Retrieval-augmented Multi-label Text Classification
CoRR(2023)
摘要
Multi-label text classification (MLC) is a challenging task in settings of large label sets, where label support follows a Zipfian distribution. In this paper, we address this problem through retrieval augmentation, aiming to improve the sample efficiency of classification models. Our approach closely follows the standard MLC architecture of a Transformer-based encoder paired with a set of classification heads. In our case, however, the input document representation is augmented through cross-attention to similar documents retrieved from the training set and represented in a task-specific manner. We evaluate this approach on four datasets from the legal and biomedical domains, all of which feature highly skewed label distributions. Our experiments show that retrieval augmentation substantially improves model performance on the long tail of infrequent labels especially so for lower-resource training scenarios and more challenging long-document data scenarios.
更多查看译文
关键词
text classification,retrieval-augmented,multi-label
AI 理解论文
溯源树
样例
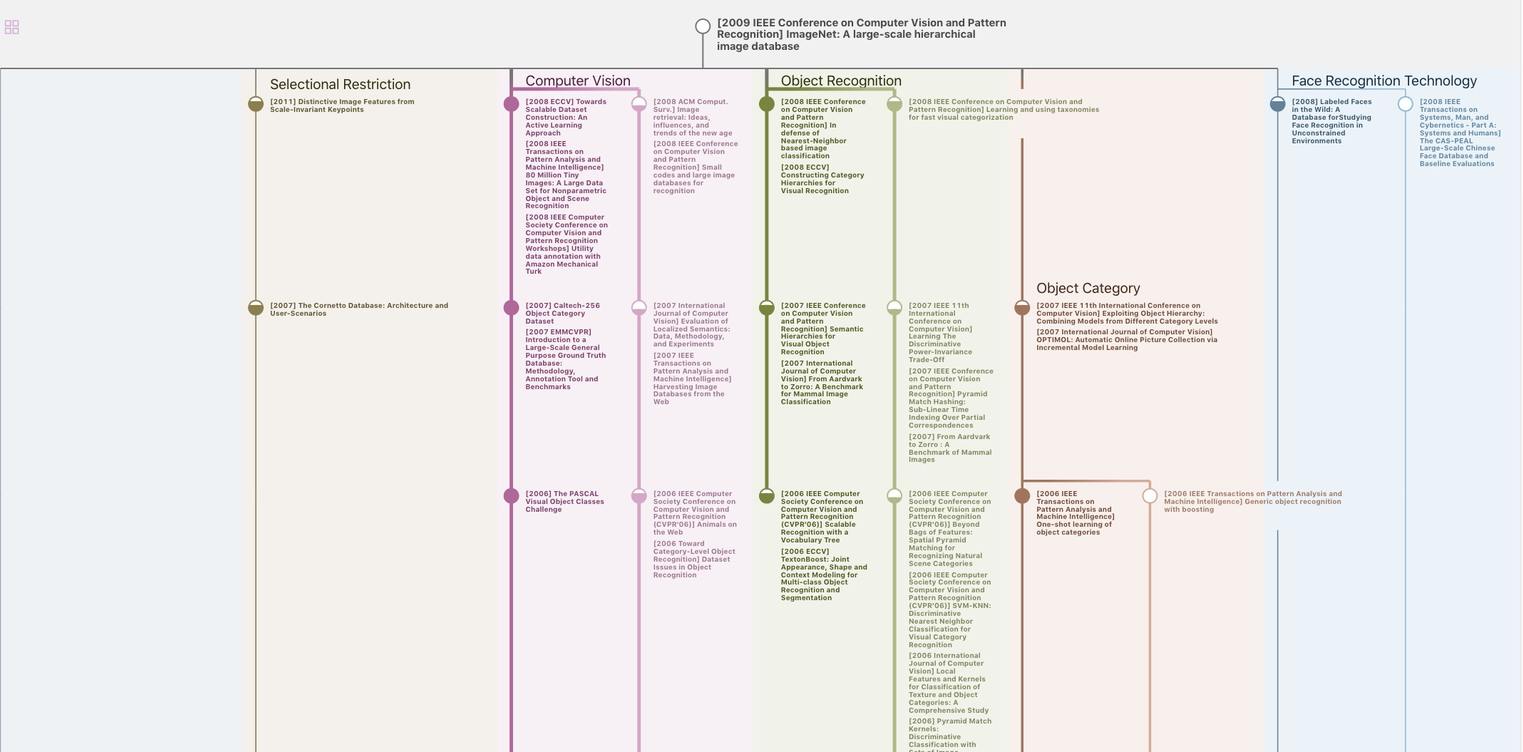
生成溯源树,研究论文发展脉络
Chat Paper
正在生成论文摘要