Unsupervised Visible-Infrared Person ReID by Collaborative Learning with Neighbor-Guided Label Refinement
CoRR(2023)
摘要
Unsupervised learning visible-infrared person re-identification (USL-VI-ReID)
aims at learning modality-invariant features from unlabeled cross-modality
dataset, which is crucial for practical applications in video surveillance
systems. The key to essentially address the USL-VI-ReID task is to solve the
cross-modality data association problem for further heterogeneous joint
learning. To address this issue, we propose a Dual Optimal Transport Label
Assignment (DOTLA) framework to simultaneously assign the generated labels from
one modality to its counterpart modality. The proposed DOTLA mechanism
formulates a mutual reinforcement and efficient solution to cross-modality data
association, which could effectively reduce the side-effects of some
insufficient and noisy label associations. Besides, we further propose a
cross-modality neighbor consistency guided label refinement and regularization
module, to eliminate the negative effects brought by the inaccurate supervised
signals, under the assumption that the prediction or label distribution of each
example should be similar to its nearest neighbors. Extensive experimental
results on the public SYSU-MM01 and RegDB datasets demonstrate the
effectiveness of the proposed method, surpassing existing state-of-the-art
approach by a large margin of 7.76
supervised VI-ReID methods.
更多查看译文
关键词
collaborative learning,label refinement,visible-infrared,neighbor-guided
AI 理解论文
溯源树
样例
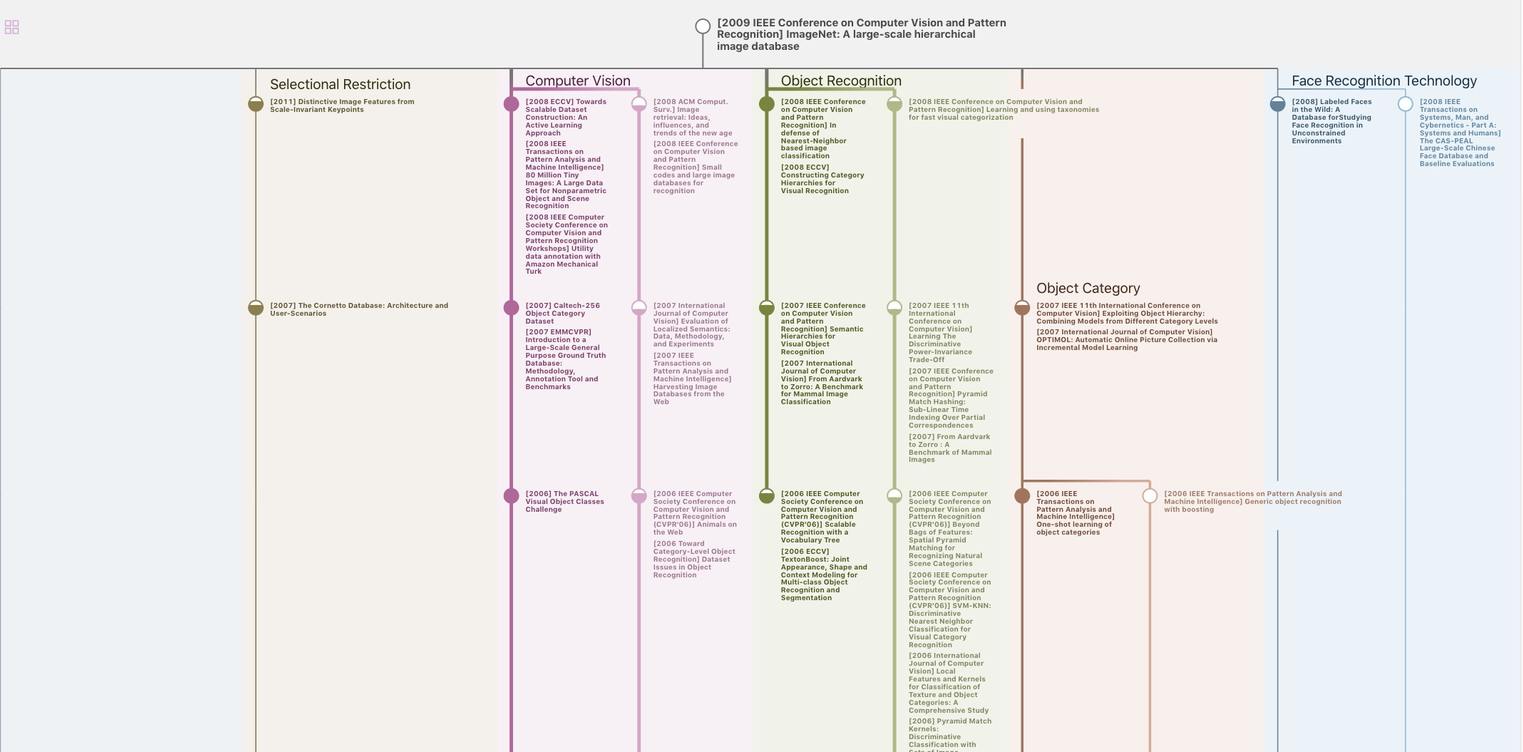
生成溯源树,研究论文发展脉络
Chat Paper
正在生成论文摘要