Matcher: Segment Anything with One Shot Using All-Purpose Feature Matching
ICLR 2024(2023)
摘要
Powered by large-scale pre-training, vision foundation models exhibit
significant potential in open-world image understanding. However, unlike large
language models that excel at directly tackling various language tasks, vision
foundation models require a task-specific model structure followed by
fine-tuning on specific tasks. In this work, we present Matcher, a novel
perception paradigm that utilizes off-the-shelf vision foundation models to
address various perception tasks. Matcher can segment anything by using an
in-context example without training. Additionally, we design three effective
components within the Matcher framework to collaborate with these foundation
models and unleash their full potential in diverse perception tasks. Matcher
demonstrates impressive generalization performance across various segmentation
tasks, all without training. For example, it achieves 52.7
with one example, surpassing the state-of-the-art specialist model by 1.6
addition, Matcher achieves 33.0
semantic segmentation, outperforming the state-of-the-art generalist model by
14.4
flexibility of Matcher when applied to images in the wild. Our code can be
found at https://github.com/aim-uofa/Matcher.
更多查看译文
关键词
Vision Foundation Models,Segment Anything,Training-Free Generalist,Matcher
AI 理解论文
溯源树
样例
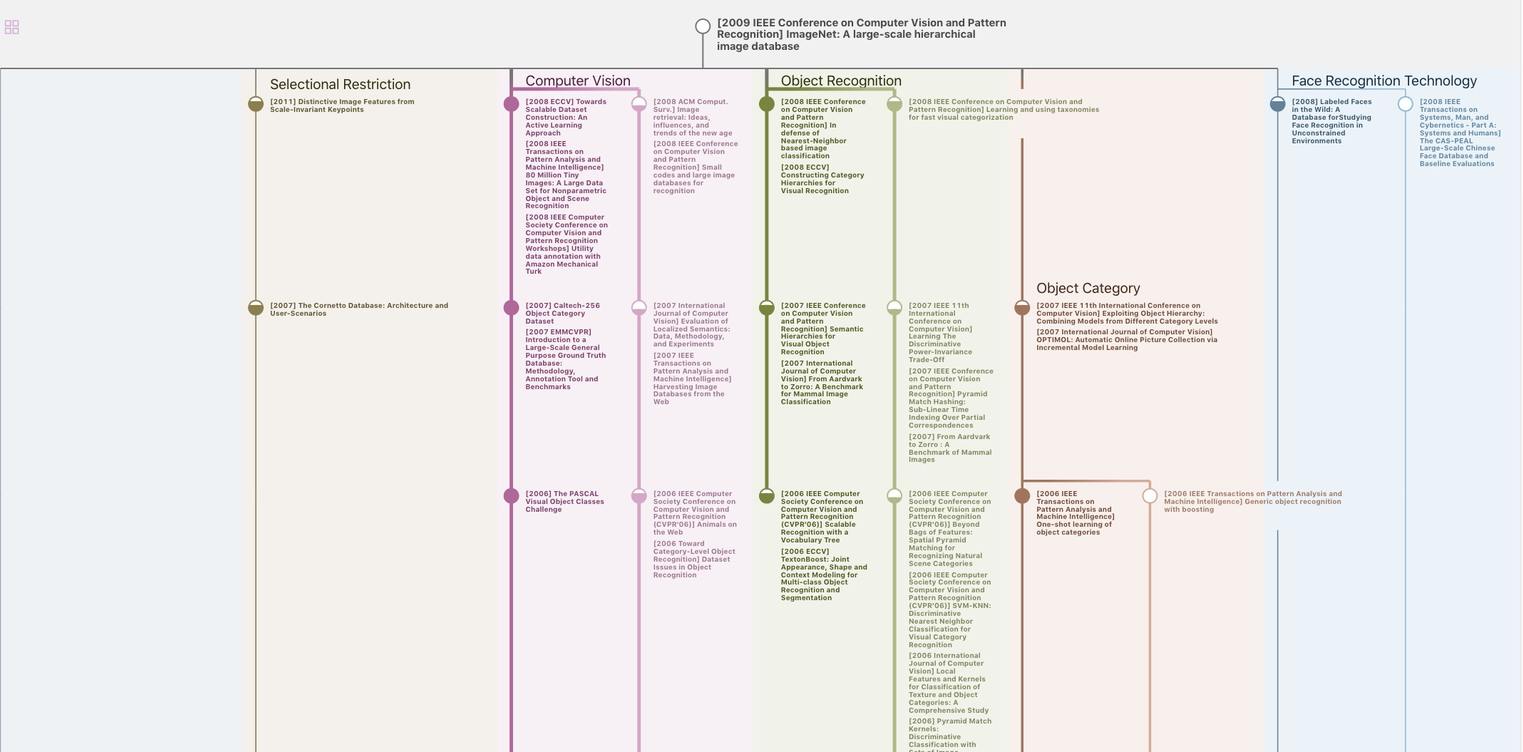
生成溯源树,研究论文发展脉络
Chat Paper
正在生成论文摘要