AD-MERCS: Modeling Normality and Abnormality in Unsupervised Anomaly Detection
CoRR(2023)
摘要
Most anomaly detection systems try to model normal behavior and assume anomalies deviate from it in diverse manners. However, there may be patterns in the anomalies as well. Ideally, an anomaly detection system can exploit patterns in both normal and anomalous behavior. In this paper, we present AD-MERCS, an unsupervised approach to anomaly detection that explicitly aims at doing both. AD-MERCS identifies multiple subspaces of the instance space within which patterns exist, and identifies conditions (possibly in other subspaces) that characterize instances that deviate from these patterns. Experiments show that this modeling of both normality and abnormality makes the anomaly detector performant on a wide range of types of anomalies. Moreover, by identifying patterns and conditions in (low-dimensional) subspaces, the anomaly detector can provide simple explanations of why something is considered an anomaly. These explanations can be both negative (deviation from some pattern) as positive (meeting some condition that is typical for anomalies).
更多查看译文
关键词
unsupervised anomaly
AI 理解论文
溯源树
样例
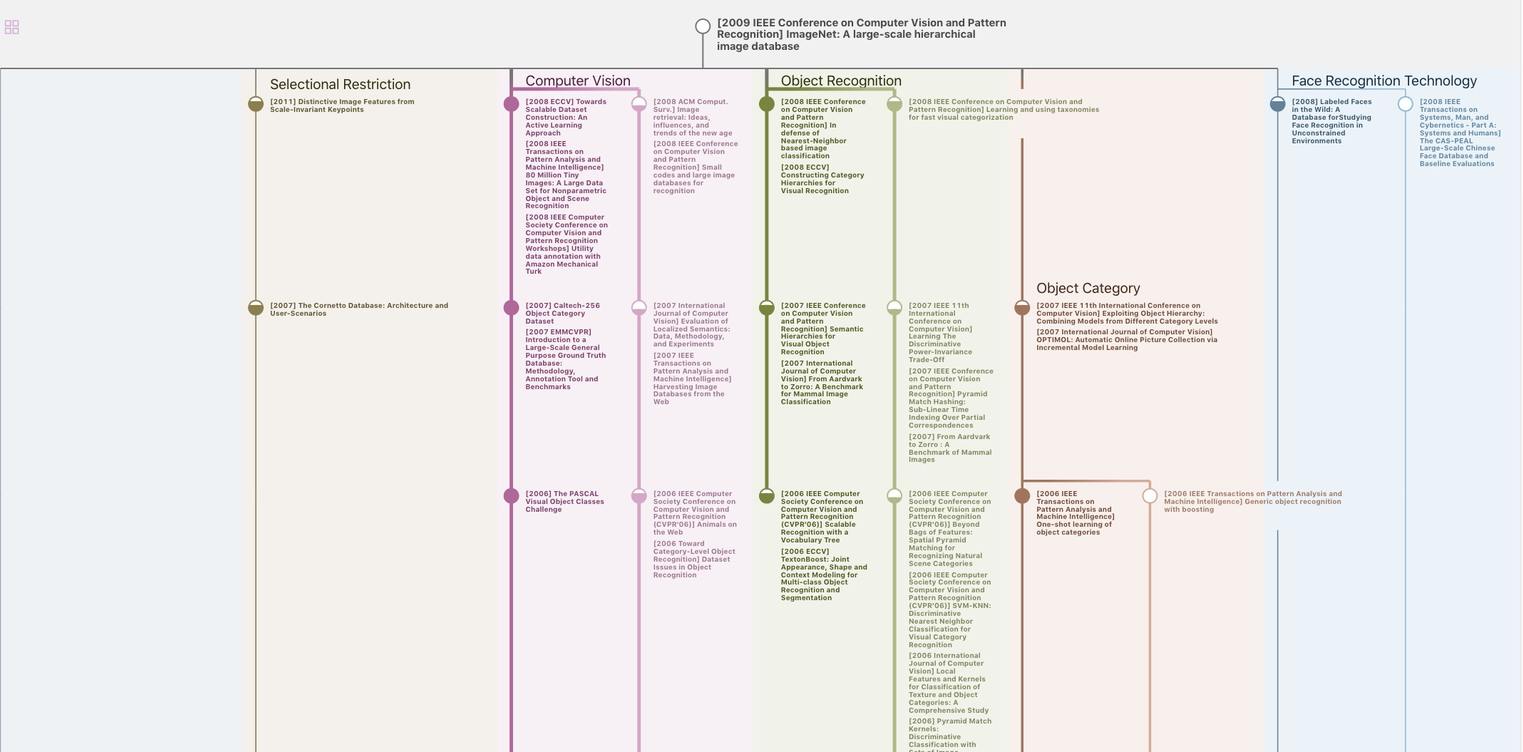
生成溯源树,研究论文发展脉络
Chat Paper
正在生成论文摘要