A Framework for Bidirectional Decoding: Case Study in Morphological Inflection.
CoRR(2023)
摘要
Transformer-based encoder-decoder models that generate outputs in a left-to-right fashion have become standard for sequence-to-sequence tasks. In this paper, we propose a framework for decoding that produces sequences from the "outside-in": at each step, the model chooses to generate a token on the left, on the right, or join the left and right sequences. We argue that this is more principled than prior bidirectional decoders. Our proposal supports a variety of model architectures and includes several training methods, such as a dynamic programming algorithm that marginalizes out the latent ordering variable. Our model improves considerably over a simple baseline based on unidirectional transformers on the SIGMORPHON 2023 inflection task and sets SOTA on the 2022 shared task. The model performs particularly well on long sequences, can learn the split point of words composed of stem and affix (without supervision), and performs better relative to the baseline on datasets that have fewer unique lemmas (but more examples per lemma).
更多查看译文
关键词
bidirectional decoding,morphological
AI 理解论文
溯源树
样例
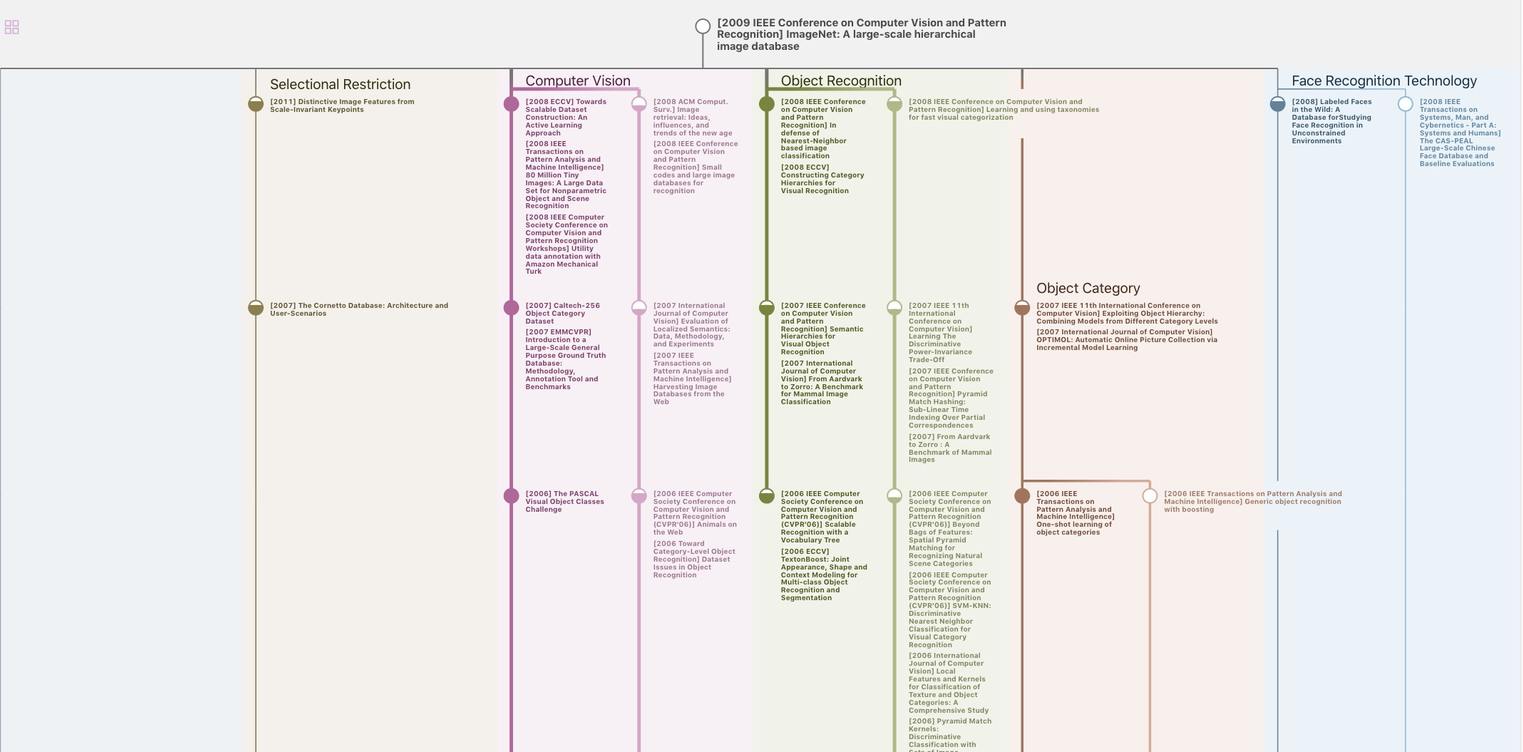
生成溯源树,研究论文发展脉络
Chat Paper
正在生成论文摘要