Bayesian Numerical Integration with Neural Networks
CoRR(2023)
摘要
Bayesian probabilistic numerical methods for numerical integration offer significant advantages over their non-Bayesian counterparts: they can encode prior information about the integrand, and can quantify uncertainty over estimates of an integral. However, the most popular algorithm in this class, Bayesian quadrature, is based on Gaussian process models and is therefore associated with a high computational cost. To improve scalability, we propose an alternative approach based on Bayesian neural networks which we call Bayesian Stein networks. The key ingredients are a neural network architecture based on Stein operators, and an approximation of the Bayesian posterior based on the Laplace approximation. We show that this leads to orders of magnitude speed-ups on the popular Genz functions benchmark, and on challenging problems arising in the Bayesian analysis of dynamical systems, and the prediction of energy production for a large-scale wind farm.
更多查看译文
关键词
bayesian numerical integration,neural networks
AI 理解论文
溯源树
样例
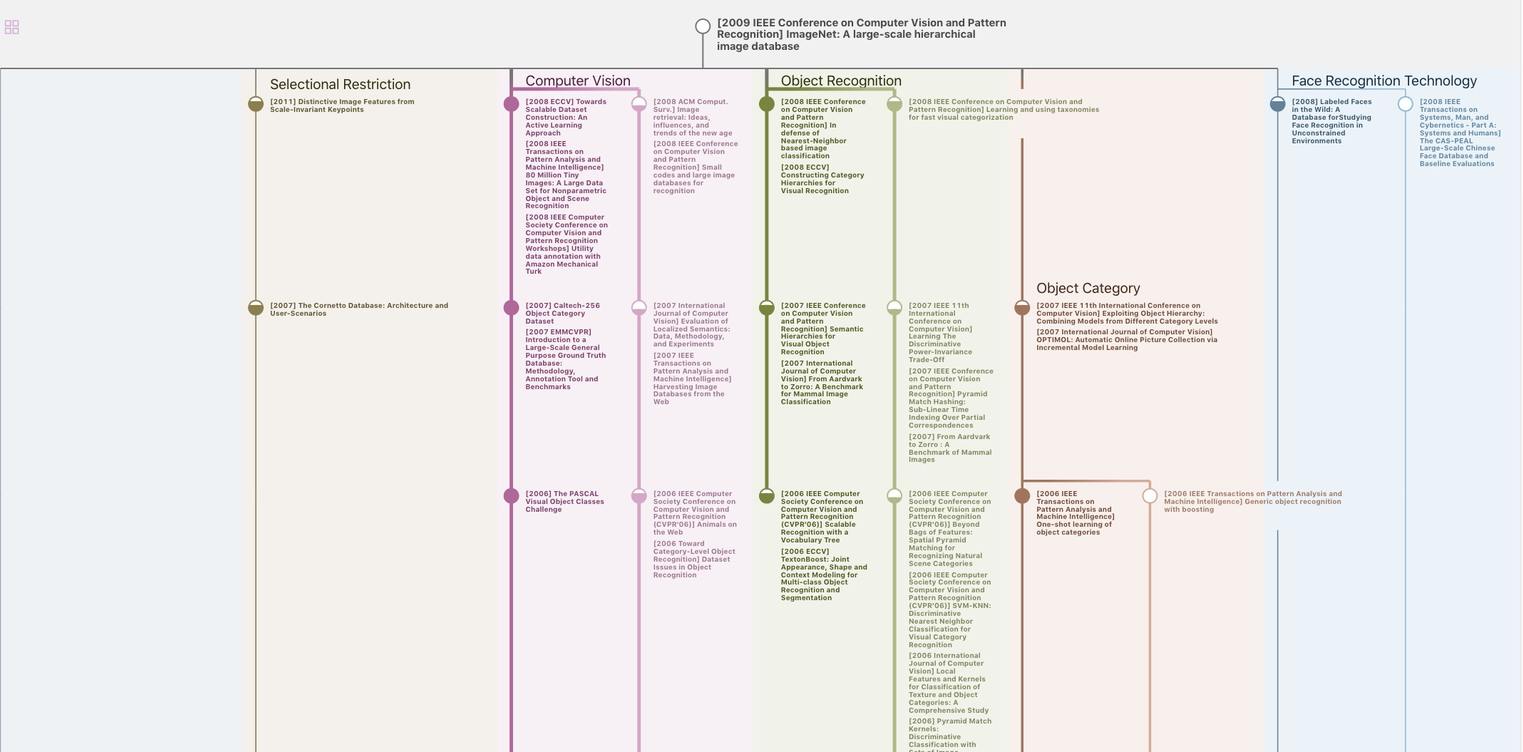
生成溯源树,研究论文发展脉络
Chat Paper
正在生成论文摘要