Many or Few Samples?: Comparing Transfer, Contrastive and Meta-Learning in Encrypted Traffic Classification
CoRR(2023)
摘要
The popularity of Deep Learning (DL), coupled with network traffic visibility reduction due to the increased adoption of HTTPS, QUIC, and DNS-SEC, re-ignited interest towards Traffic Classification (TC). However, to tame the dependency from task-specific large labeled datasets, we need to find better ways to learn representations that are valid across tasks. In this work we investigate this problem comparing transfer learning, meta-learning and contrastive learning against reference Machine Learning (ML) tree-based and monolithic DL models (16 methods total). Using two publicly available datasets, namely MIRAGE19 (40 classes) and AppClassNet (500 classes), we show that (
$i$
) by using DL methods on large datasets we can obtain more general representations with (i i) contrastive learning methods yielding the best performance and (iii) meta-learning the worst one. While (iv) tree-based models can be impractical for large tasks but fit well small tasks, (v) DL methods that reuse better learned representations are closing their performance gap against trees also for small tasks.
更多查看译文
关键词
traffic,classification,transfer,meta-learning
AI 理解论文
溯源树
样例
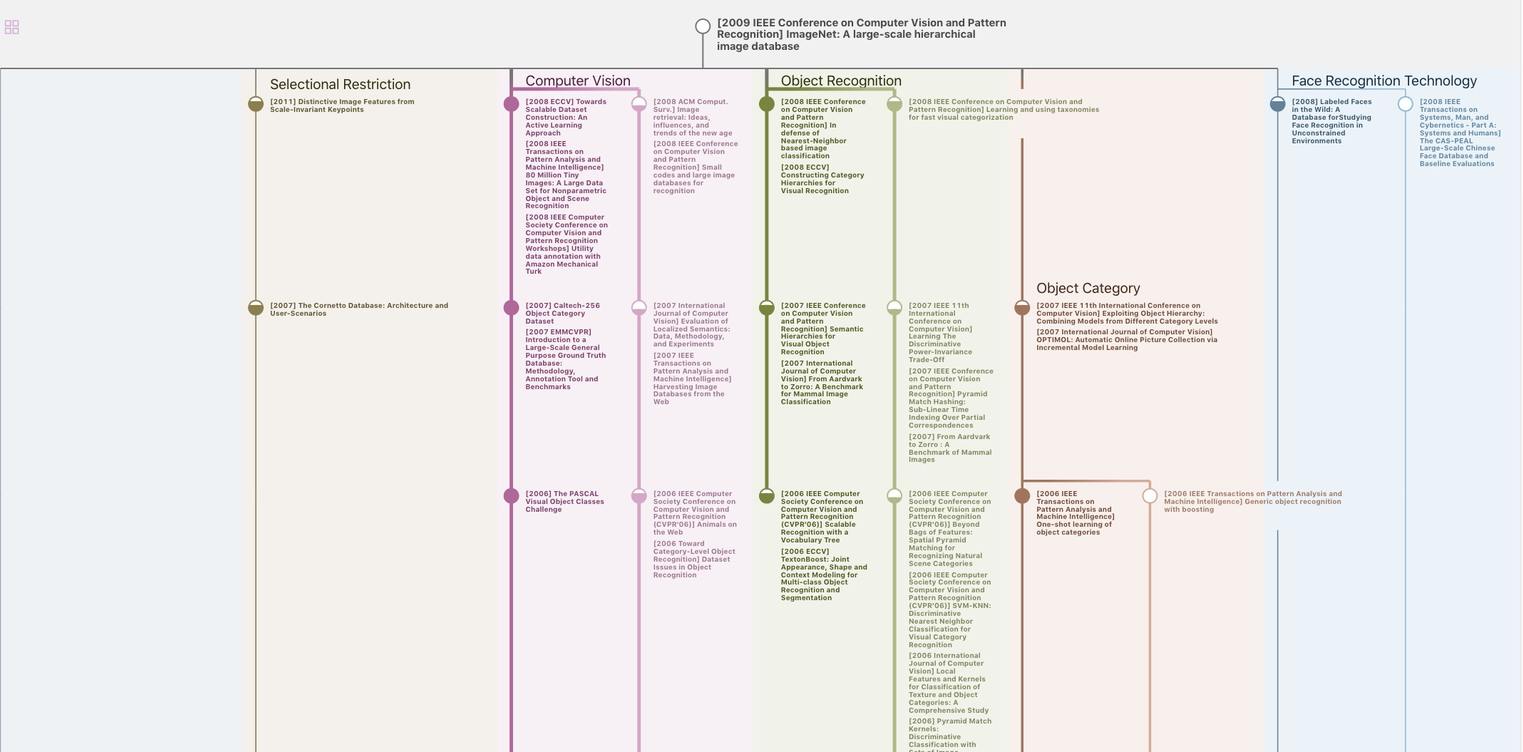
生成溯源树,研究论文发展脉络
Chat Paper
正在生成论文摘要