Prefix Propagation: Parameter-Efficient Tuning for Long Sequences
conf_acl(2023)
摘要
Parameter-efficient tuning aims to mitigate the large memory requirements of adapting pretrained language models for downstream tasks. For example, one popular method, prefix-tuning, prepends trainable tokens to sequences while freezing the rest of the model's parameters. Although such models attain comparable performance with fine-tuning when applied to sequences with short to moderate lengths, we show their inferior performance when modelling long sequences. To bridge this gap, we propose prefix-propagation, a simple but effective approach that conditions prefixes on previous hidden states. We empirically demonstrate that prefix-propagation outperforms prefix-tuning across long-document tasks, while using 50% fewer parameters. To further investigate the proposed architecture, we also show its advantage in calibration, and perform additional study on its relationship with kernel attention. To the best of our knowledge, this work is the first to focus on parameter-efficient learning for long-sequence language tasks.
更多查看译文
关键词
long sequences,propagation,parameter-efficient
AI 理解论文
溯源树
样例
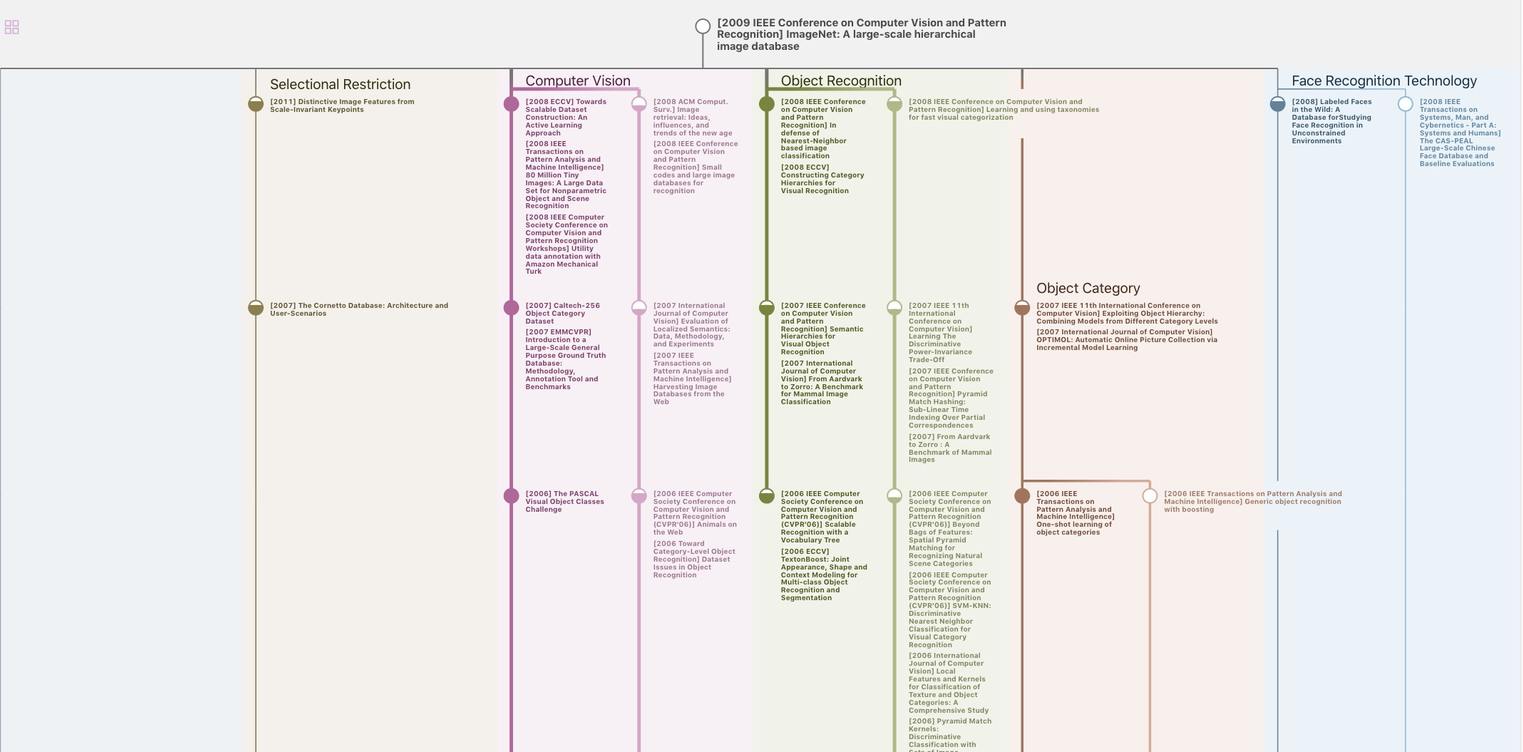
生成溯源树,研究论文发展脉络
Chat Paper
正在生成论文摘要