DADIN: Domain Adversarial Deep Interest Network for cross domain recommender systems
EXPERT SYSTEMS WITH APPLICATIONS(2024)
摘要
The cross-domain recommendation (CDR) model addresses challenges such as data sparsity, the long tail distribution of user-item interactions, and the cold start of items or users. However, solely transferring domain -shared knowledge based on the co-occurrence patterns, without considering user preferences, leads to negative transfer in CDR. To overcome these limitations, we propose an advanced deep learning CDR model called the Domain Adversarial Deep Interest Network (DADIN) aims to facilitate smooth knowledge transfer from the source domain to the target domain and effectively alleviate negative transfer. Firstly, the joint distribution alignment of user preference in DADIN is realized by introducing a skip-connection-based domain agnostic layer, and then the domain classifier is artificially designed to distinguish between the information coming from the source domain or the target domain. Additionally, DADIN combines prediction loss, global domain confusion loss, and intra-class domain confusion losses through the Min-Max game and gradient reverse layer to achieve collaborative optimization. Two real-world experiments show the area under curve (AUC) of DADIN is 0.78 on the Huawei dataset, and it outperforms its competitors by 0.71% on the Amazon dataset, showcasing its state-of-the-art performance. Moreover, our ablation studies further demonstrate that domain adversarial technique increases the AUC by 2.34% on the Huawei dataset and 16.67% on the Amazon dataset, respectively.
更多查看译文
关键词
Cross-domain recommendation,Click-through rate,Cold-start recommender systems,Transfer learning
AI 理解论文
溯源树
样例
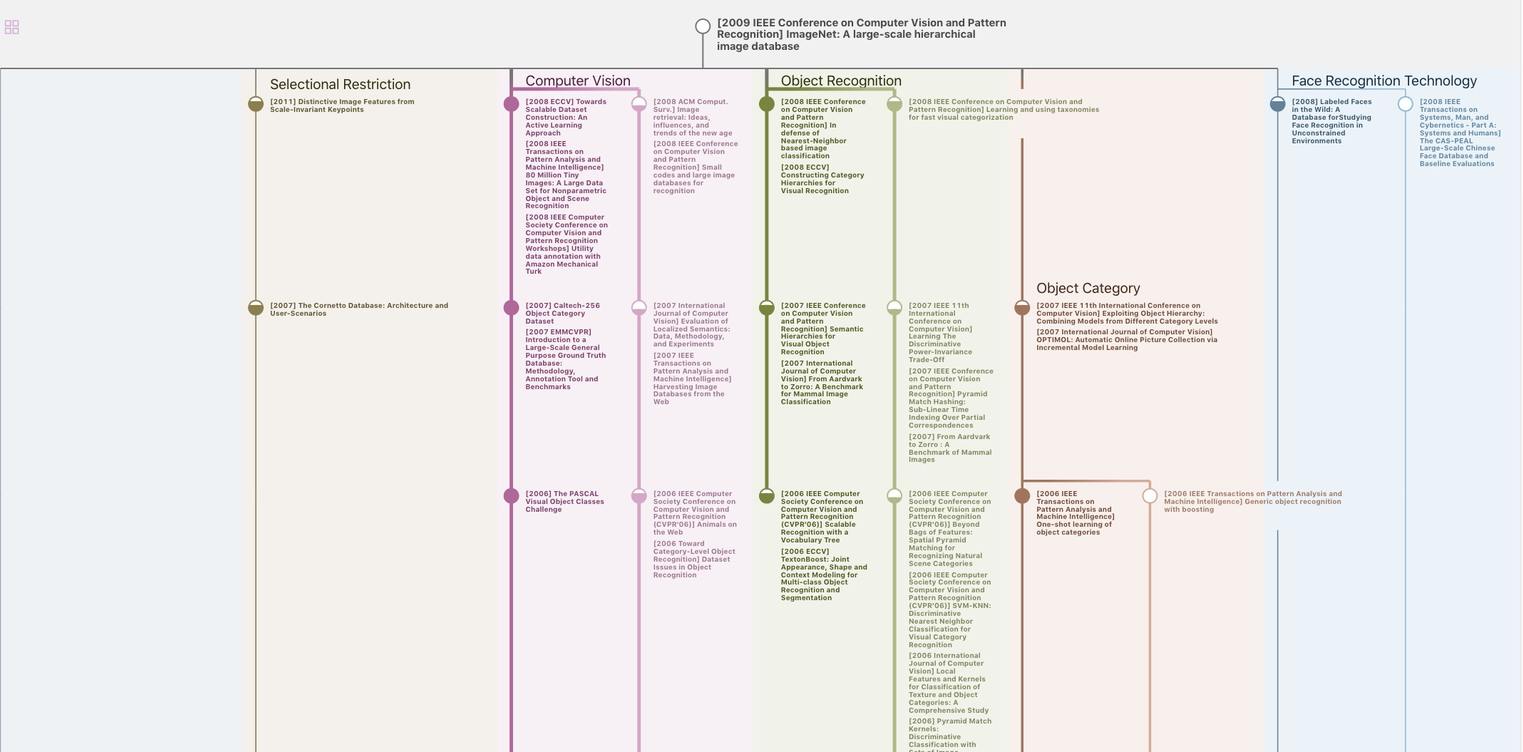
生成溯源树,研究论文发展脉络
Chat Paper
正在生成论文摘要