On First-Order Meta-Reinforcement Learning with Moreau Envelopes
2023 62ND IEEE CONFERENCE ON DECISION AND CONTROL, CDC(2023)
摘要
Meta-Reinforcement Learning ( MRL) is a promising framework for training agents that can quickly adapt to new environments and tasks. In this work, we study the MRL problem under the policy gradient formulation, where we propose a novel algorithm that uses Moreau envelope surrogate regularizers to jointly learn a meta-policy that is adjustable to the environment of each individual task. Our algorithm, called Moreau Envelope Meta-Reinforcement Learning (MEMRL), learns a meta-policy that can adapt to a distribution of tasks by efficiently updating the policy parameters using a combination of gradient-based optimization and Moreau Envelope regularization. Moreau Envelopes provide a smooth approximation of the policy optimization problem, which enables us to apply standard optimization techniques and converge to an appropriate stationary point. We provide a detailed analysis of the MEMRL algorithm, where we show a sublinear convergence rate to a first-order stationary point for non-convex policy gradient optimization. We finally show the effectiveness of MEMRL on a multi-task 2D-navigation problem.
更多查看译文
关键词
learning,first-order,meta-reinforcement
AI 理解论文
溯源树
样例
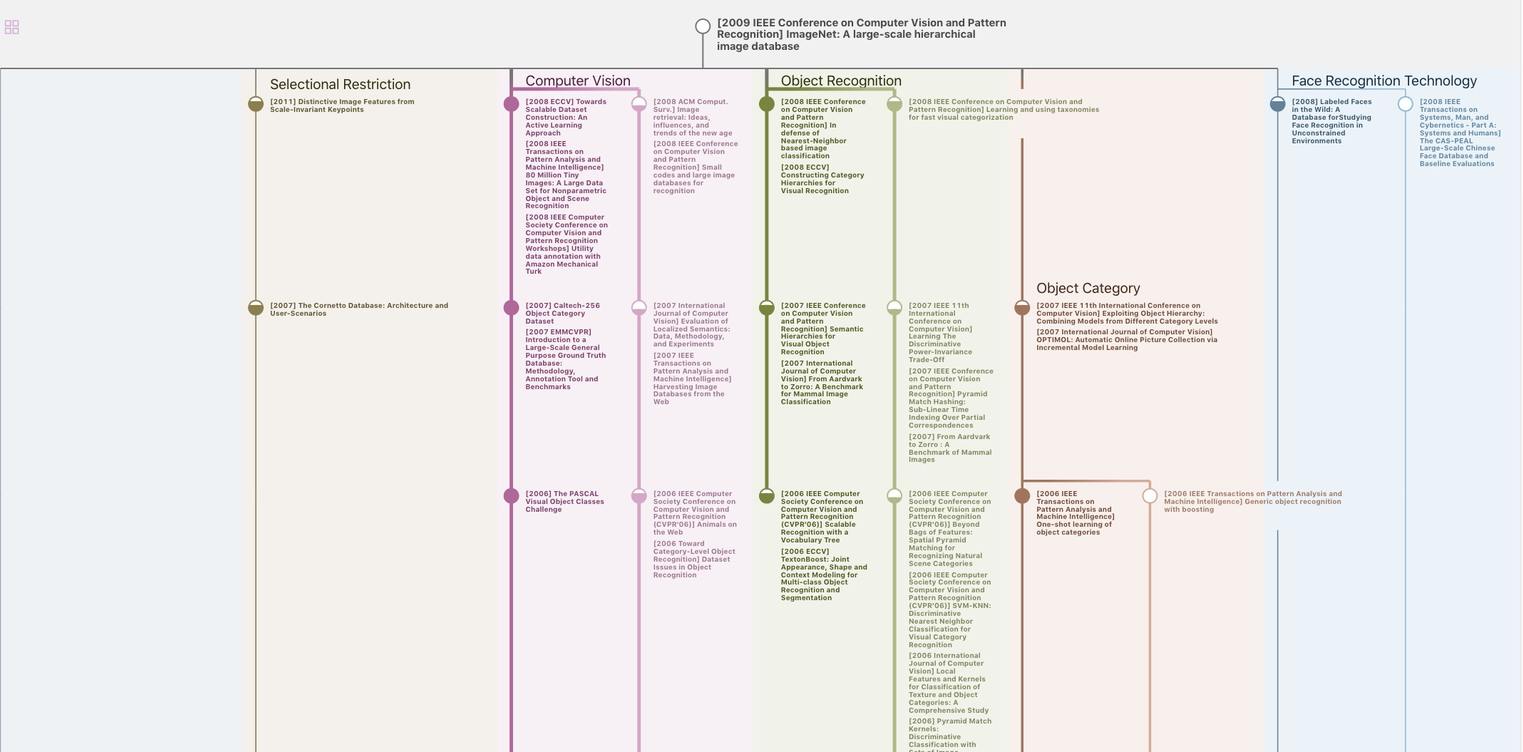
生成溯源树,研究论文发展脉络
Chat Paper
正在生成论文摘要