Let's Sample Step by Step: Adaptive-Consistency for Efficient Reasoning with LLMs
CoRR(2023)
摘要
A popular approach for improving the correctness of output from large language models (LLMs) is Self-Consistency - poll the LLM multiple times and output the most frequent solution. Existing Self-Consistency techniques always draw a constant number of samples per question, where a better approach will be to non-uniformly distribute the available budget based on the amount of agreement in the samples drawn so far. In response, we introduce Adaptive-Consistency, a cost-efficient, model-agnostic technique that dynamically adjusts the number of samples per question using a lightweight stopping criterion. Our experiments over 13 datasets and two LLMs demonstrate that Adaptive-Consistency reduces sample budget by up to 6.0 times with an average accuracy drop of less than 0.1%.
更多查看译文
关键词
efficient reasoning
AI 理解论文
溯源树
样例
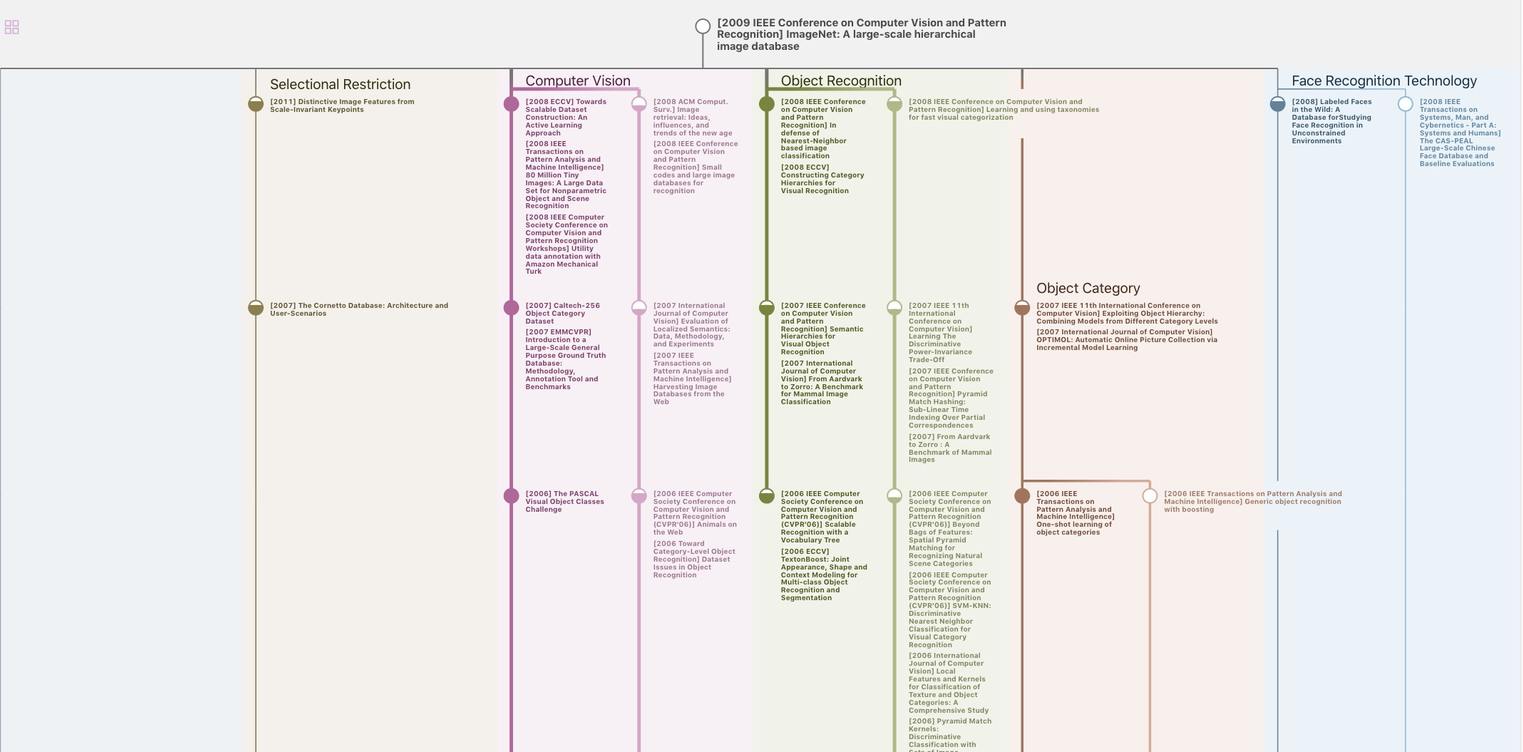
生成溯源树,研究论文发展脉络
Chat Paper
正在生成论文摘要