Faster Differentially Private Convex Optimization via Second-Order Methods
arXiv (Cornell University)(2023)
摘要
Differentially private (stochastic) gradient descent is the workhorse of DP private machine learning in both the convex and non-convex settings. Without privacy constraints, second-order methods, like Newton's method, converge faster than first-order methods like gradient descent. In this work, we investigate the prospect of using the second-order information from the loss function to accelerate DP convex optimization. We first develop a private variant of the regularized cubic Newton method of Nesterov and Polyak, and show that for the class of strongly convex loss functions, our algorithm has quadratic convergence and achieves the optimal excess loss. We then design a practical second-order DP algorithm for the unconstrained logistic regression problem. We theoretically and empirically study the performance of our algorithm. Empirical results show our algorithm consistently achieves the best excess loss compared to other baselines and is 10-40x faster than DP-GD/DP-SGD.
更多查看译文
关键词
private convex optimization,second-order
AI 理解论文
溯源树
样例
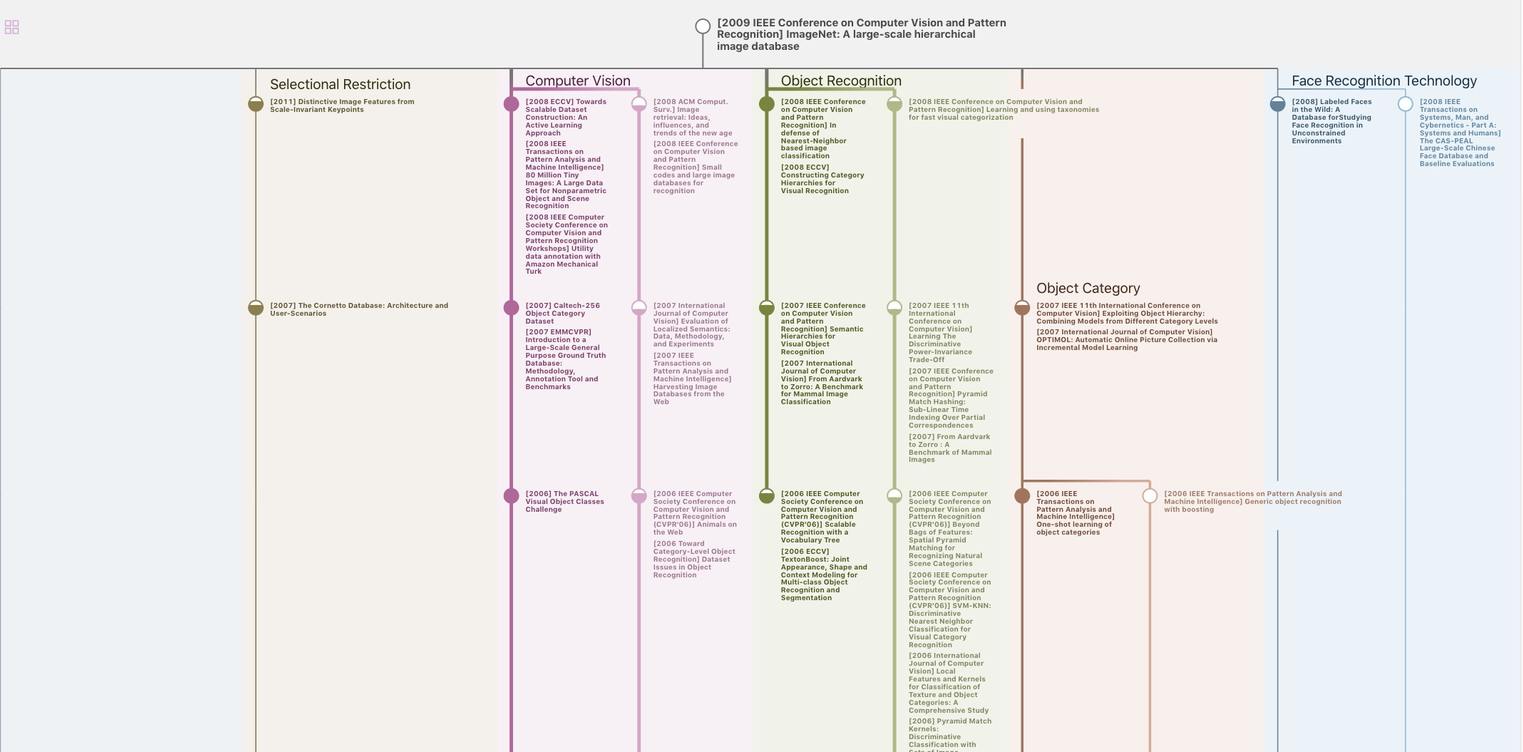
生成溯源树,研究论文发展脉络
Chat Paper
正在生成论文摘要