Uncertainty-Aware Adaptive Semidirect LiDAR Scan Matching for Ground Vehicle Positioning
IEEE Sensors Journal(2023)
摘要
Iterative closest point (ICP) algorithms are widely used in the literature for the estimation of relative transformations using 3-D light detection and ranging (LiDAR) point clouds. This class of algorithms proves to be efficient when the 3-D data share sufficient overlapping parts and a good initial guess is provided. However, large relative motions and mutual occlusion of objects in real-road scenarios hinder traditional optimization-based ICP from achieving optimal estimation. This article explores both direct and feature-based 3-D LiDAR scan matching using the ICP framework in different contexts, such as parking, residential, urban, and highway scenarios. In order to guarantee the scan matching performances in scenarios with scarce geometric information and fast ego-vehicle motion, we propose an adaptive semidirect scan matching (SD-SM) method together with an alignment uncertainty quantification. The proposed SD-SM is tested on both the public KITTI and self-recorded LS2N datasets, which accomplishes the robust six-degree-of-freedom (DOF) pose estimation and consistent scene reconstruction. We demonstrate that the proposed approach outperforms the state of the art and achieves the leading results with 68.3% average relative fitness and 5.71-cm average root-mean-square error (RMSE).
更多查看译文
关键词
uncertainty-aware
AI 理解论文
溯源树
样例
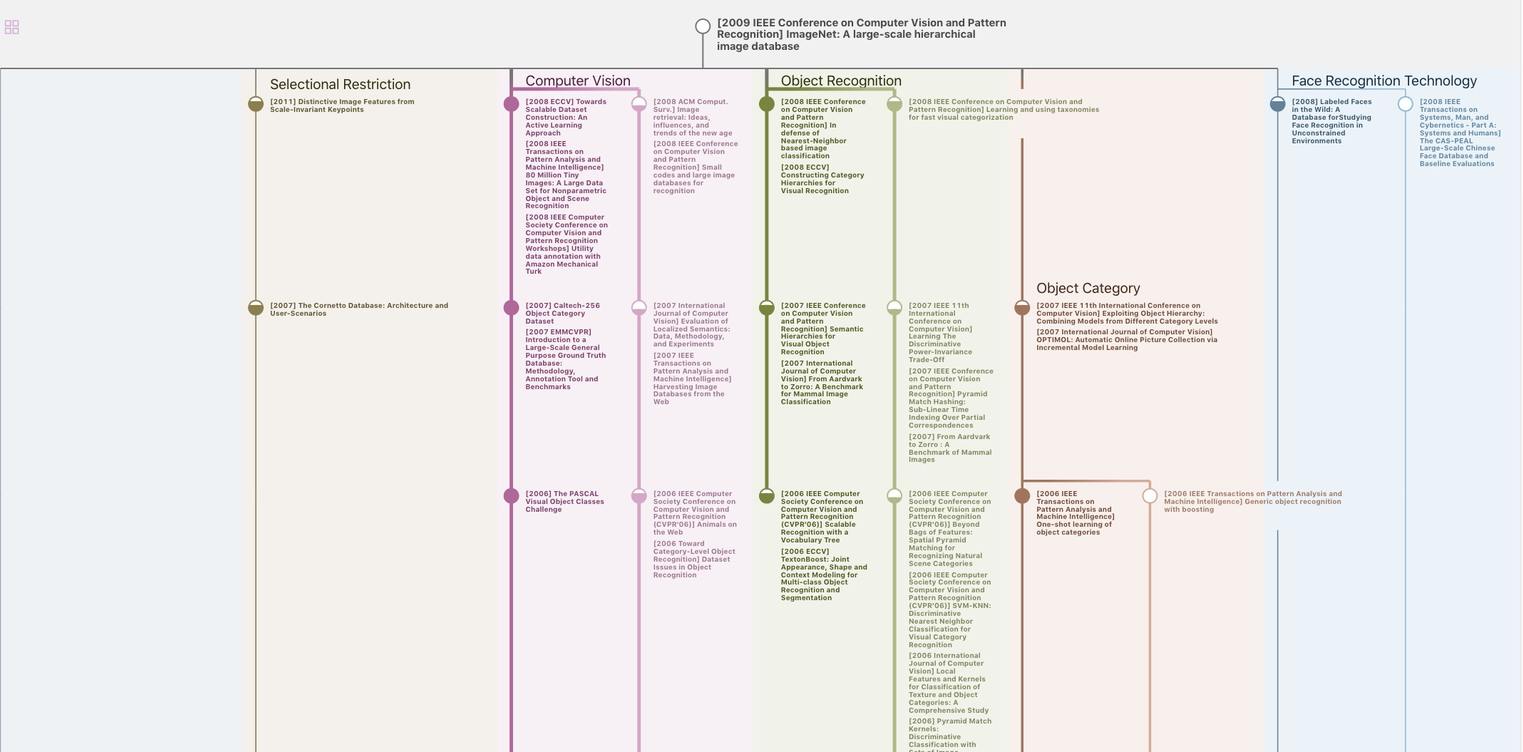
生成溯源树,研究论文发展脉络
Chat Paper
正在生成论文摘要