Self-supervised Predictive Coding Models Encode Speaker and Phonetic Information in Orthogonal Subspaces
CoRR(2023)
摘要
Self-supervised speech representations are known to encode both speaker and phonetic information, but how they are distributed in the high-dimensional space remains largely unexplored. We hypothesize that they are encoded in orthogonal subspaces, a property that lends itself to simple disentanglement. Applying principal component analysis to representations of two predictive coding models, we identify two subspaces that capture speaker and phonetic variances, and confirm that they are nearly orthogonal. Based on this property, we propose a new speaker normalization method which collapses the subspace that encodes speaker information, without requiring transcriptions. Probing experiments show that our method effectively eliminates speaker information and outperforms a previous baseline in phone discrimination tasks. Moreover, the approach generalizes and can be used to remove information of unseen speakers.
更多查看译文
关键词
orthogonal subspaces,phonetic,self-supervised
AI 理解论文
溯源树
样例
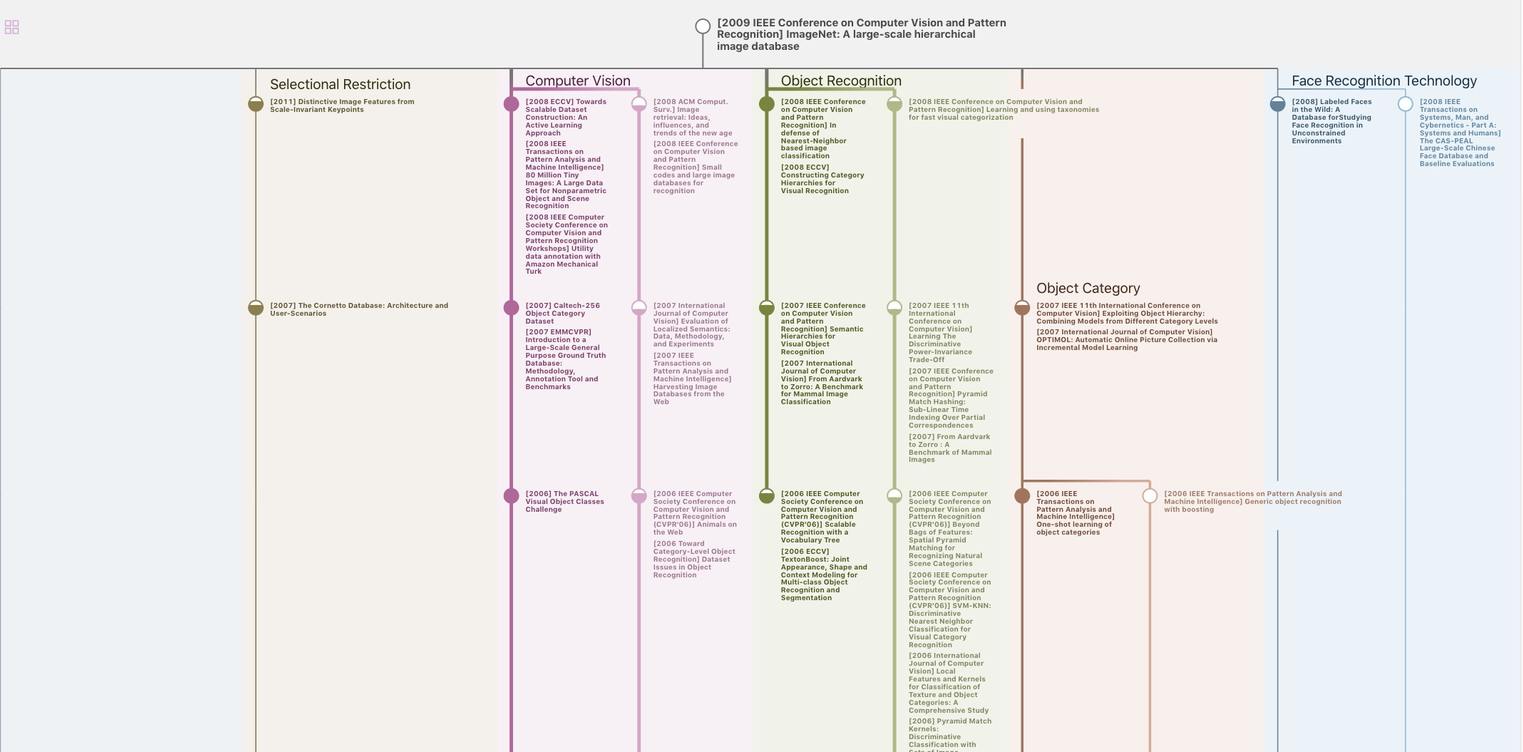
生成溯源树,研究论文发展脉络
Chat Paper
正在生成论文摘要