Using machine learning technology to enhance digital social understanding for the COVID-19 infodemic: Development and implementation of the Early AI-Supported Response with Social Listening (EARS) platform (Preprint)
crossref(2023)
摘要
BACKGROUND Amidst the COVID-19 pandemic, there has been a need for rapid social understanding to inform infodemic management and response. While social media analysis platforms have traditionally been designed for commercial brands for marketing and sales purposes, it has been under-utilized and under-adapted for comprehensive understanding of social dynamics in areas such as public health. Traditional systems have challenges for public health usage and new tools and innovative methods are needed. The WHO Early AI-Supported Response with Social Listening (EARS) platform was developed to overcome some of these challenges. OBJECTIVE To describe the methodological approach to the development of the EARS platform, including data sourcing, development and validation of a machine learning categorization approach, as well as results from the pilot. METHODS Data for EARS is collected daily from online conversations in publicly available sources, including Twitter, Facebook public pages, online forums, news comments, and blogs in 9 languages. Public health and social media experts developed a taxonomy to categorize COVID-19 narratives to 5 relevant main categories and 41 sub-categories. We developed a semi-supervised machine learning algorithm to categorize social media posts to categories and various filters. To validate the results obtained by the machine learning-based approach we compared it to a search filters methodology applying boolean queries with the same amount of information and measured recall and precision. A Hotelling T2 was run to determine the effect of the classification method on the combined variables. RESULTS The EARS platform has been developed, validated and applied to monitor the conversation about COVID-19 since December 2020. A total of 215,469,045 social posts were collected for processing from December 2020 to February 2022. The machine learning algorithm outperformed the boolean search filters method by a large margin for precision and recall in both English and Spanish (p<.001). Demographic and other filters provided useful insights on data, and gender split of users in the platform was largely consistent with population level data on social media use. CONCLUSIONS The EARS platform has been specifically developed to address the changing needs of public health analysts during the COVID-19 pandemic. The application of a public health taxonomy and AI technology to a user-friendly social listening platform, accessible directly by analysts, is a significant step in better enabling understanding of global narratives. The platform was designed in a way to allow for scalability, and iterations and new countries and languages have been added. This research has shown that a machine learning approach is more accurate than using only keywords and has benefit for categorizing and understanding large amounts of digital social data during an infodemic. Further technical developments are needed and planned to provide continuous improvements and meet the challenges in generation of infodemic insights from social media faced by infodemic managers and public health professionals.
更多查看译文
AI 理解论文
溯源树
样例
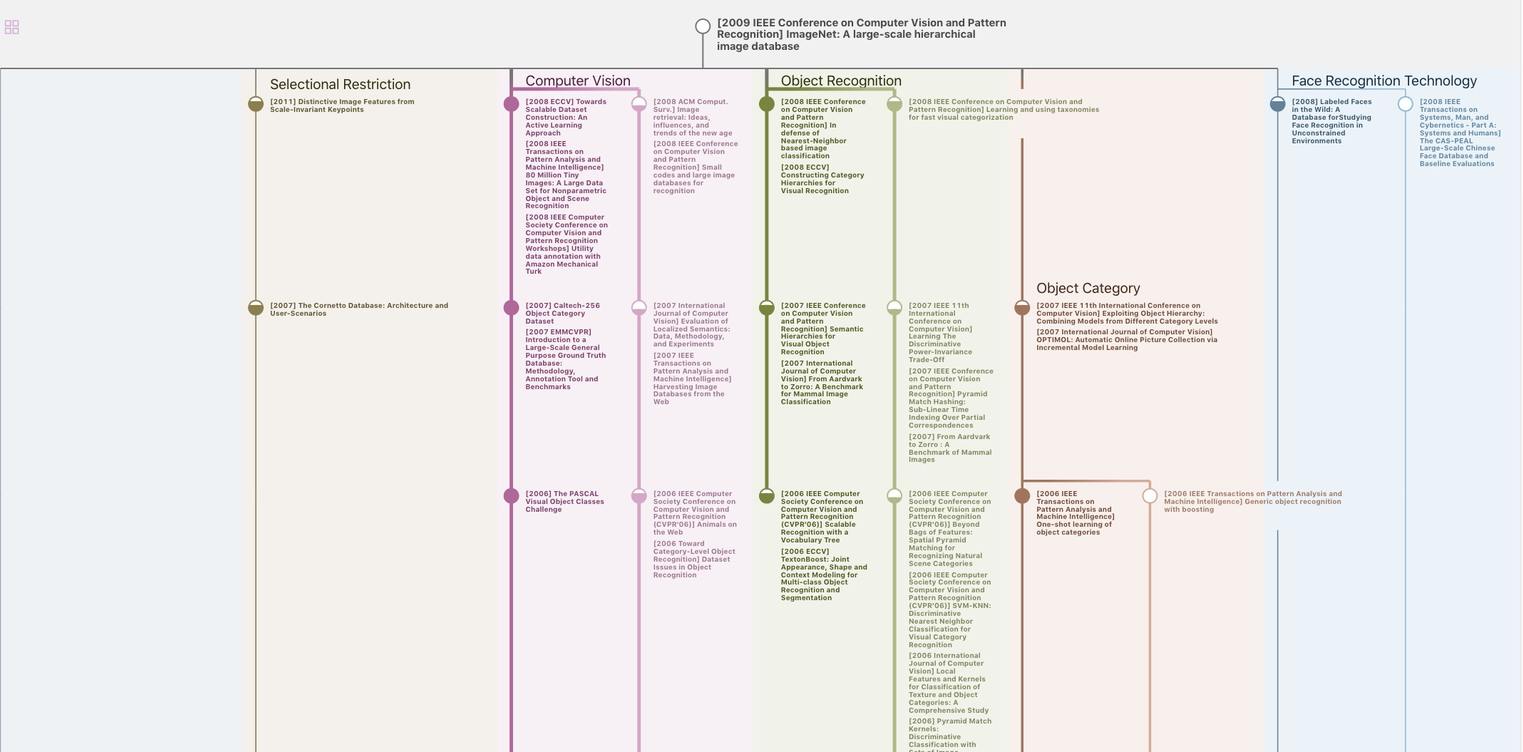
生成溯源树,研究论文发展脉络
Chat Paper
正在生成论文摘要