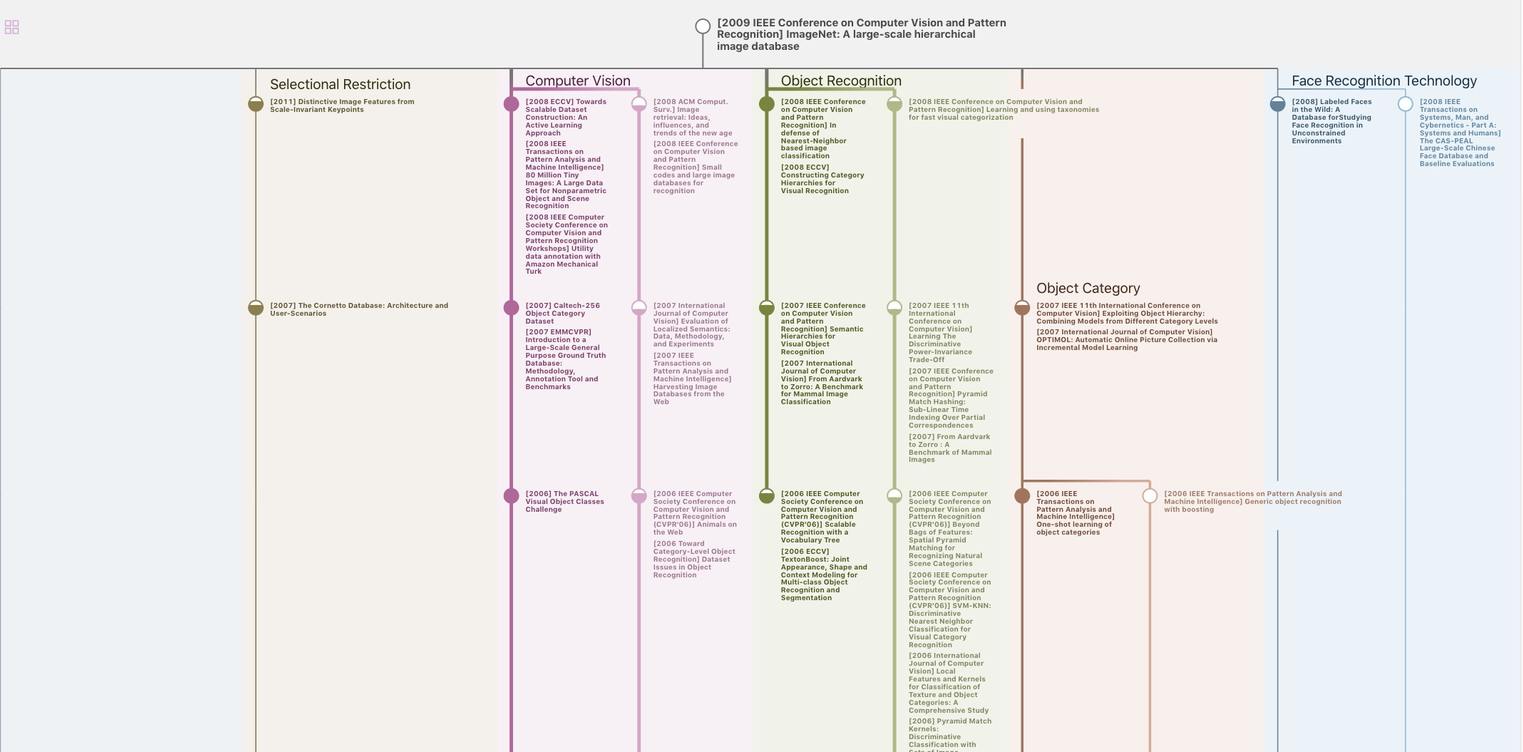
生成溯源树,研究论文发展脉络
Chat Paper
正在生成论文摘要