AnyPredict: Foundation Model for Tabular Prediction
CoRR(2023)
摘要
Foundation models are pre-trained on massive data to perform well across many downstream tasks. They have demonstrated significant success in natural language processing and computer vision. Nonetheless, the use of such models in tabular prediction tasks has been limited, with the main hurdles consisting of (1) the lack of large-scale and diverse tabular datasets with standardized labels and (2) the schema mismatch and predictive target heterogeneity across domains. This paper proposes a method for building training data at scale for tabular prediction foundation models (AnyPredict) using both in-domain and a wide range of out-domain datasets. The method uses a data engine that leverages large language models (LLMs) to consolidate tabular samples to overcome the barrier across tables with varying schema and align out-domain data with the target task using a ``learn, annotate, and audit'' pipeline. The expanded training data enables the pre-trained AnyPredict to support every tabular dataset in the domain without fine-tuning, resulting in significant improvements over supervised baselines: it reaches an average ranking of 1.57 and 1.00 on 7 patient outcome prediction datasets and 3 trial outcome prediction datasets, respectively. In addition, AnyPredict exhibits impressive zero-shot performances: it outperforms supervised XGBoost models by 8.9% and 17.2% on average in two prediction tasks, respectively.
更多查看译文
AI 理解论文
溯源树
样例
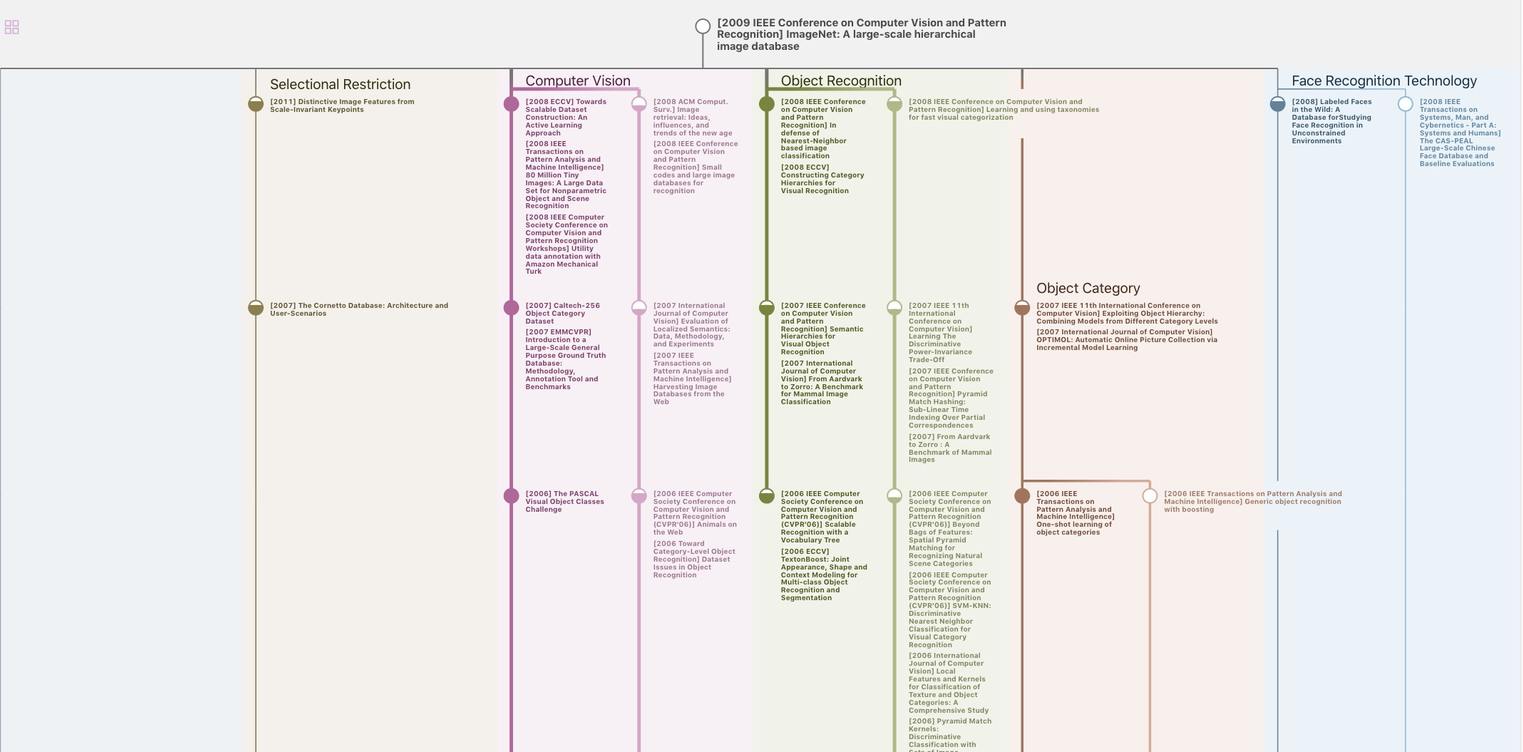
生成溯源树,研究论文发展脉络
Chat Paper
正在生成论文摘要