Learning Interpretable Style Embeddings via Prompting LLMs.
CoRR(2023)
摘要
Style representation learning builds content-independent representations of author style in text. Stylometry, the analysis of style in text, is often performed by expert forensic linguists and no large dataset of stylometric annotations exists for training. Current style representation learning uses neural methods to disentangle style from content to create style vectors, however, these approaches result in uninterpretable representations, complicating their usage in downstream applications like authorship attribution where auditing and explainability is critical. In this work, we use prompting to perform stylometry on a large number of texts to create a synthetic dataset and train human-interpretable style representations we call LISA embeddings. We release our synthetic stylometry dataset and our interpretable style models as resources.
更多查看译文
关键词
interpretable style embeddings,learning
AI 理解论文
溯源树
样例
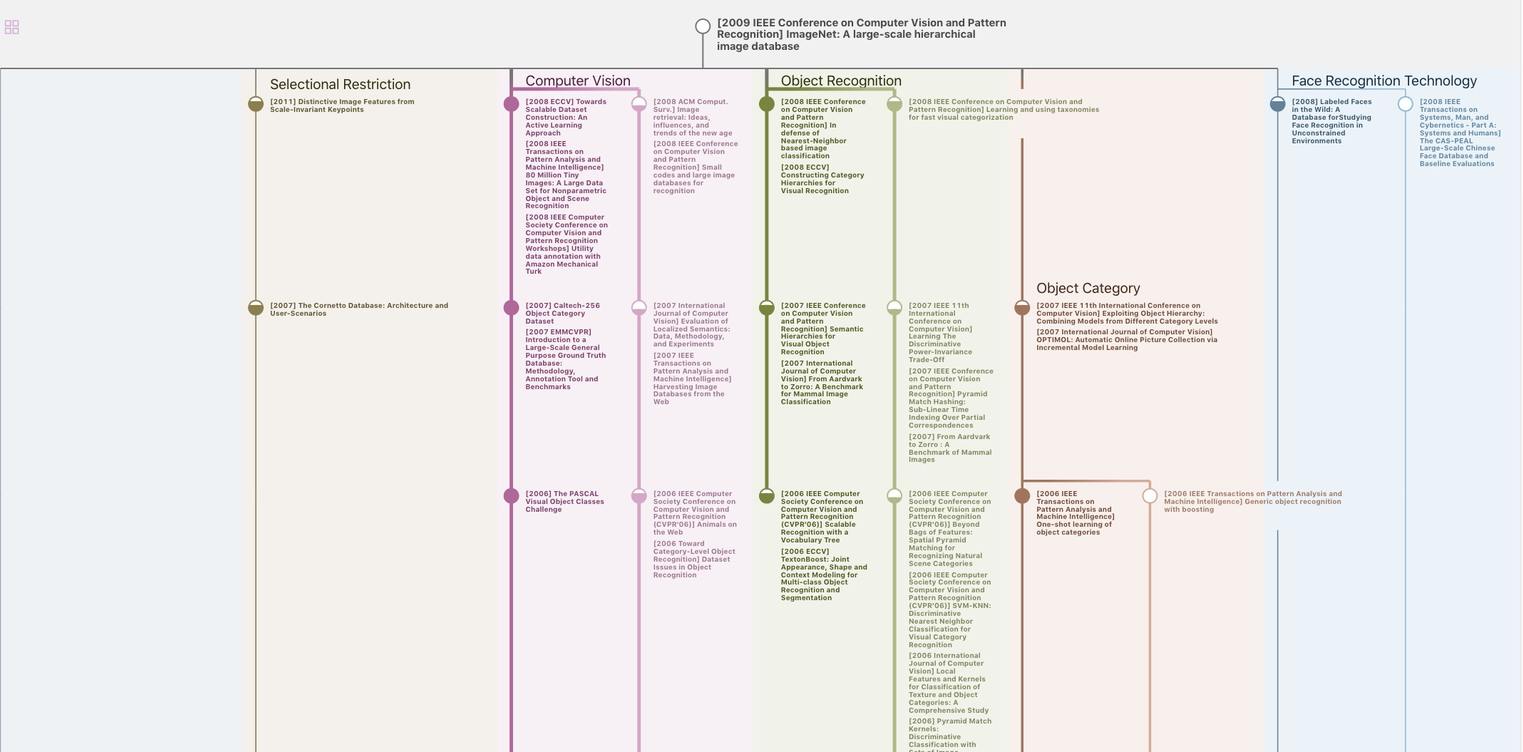
生成溯源树,研究论文发展脉络
Chat Paper
正在生成论文摘要